Grouper: Optimizing Crowdsourced Face Annotations
2016 IEEE Conference on Computer Vision and Pattern Recognition Workshops (CVPRW)(2016)
摘要
This study focuses on the problem of extracting consistent and accurate face bounding box annotations from crowdsourced workers. Aiming to provide benchmark datasets for facial recognition training and testing, we create a 'gold standard' set against which consolidated face bounding box annotations can be evaluated. An evaluation methodology based on scores for several features of bounding box annotations is presented and is shown to predict consolidation performance using information gathered from crowdsourced annotations. Based on this foundation, we present "Grouper," a method leveraging density-based clustering to consolidate annotations by crowd workers. We demonstrate that the proposed consolidation scheme, which should be extensible to any number of region annotation consolidations, improves upon metadata released with the IARPA Janus Benchmark-A. Finally, we compare FR performance using the originally provided IJB-A annotations and Grouper and determine that similarity to the gold standard as measured by our evaluation metric does predict recognition performance.
更多查看译文
关键词
Grouper,crowdsourced face annotation optimization,consistent extraction,face bounding box annotations,benchmark datasets,facial recognition training,facial recognition testing,density-based clustering,metadata,IARPA Janus Benchmark-A,IJB-A annotations,evaluation metric
AI 理解论文
溯源树
样例
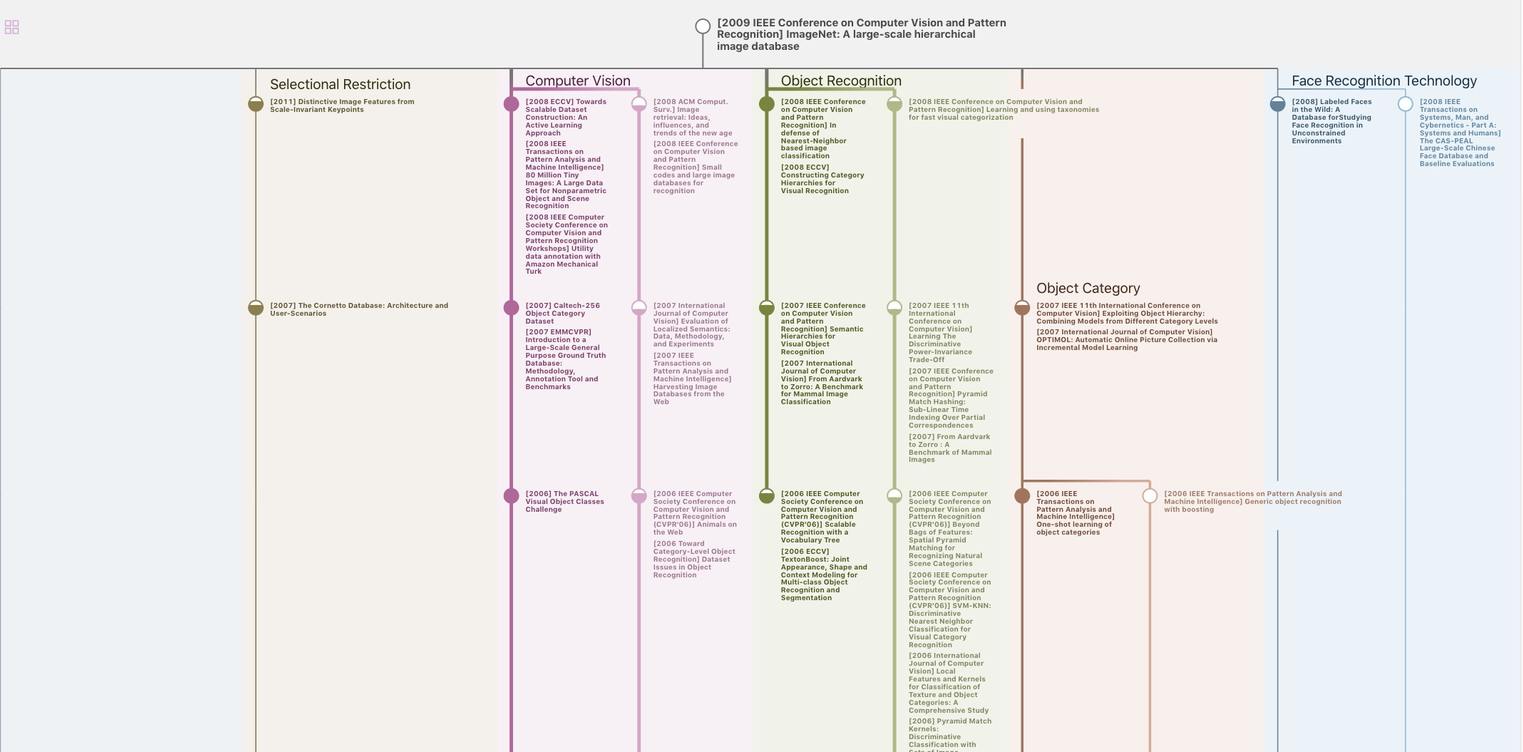
生成溯源树,研究论文发展脉络
Chat Paper
正在生成论文摘要