Pulsar Candidate Selection by Assembling Positive Sample Emphasized Classifiers
2016 12th International Conference on Computational Intelligence and Security (CIS)(2016)
摘要
Pulsar candidate selection identifies prospective observations of modern radio pulsar surveys for further inspection in search of real pulsars. Typically, human experts visually select valuable candidates and eliminate radio frequency interference or other noises. Recently, machine learning methods are adopted to automate this task, which saves human labor and makes it possible for processing millions of observations efficiently. Considering the number of positive training samples are relatively too small and the cost of incorrectly labeling a real pulsar candidate as negative is large, we propose a novel hierarchical candidate-sifting model by emphasizing the cost of incorrect prediction of positive samples and assembling multiple classifiers trained with different weighting parameters. Experiments on three pulsar selection datasets demonstrate our proposed method improves the pulsar-sifting performance a lot according to several standard evaluation metrics.
更多查看译文
关键词
Pulsar candidate selection,positive sample emphasized,assemble classifiers
AI 理解论文
溯源树
样例
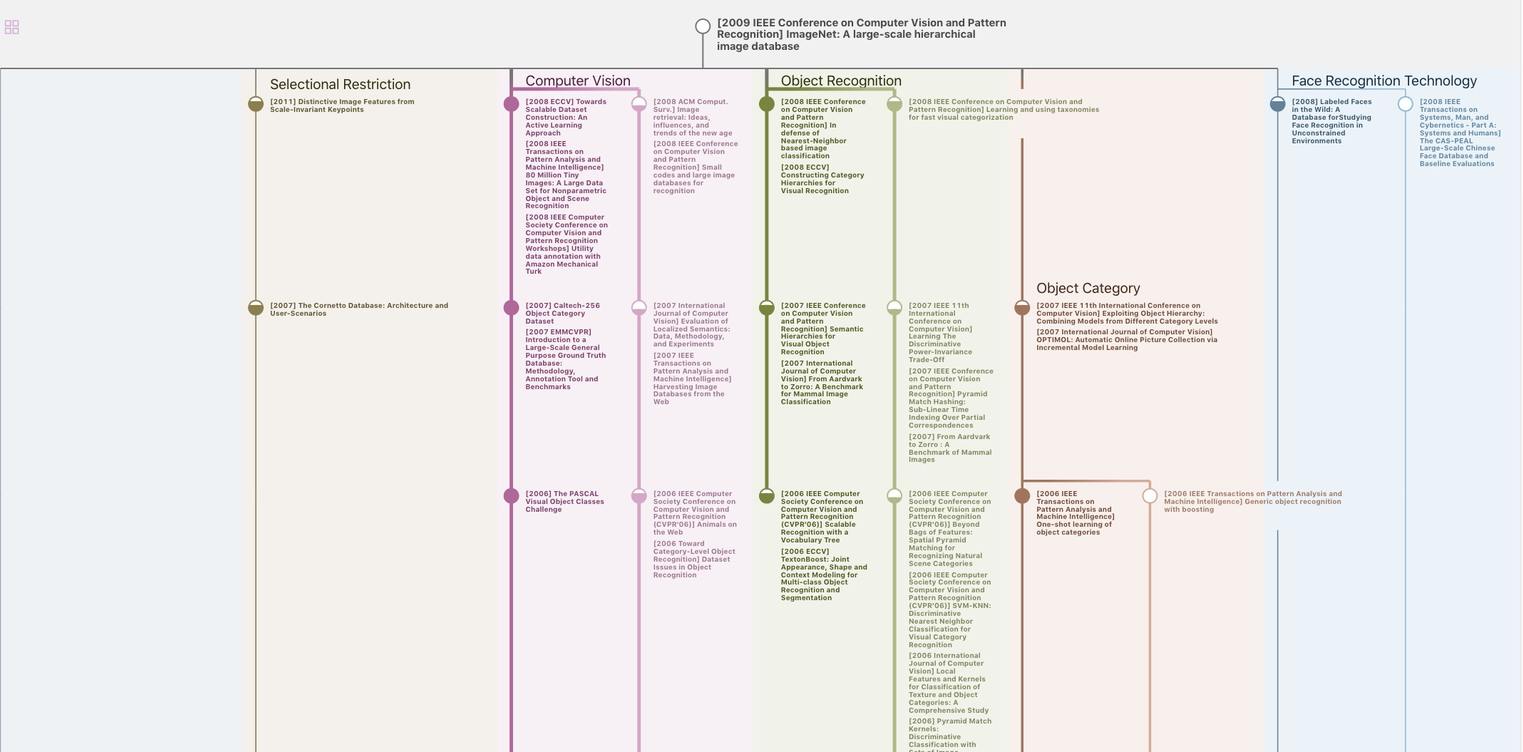
生成溯源树,研究论文发展脉络
Chat Paper
正在生成论文摘要