A Joint Sparse Clustering And Classification Approach With Applications To Hospitalization Prediction
2016 IEEE 55TH CONFERENCE ON DECISION AND CONTROL (CDC)(2016)
摘要
We develop a new method for a particular type of a classification problem, where the positive class is a mixture of multiple clusters and the negative class is drawn from a single cluster. The new method employs an alternating optimization approach, which jointly discovers the clusters in the positive class, and at the same time, optimizes the classifiers that separate each positive cluster from the negative samples. The classifiers are designed under the Support Vector Machines (SVM) framework with double regularizations, and the whole alternating process is shown to converge. We compare this new method to the conventional SVM with a linear kernel or an RBF kernel and two other hierarchical classifiers which first cluster once and then classify. Experimental results with both simulated data and actual data demonstrate better prediction accuracy, as well as, successful cluster detection.
更多查看译文
关键词
Clustering, classification, large-scale problems, healthcare, predictive analytics, heart diseases
AI 理解论文
溯源树
样例
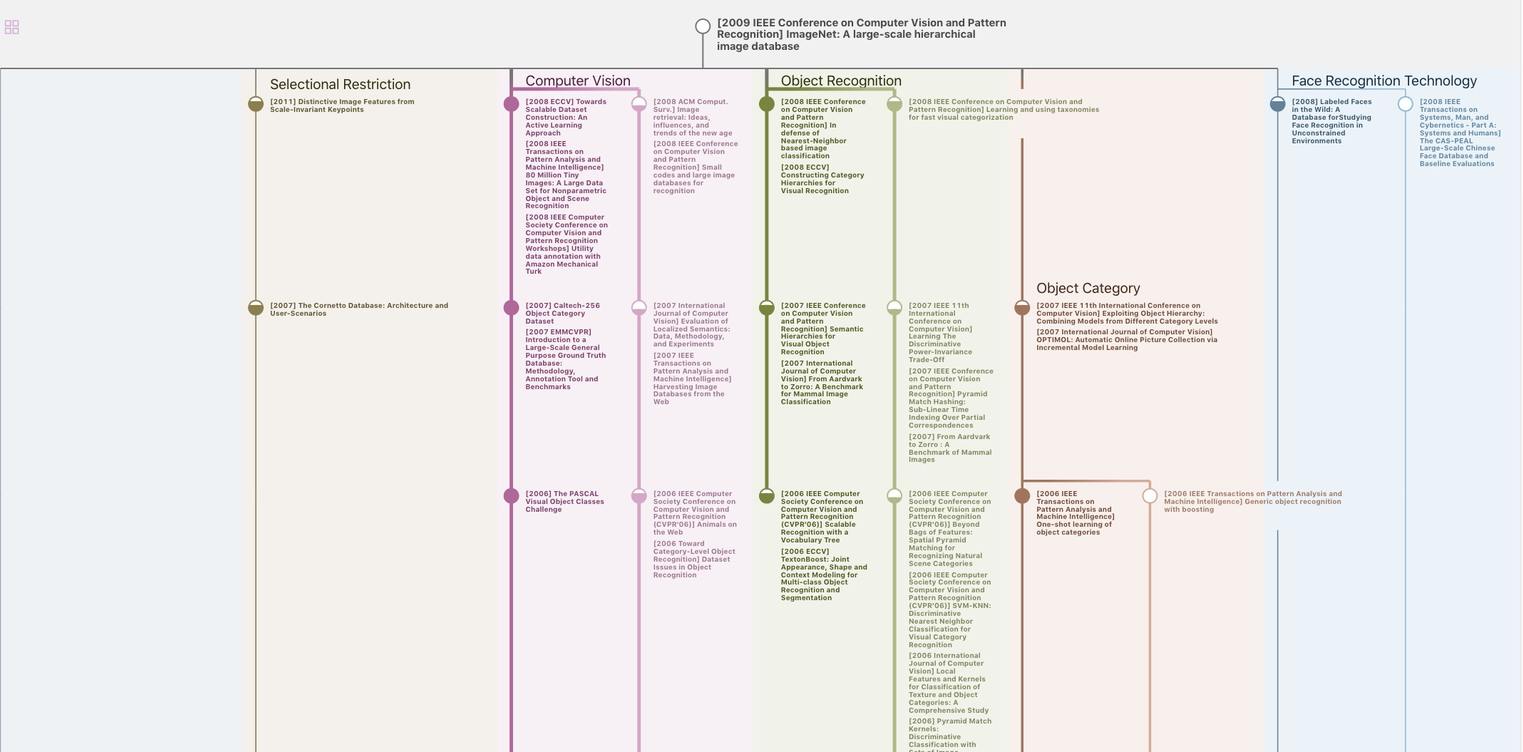
生成溯源树,研究论文发展脉络
Chat Paper
正在生成论文摘要