Machine Learning Classification Model for Network Based Intrusion Detection System
International Conference for Internet Technology and Secured Transactions (ICITST)(2016)
Univ Jyvaskyla
Abstract
With an enormous increase in number of mobile users, mobile threats are also growing rapidly. Mobile malwares can lead to several cybersecurity threats i.e. stealing sensitive information, installing backdoors, ransomware attacks and sending premium SMSs etc. Previous studies have shown that due to the sophistication of threats and tailored techniques to avoid detection, not every antivirus system is capable of detecting advance threats. However, an extra layer of security at the network side can protect users from these advanced threats by analyzing the traffic patterns. To detect these threats, this paper proposes and evaluates, a Machine Learning (ML) based model for Network based Intrusion Detection Systems (NIDS). In this research, several supervised ML classifiers were built using data-sets containing labeled instances of network traffic features generated by several malicious and benign applications. The focus of this research is on Android based malwares due to its global share in mobile malware and popularity among users. Based on the evaluation results, the model was able to detect known and unknown threats with the accuracy of up to 99.4%. This ML model can also be integrated with traditional intrusion detection systems in order to detect advanced threats and reduce false positives.
MoreTranslated text
Key words
Intrusion Detection,Machine Learning,Mobile Malware,Traffic Analysis,Anomaly Detection
求助PDF
上传PDF
View via Publisher
AI Read Science
AI Summary
AI Summary is the key point extracted automatically understanding the full text of the paper, including the background, methods, results, conclusions, icons and other key content, so that you can get the outline of the paper at a glance.
Example
Background
Key content
Introduction
Methods
Results
Related work
Fund
Key content
- Pretraining has recently greatly promoted the development of natural language processing (NLP)
- We show that M6 outperforms the baselines in multimodal downstream tasks, and the large M6 with 10 parameters can reach a better performance
- We propose a method called M6 that is able to process information of multiple modalities and perform both single-modal and cross-modal understanding and generation
- The model is scaled to large model with 10 billion parameters with sophisticated deployment, and the 10 -parameter M6-large is the largest pretrained model in Chinese
- Experimental results show that our proposed M6 outperforms the baseline in a number of downstream tasks concerning both single modality and multiple modalities We will continue the pretraining of extremely large models by increasing data to explore the limit of its performance
Upload PDF to Generate Summary
Must-Reading Tree
Example
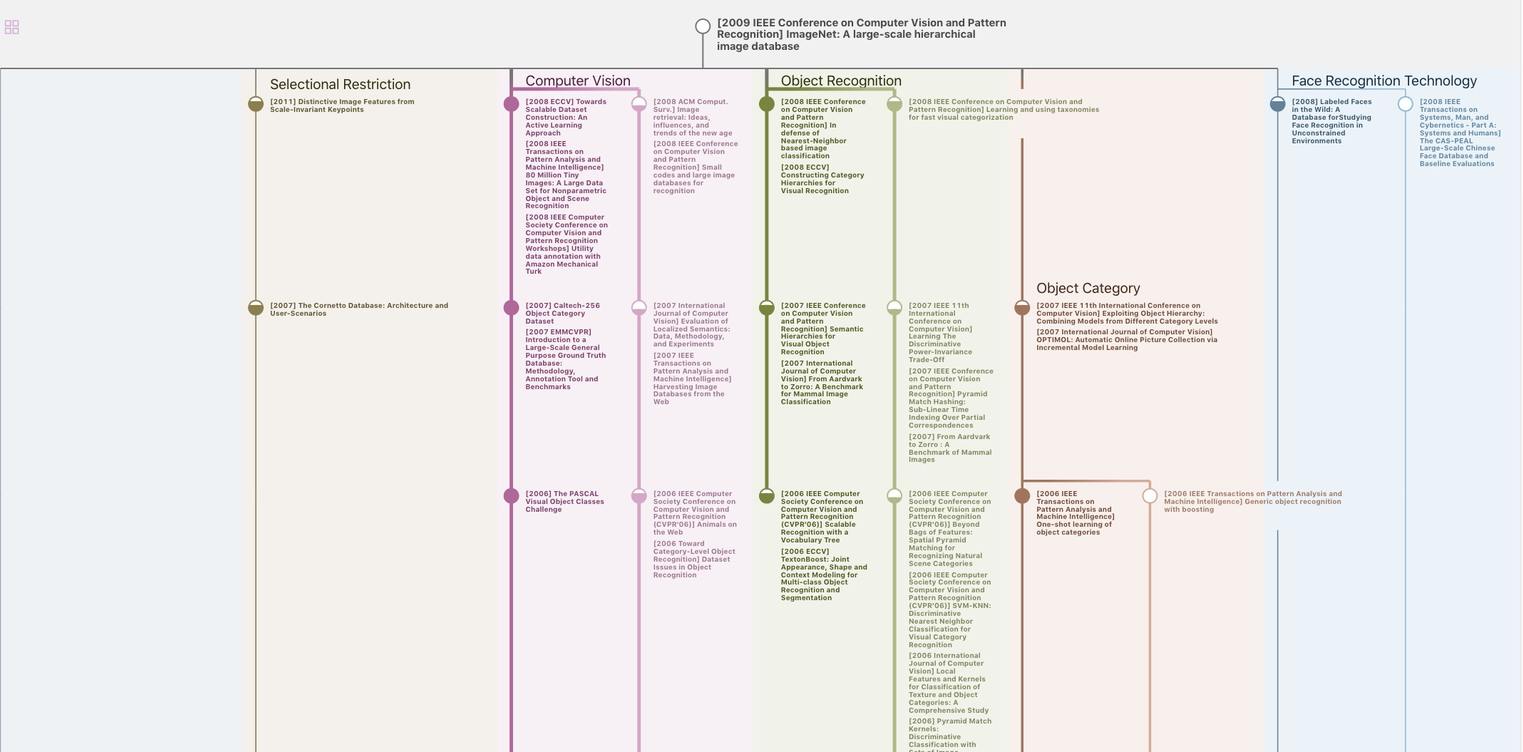
Generate MRT to find the research sequence of this paper
Related Papers
2012
被引用83 | 浏览
Data Disclaimer
The page data are from open Internet sources, cooperative publishers and automatic analysis results through AI technology. We do not make any commitments and guarantees for the validity, accuracy, correctness, reliability, completeness and timeliness of the page data. If you have any questions, please contact us by email: report@aminer.cn
Chat Paper
GPU is busy, summary generation fails
Rerequest