Robust Kernel Estimation With Outliers Handling For Image Deblurring
2016 IEEE Conference on Computer Vision and Pattern Recognition (CVPR)(2016)
摘要
Estimating blur kernels from real world images is a challenging problem as the linear image formation assumption does not hold when significant outliers, such as saturated pixels and non-Gaussian noise, are present. While some existing non-blind deblurring algorithms can deal with outliers to a certain extent, few blind deblurring methods are developed to well estimate the blur kernels from the blurred images with outliers. In this paper, we present an algorithm to address this problem by exploiting reliable edges and removing outliers in the intermediate latent images, thereby estimating blur kernels robustly. We analyze the effects of outliers on kernel estimation and show that most state-of-the-art blind deblurring methods may recover delta kernels when blurred images contain significant outliers. We propose a robust energy function which describes the properties of outliers for the final latent image restoration. Furthermore, we show that the proposed algorithm can be applied to improve existing methods to deblur images with outliers. Extensive experiments on different kinds of challenging blurry images with significant amount of outliers demonstrate the proposed algorithm performs favorably against the state-of-the-art methods.
更多查看译文
关键词
blurry images,latent image restoration,robust energy function,delta kernels,blurred images,blind deblurring methods,nonblind deblurring algorithms,nonGaussian noise,linear image formation assumption,real world images,blur kernels,image deblurring,outliers handling,robust kernel estimation
AI 理解论文
溯源树
样例
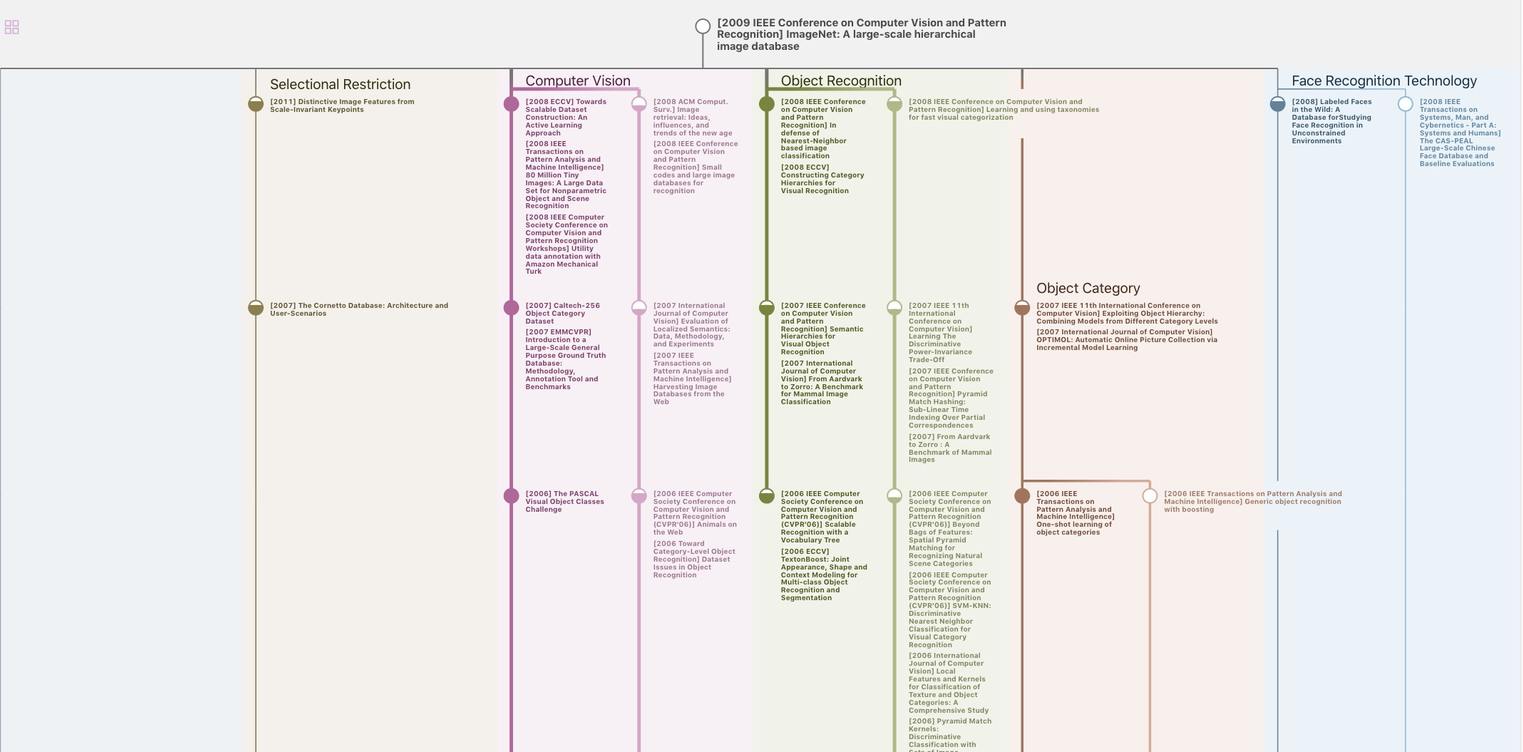
生成溯源树,研究论文发展脉络
Chat Paper
正在生成论文摘要