Two-Stage Pooling Of Deep Convolutional Features For Image Retrieval
2016 IEEE International Conference on Image Processing (ICIP)(2016)
摘要
Convolutional Neural Network (CNN) based image representations have achieved high performance in image retrieval tasks. However, traditional CNN based global representations either provide high dimensional features, which incurs large memory consumption and computing cost, or inadequately capture discriminative information in images, which degenerates the functionality of CNN features. To address those issues, we propose a two-stage partial mean pooling (PMP) approach to construct compact and discriminative global feature representations. The proposed PMP is meant to tackle the limits of traditional max pooling and mean (or average) pooling. By injecting the PMP pooling strategy into the CNN based patch-level mid-level feature extraction and representation, we have significantly improved the state-of-the-art retrieval performance over several common benchmark datasets.
更多查看译文
关键词
image representation,image retrieval,convolutional neural network,feature pooling,compact descriptor
AI 理解论文
溯源树
样例
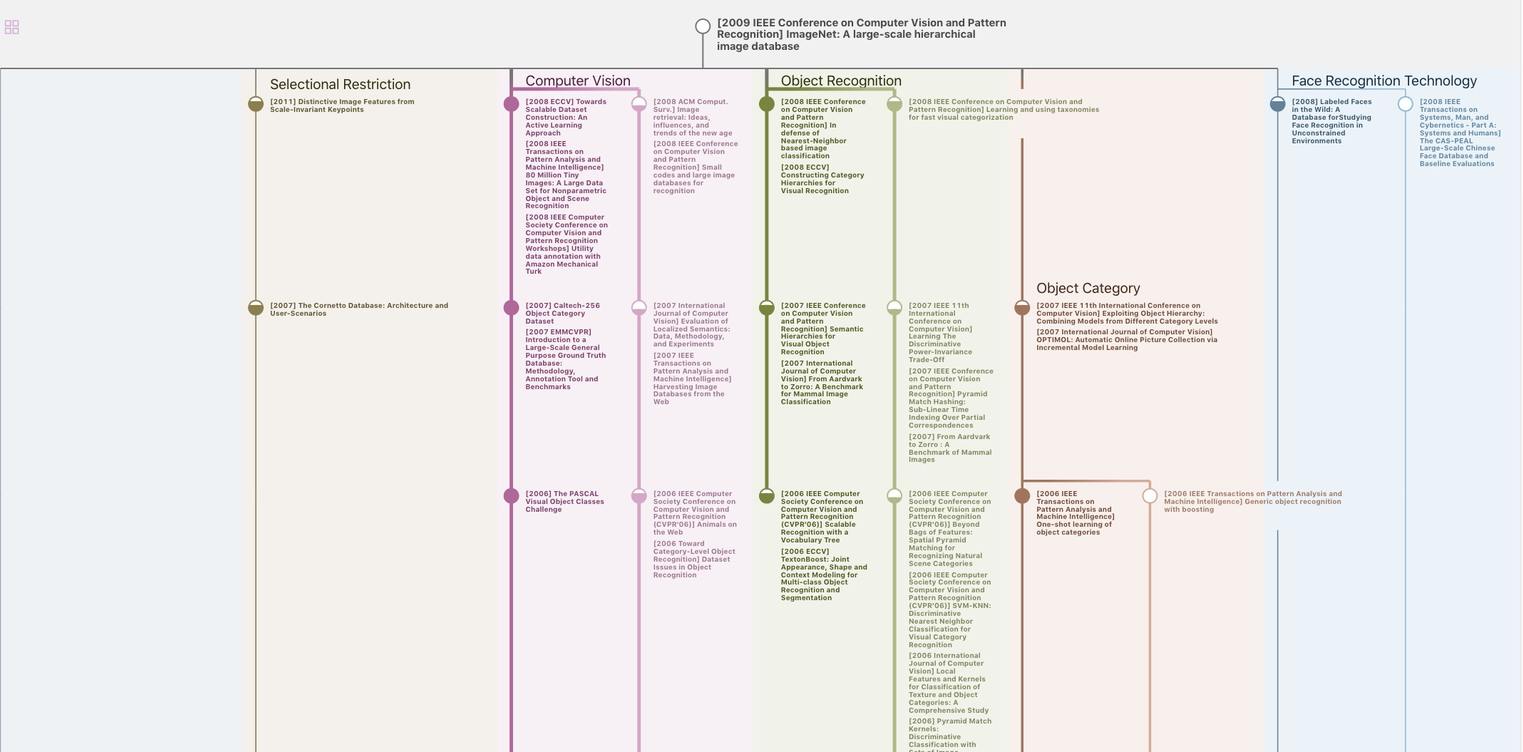
生成溯源树,研究论文发展脉络
Chat Paper
正在生成论文摘要