Model compression applied to small-footprint keyword spotting
17TH ANNUAL CONFERENCE OF THE INTERNATIONAL SPEECH COMMUNICATION ASSOCIATION (INTERSPEECH 2016), VOLS 1-5: UNDERSTANDING SPEECH PROCESSING IN HUMANS AND MACHINES(2016)
摘要
Several consumer speech devices feature voice interfaces that perform on-device keyword spotting to initiate user interactions. Accurate on-device keyword spotting within a tight CPU budget is crucial for such devices. Motivated by this, we investigated two ways to improve deep neural network (DNN) acoustic models for keyword spotting without increasing CPU usage. First, we used low-rank weight matrices throughout the DNN. This allowed us to increase representational power by increasing the number of hidden nodes per layer without changing the total number of multiplications. Second, we used knowledge distilled from an ensemble of much larger DNNs used only during training. We systematically evaluated these two approaches on a massive corpus of far-field utterances. Alone both techniques improve performance and together they combine to give significant reductions in false alarms and misses without increasing CPU or memory usage.
更多查看译文
关键词
keyword spotting, model compression
AI 理解论文
溯源树
样例
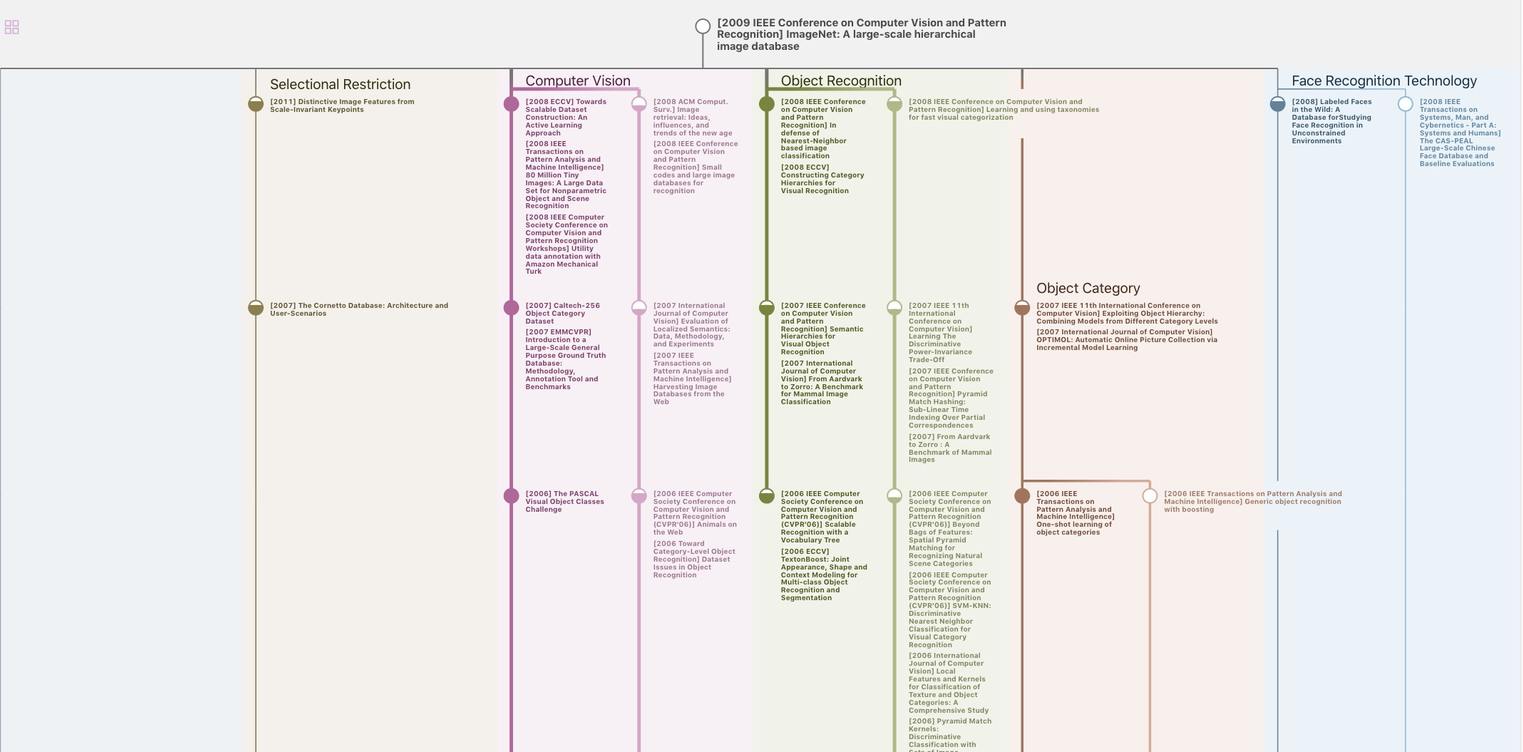
生成溯源树,研究论文发展脉络
Chat Paper
正在生成论文摘要