Subspace Detection of DNN Posterior Probabilities via Sparse Representation for Query by Example Spoken Term Detection
17TH ANNUAL CONFERENCE OF THE INTERNATIONAL SPEECH COMMUNICATION ASSOCIATION (INTERSPEECH 2016), VOLS 1-5: UNDERSTANDING SPEECH PROCESSING IN HUMANS AND MACHINES(2016)
摘要
We cast the query by example spoken term detection (QbE-STD) problem as subspace detection where query and background subspaces are modeled as union of low-dimensional subspaces. The speech exemplars used for subspace modeling are classconditional posterior probabilities estimated using deep neural network (DNN). The query and background training exemplars are exploited to model the underlying low dimensional subspaces through dictionary learning for sparse representation. Given the dictionaries characterizing the query and background subspaces, QbE-STD is performed based on the ratio of the two corresponding sparse representation reconstruction errors. The proposed subspace detection method can be formulated as the generalized likelihood ratio test for composite hypothesis testing. The experimental evaluation demonstrate that the proposed method is able to detect the query given a single example and performs significantly better than a highly competitive QbE-STD baseline system based on dynamic time warping (DTW) for exemplar matching.
更多查看译文
关键词
Deep neural network posterior probabilities, Subspace detection, Dictionary learning, Sparse representation
AI 理解论文
溯源树
样例
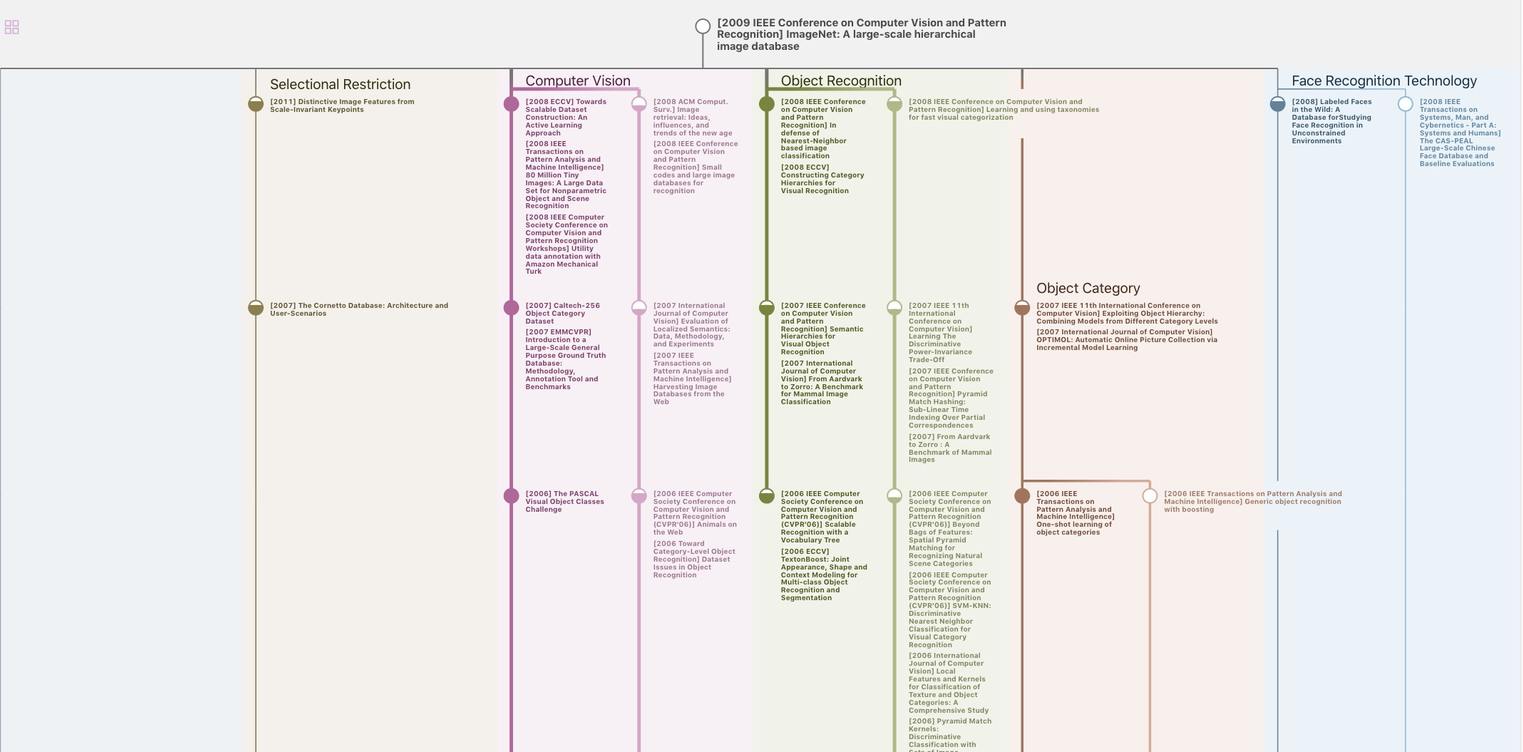
生成溯源树,研究论文发展脉络
Chat Paper
正在生成论文摘要