An Ensemble-Based Recommendation Engine For Scientific Data Transfers
SC16: The International Conference for High Performance Computing, Networking, Storage and Analysis Salt Lake City Utah November, 2016(2016)
摘要
Big data scientists face the challenge of locating valuable datasets across a network of distributed storage locations. We explore methods for recommending storage locations ("endpoints") for users based on a range of prediction models including collaborative filtering and heuristics that consider available information such as user, institution, access history, endpoint ownership, and endpoint usage. We combine the strengths of these models by training a deep recurrent neural network on their predictions. Collectively we show, via analysis of historical usage from the Globus research data management service, that our approach can predict the next storage location accessed by users with 80.3% and 95.3% accuracy for top-1 and top-3 recommendations, respectively. Additionally, our heuristics can predict the endpoints that users will use in the future with over 75% precision and recall.
更多查看译文
关键词
ensemble-based recommendation engine,scientific data transfers,Big Data scientists,distributed storage locations,prediction models,collaborative filtering,deep recurrent neural network training,historical usage analysis,Globus research data management service,storage location
AI 理解论文
溯源树
样例
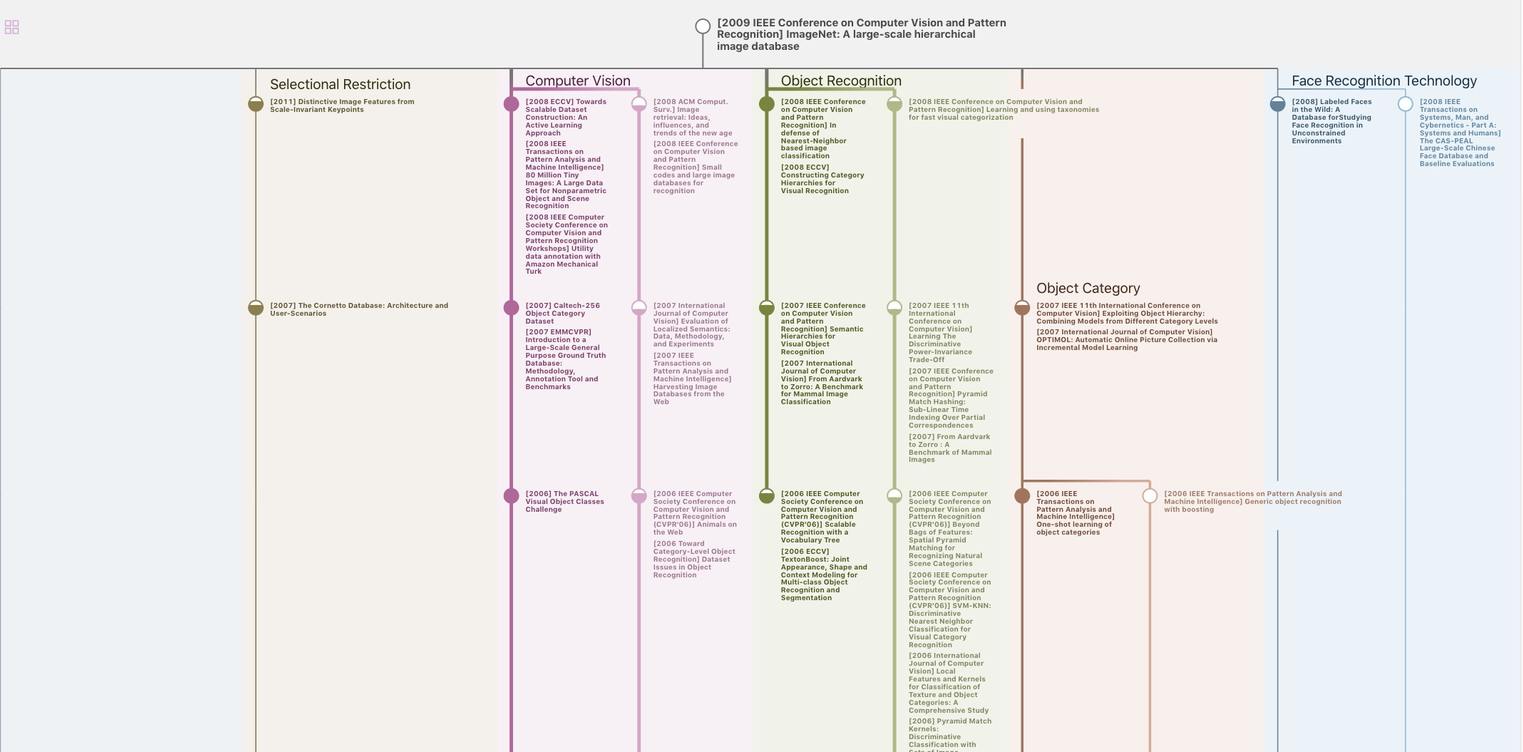
生成溯源树,研究论文发展脉络
Chat Paper
正在生成论文摘要