Particle-Based Gaussian Process Optimization For Input Design In Nonlinear Dynamical Models
2016 IEEE 55TH CONFERENCE ON DECISION AND CONTROL (CDC)(2016)
摘要
We propose a novel approach to input design for identification of nonlinear state space models. The optimal input sequence is obtained by maximizing a scalar cost function of the Fisher information matrix. Since the Fisher information matrix is unavailable in closed form, it is estimated using particle methods. In addition, we make use of Gaussian process optimization to find the optimal input and to mitigate the problem of a large computational cost incurred by the particle method, as the method reduces the number of functional evaluations. Numerical examples are provided to illustrate the performance of the resulting algorithm.
更多查看译文
关键词
System identification, input design, Gaussian process optimization
AI 理解论文
溯源树
样例
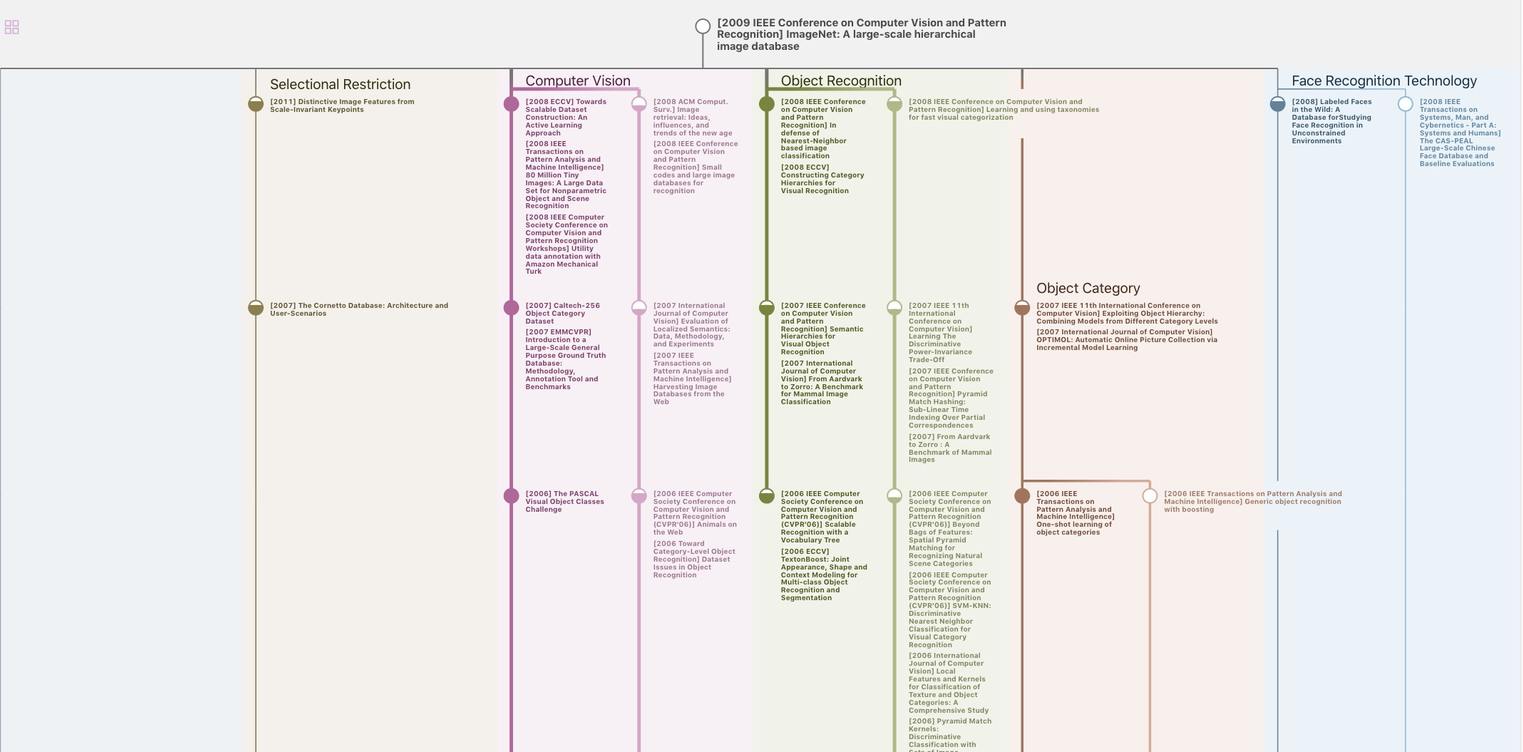
生成溯源树,研究论文发展脉络
Chat Paper
正在生成论文摘要