Distributed Incremental Wlpsvm Learning
2016 IEEE Symposium Series on Computational Intelligence (SSCI)(2016)
摘要
Weighted linear proximal support vector machine (wLPSVM) is known as an efficient binary classification algorithm with good accuracy and class-imbalance robustness. In this work, original batch wLPSVM is facilitated with distributed incremental learning capability, which allows simultaneously learning from multiple streaming data sources that are geographically distributed. In our approach, incremental and distributed learning are solved as a merging problem at the same time. A new wLPSVM expression is derived. In the new expression, knowledge from samples are presented as a set of class-wised core matrices, and merging knowledge from two subsets of data can be simply accomplished by matrix addition. With the new expression, we are able to conduct incremental and distributed learning at the same time via merging knowledge from multiple incremental stages and multiple data sources.
更多查看译文
关键词
multiple incremental stages,class-wised core matrices,wLPSVM expression,multiple streaming data sources,distributed incremental learning capability,class-imbalance robustness,efficient binary classification algorithm,weighted linear proximal support vector machine
AI 理解论文
溯源树
样例
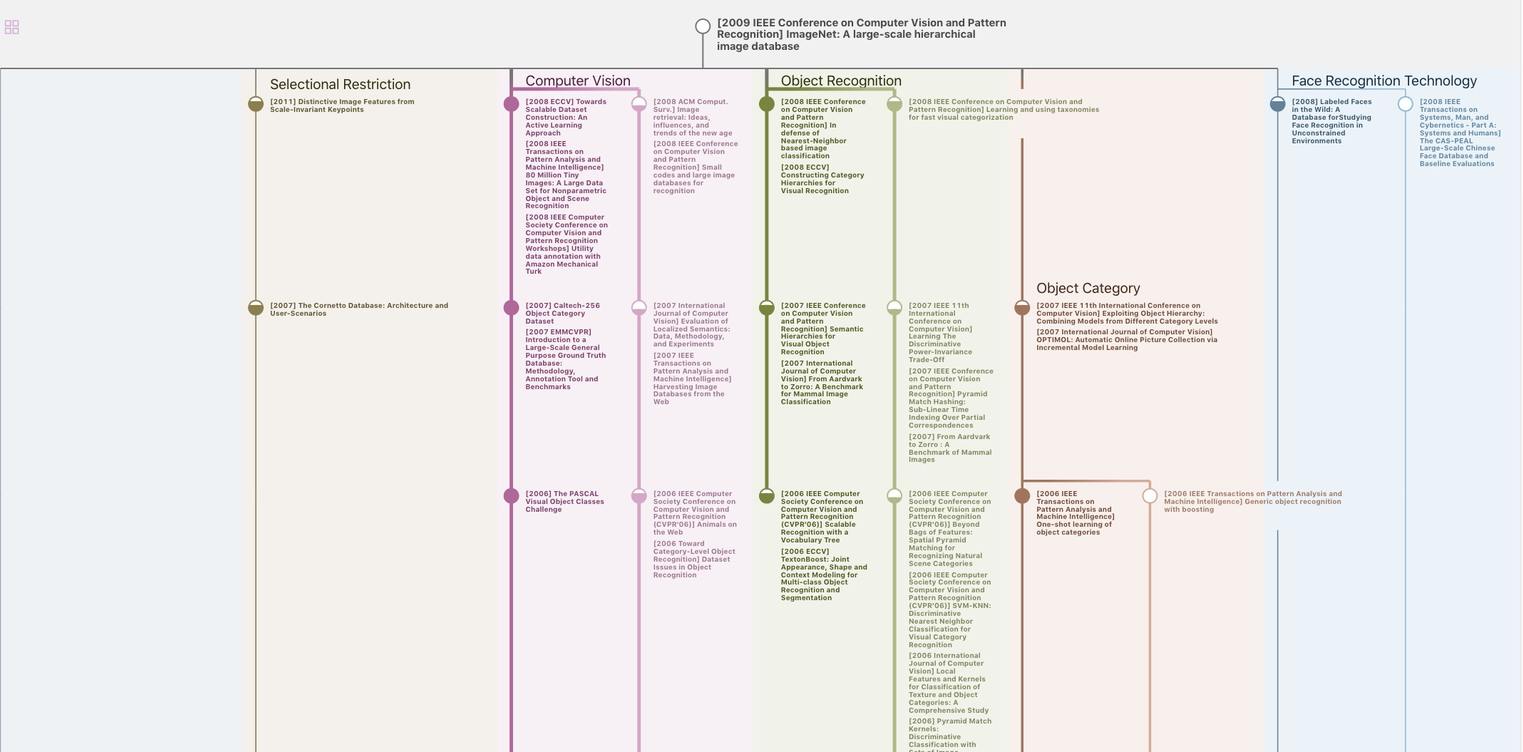
生成溯源树,研究论文发展脉络
Chat Paper
正在生成论文摘要