Methods To Transform Microarray Data For Cancer Prediction
2016 IEEE Conference on Computational Intelligence in Bioinformatics and Computational Biology (CIBCB)(2016)
摘要
Cancer classification based on microarray data has gained attention in recent years from the bioinformatics community, due to a high death toll of cancer and the significance of early diagnosis. Among the many steps in cancer classification, one that is underexplored and can significantly affect the classification performance is data transformation. We develop two transformation techniques, called the unity-based normalization with min-max interval (UBMI) and the standard score with trimmed mean (SSTM), and compare them with existing techniques in terms of accuracy, sensitivity and specificity. The results show that our proposed methods outperform the methods tested. Overall, for example, the SSTM achieves the highest values of accuracy, sensitivity, and specificity in 73 out of 138 cases. The UBMI is the runner up, with 51 winning cases. This advantage confirms the ability of the UBMI and SSTM to accentuate the difference between samples of distinct classes, and highlights the importance of data transformation, a step that otherwise is usually overlooked.
更多查看译文
关键词
feature selection,support vector machine,gene-expression microarray data,data transform
AI 理解论文
溯源树
样例
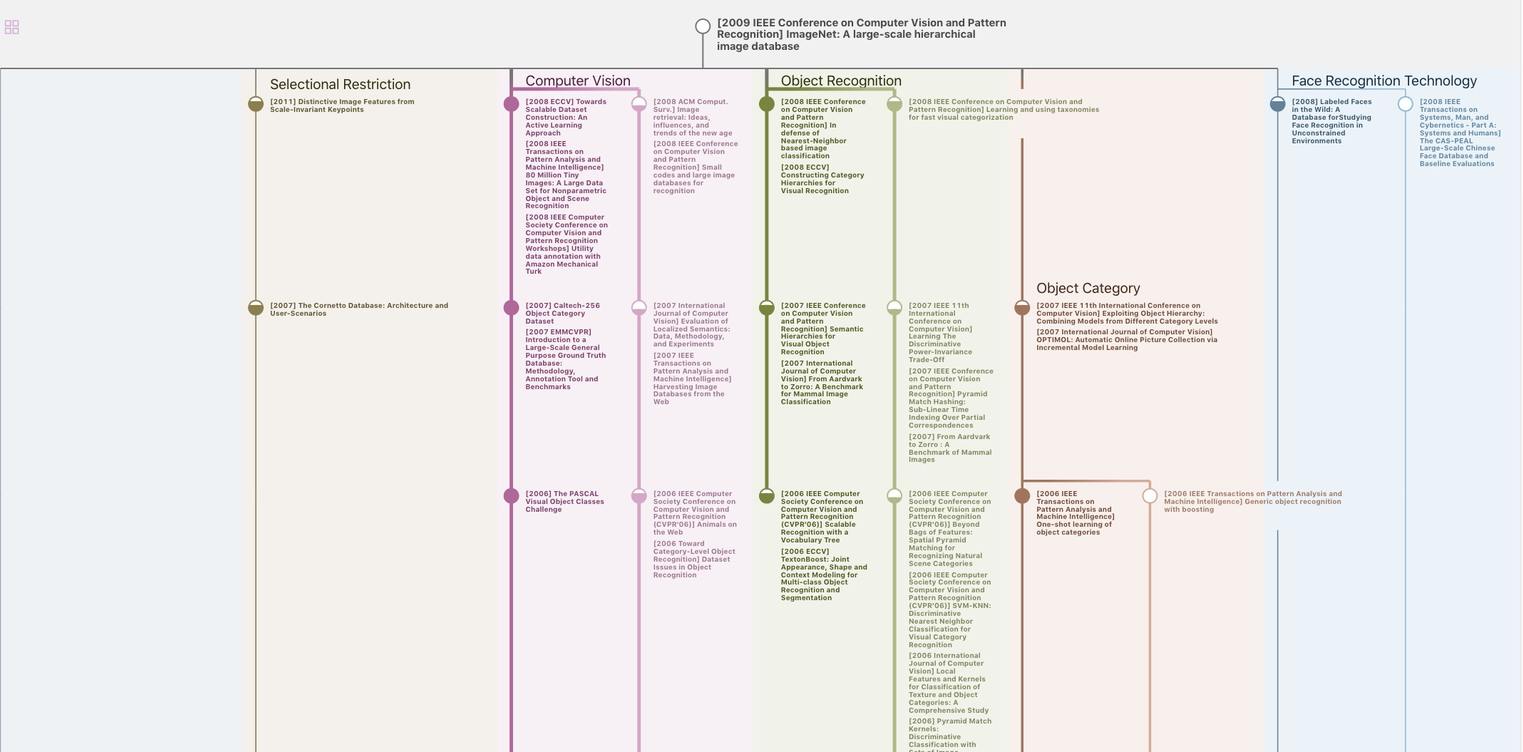
生成溯源树,研究论文发展脉络
Chat Paper
正在生成论文摘要