Multi-task learning and Weighted Cross-entropy for DNN-based Keyword Spotting
17TH ANNUAL CONFERENCE OF THE INTERNATIONAL SPEECH COMMUNICATION ASSOCIATION (INTERSPEECH 2016), VOLS 1-5: UNDERSTANDING SPEECH PROCESSING IN HUMANS AND MACHINES(2016)
摘要
We propose improved Deep Neural Network (DNN) training loss functions for more accurate single keyword spotting on resource-constrained embedded devices. The loss function modifications consist of a combination of multi-task training and weighted cross entropy. In the multi-task architecture, the keyword DNN acoustic model is trained with two tasks in parallel - the main task of predicting the keyword-specific phone states, and an auxiliary task of predicting LVCSR senones. We show that multi-task learning leads to comparable accuracy over a previously proposed transfer learning approach where the keyword DNN training is initialized by an LVCSR DNN of the same input and hidden layer sizes. The combination of LVCSR-initialization and Multi-task training gives improved keyword detection accuracy compared to either technique alone. We also propose modifying the loss function to give a higher weight on input frames corresponding to keyword phone targets, with a motivation to balance the keyword and background training data. We show that weighted cross-entropy results in additional accuracy improvements. Finally, we show that the combination of 3 techniques-LVCSR-initialization, multi-task training and weighted cross-entropy gives the best results, with significantly lower False Alarm Rate than the LVCSR-initialization technique alone, across a wide range of Miss Rates.
更多查看译文
关键词
keyword spotting, DNN, Deep Neural Network, multi-task learning, weighted cross entropy
AI 理解论文
溯源树
样例
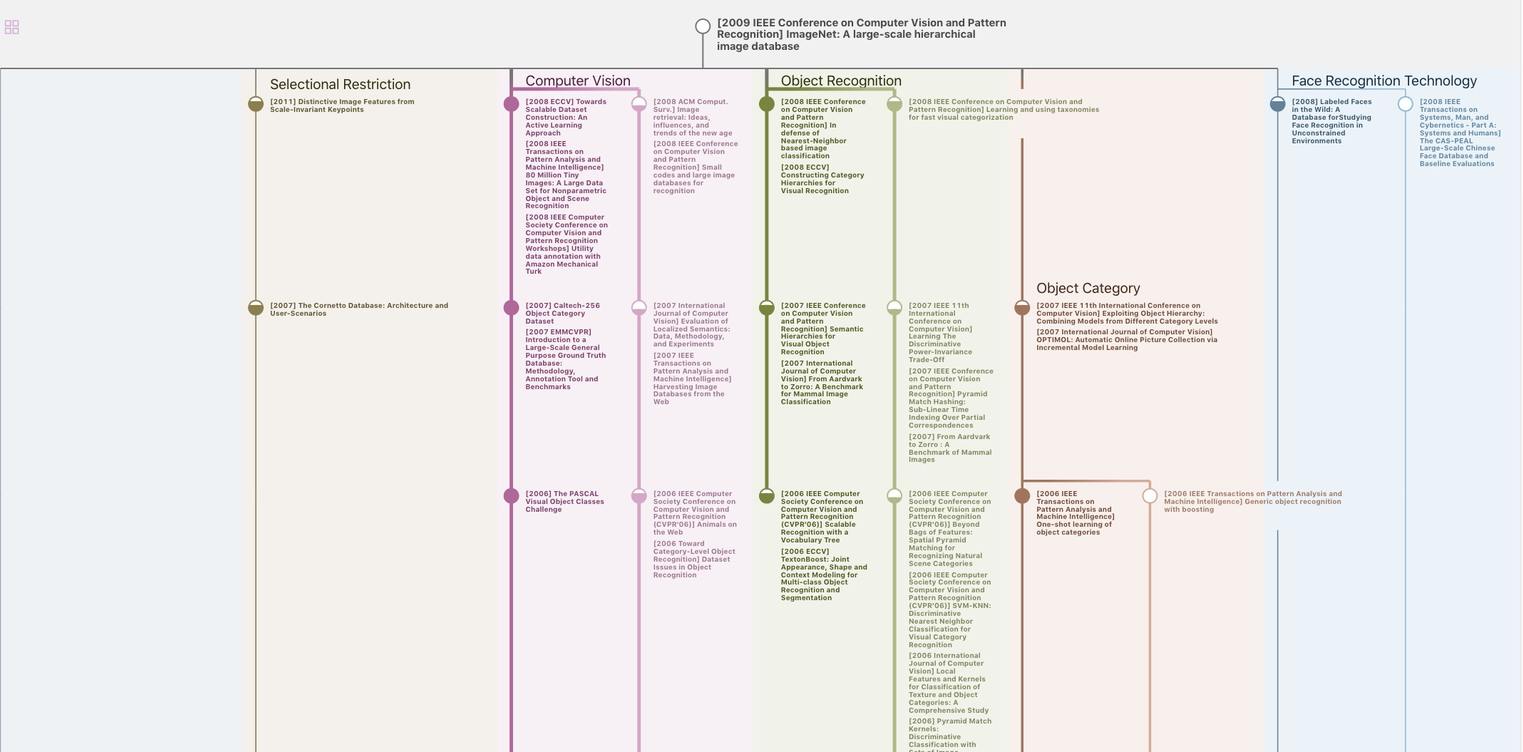
生成溯源树,研究论文发展脉络
Chat Paper
正在生成论文摘要