Human Motion Segmentation Using Cost Weights Recovered From Inverse Optimal Control
2016 IEEE-RAS 16th International Conference on Humanoid Robots (Humanoids)(2016)
摘要
A common hypothesis in human motor control is that human movement is generated by optimizing with respect to a certain criterion and is task dependent. In this paper, a method to segment human movement by detecting changes to the optimization criterion being used via inverse optimal control is proposed. The control strategy employed by the motor system is hypothesized to be a weighted sum of basis cost functions, with the basis weights changing with changes to the motion objective(s). Continuous time series data of movement is processed using a sliding fixed width window, estimating the basis weights of each cost function for each window by minimizing the Karush-Kuhn-Tucker optimality conditions. The quality of the cost function recovery is verified by evaluating the residual. The successfully estimated basis weights are averaged together to create a set of time varying basis weights that describe the changing control strategy of the motion and can be used to segment the movement with simple thresholds. The proposed algorithm is first demonstrated on simulation data and then demonstrated on a dataset of human subjects performing a series of squatting tasks. The proposed approach reliably identifies the squatting movements, achieving a segmentation accuracy of 84%.
更多查看译文
关键词
human motion segmentation,inverse optimal control,human motor control,human movement segmentation,optimization criterion,motor system,basis cost functions,continuous time series data,sliding fixed width window,Karush-Kuhn-Tucker optimality conditions,cost function recovery,time varying basis weights,squatting tasks,squatting movements
AI 理解论文
溯源树
样例
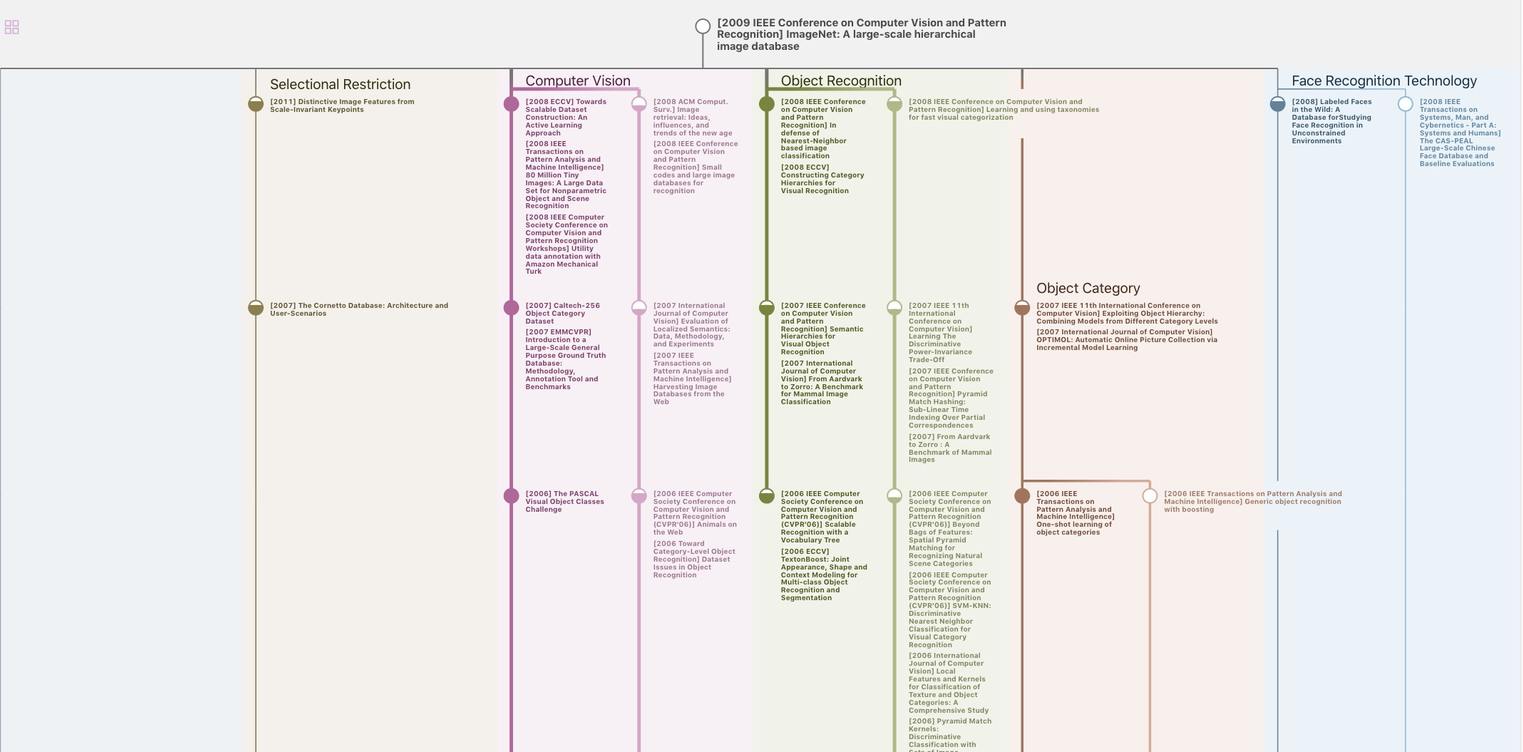
生成溯源树,研究论文发展脉络
Chat Paper
正在生成论文摘要