Exploiting Multimodality in Video Hyperlinking to Improve Target Diversity.
Lecture Notes in Computer Science(2017)
摘要
Video hyperlinking is the process of creating links within a collection of videos to help navigation and information seeking. Starting from a given set of video segments, called anchors, a set of related segments, called targets, must be provided. In past years, a number of content-based approaches have been proposed with good results obtained by searching for target segments that are very similar to the anchor in terms of content and information. Unfortunately, relevance has been obtained to the expense of diversity. In this paper, we study multimodal approaches and their ability to provide a set of diverse yet relevant targets. We compare two recently introduced cross-modal approaches, namely, deep auto-encoders and bimodal LDA, and experimentally show that both provide significantly more diverse targets than a state-of-the-art baseline. Bimodal autoencoders offer the best trade-off between relevance and diversity, with bimodal LDA exhibiting slightly more diverse targets at a lower precision.
更多查看译文
关键词
Latent Dirichlet Allocation, Video Segment, Latent Topic, Deep Neural Network, Visual Concept
AI 理解论文
溯源树
样例
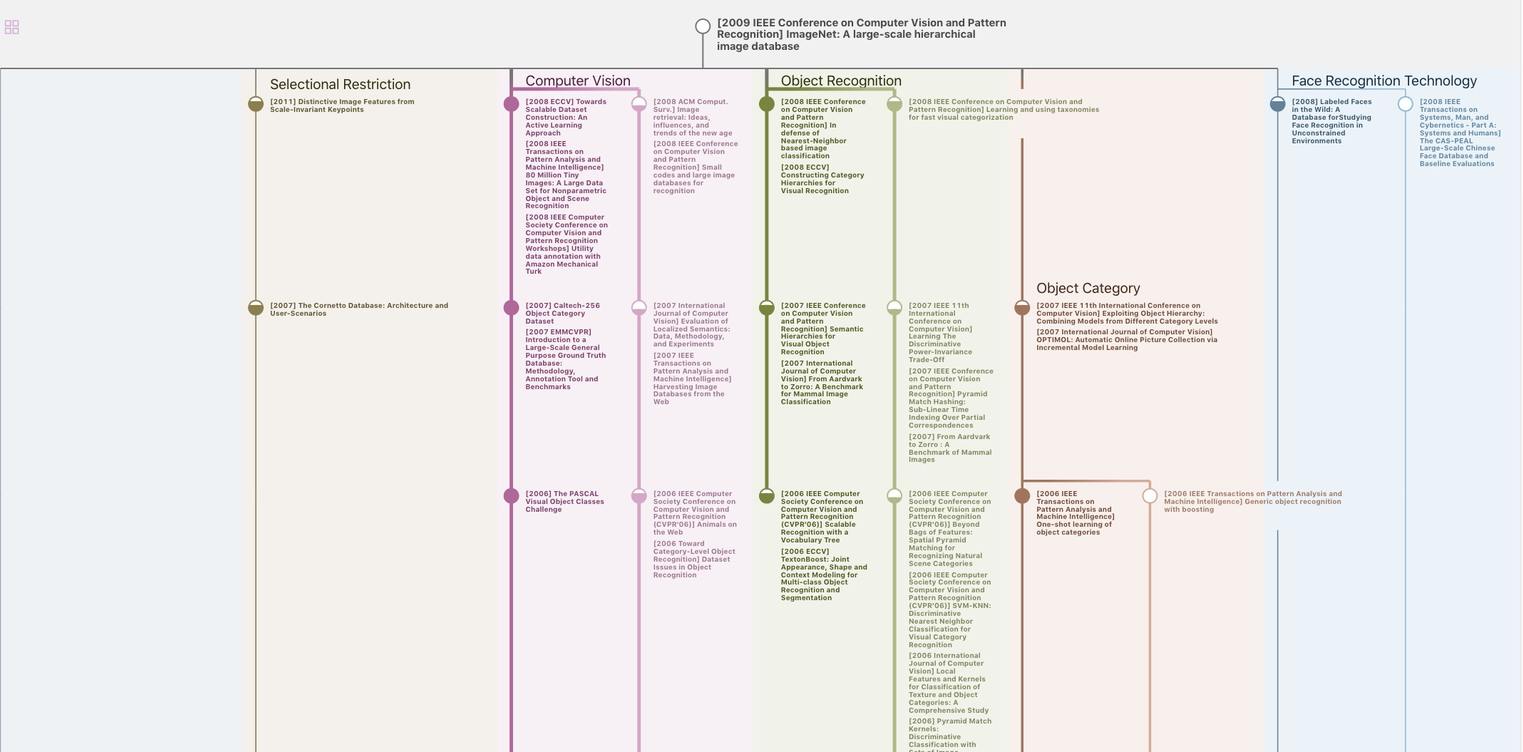
生成溯源树,研究论文发展脉络
Chat Paper
正在生成论文摘要