Data-Driven Prediction Of Evar With Confidence In Time-Varying Datasets
2016 IEEE 55TH CONFERENCE ON DECISION AND CONTROL (CDC)(2016)
摘要
The key challenge for learning-based autonomous systems operating in time-varying environments is to predict when the learned model may lose relevance. If the learned model loses relevance, then the autonomous system is at risk of making wrong decisions. The entropic value at risk (EVAR) is a computationally efficient and coherent risk measure that can be utilized to quantify this risk. In this paper, we present a Bayesian model and learning algorithms to predict the state-dependent EVAR of time-varying datasets. We discuss applications of EVAR to an exploration problem in which an autonomous agent has to choose a set of sensing locations in order to maximize the informativeness of the acquired data and learn a model of an underlying phenomenon of interest. We empirically demonstrate the efficacy of the presented model and learning algorithms on four real-world datasets.
更多查看译文
关键词
Robot sensing systems,Predictive models,Hidden Markov models,Computational modeling,Uncertainty,Data models
AI 理解论文
溯源树
样例
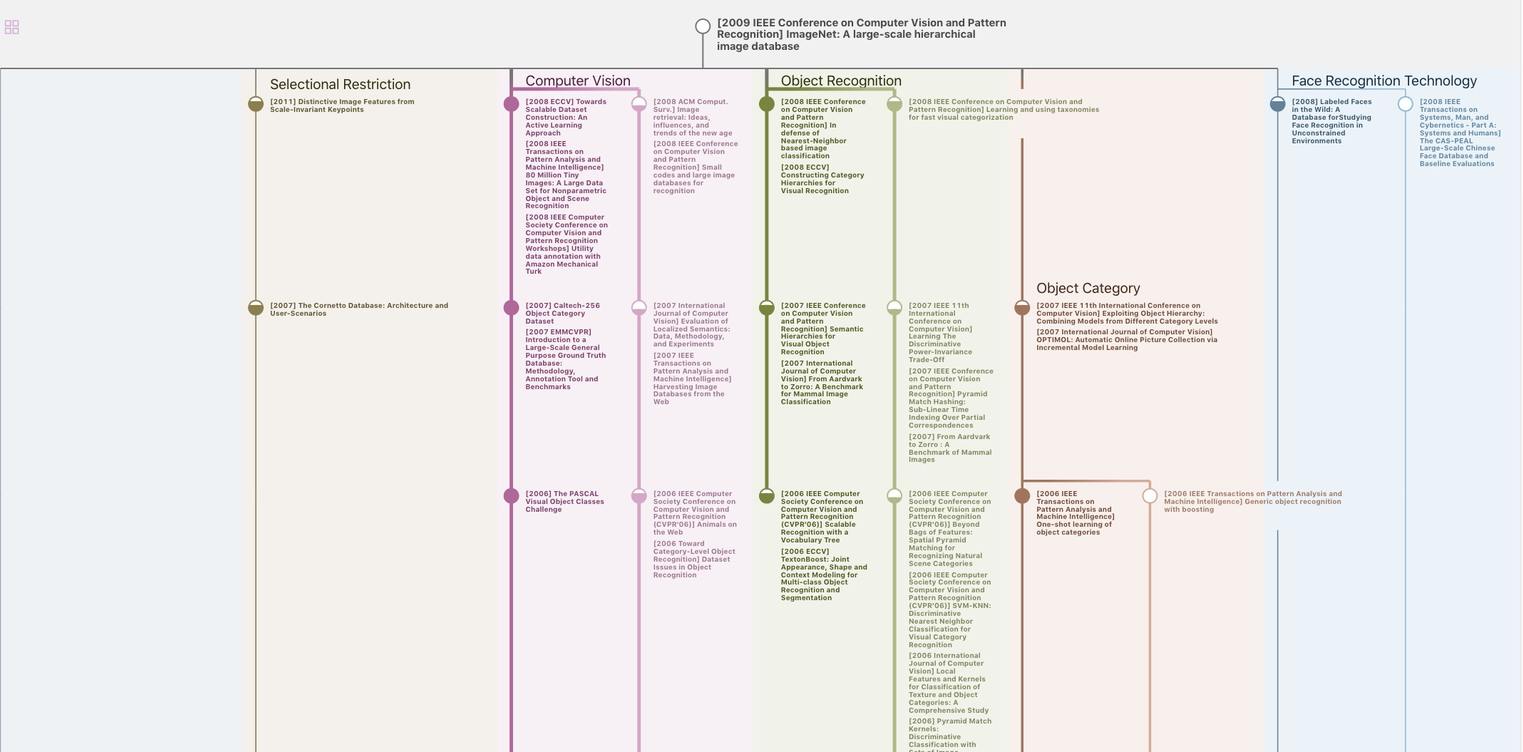
生成溯源树,研究论文发展脉络
Chat Paper
正在生成论文摘要