Leveraging 2D and 3D cues for fine-grained object classification
2016 IEEE INTERNATIONAL CONFERENCE ON IMAGE PROCESSING (ICIP)(2016)
摘要
Objects in fine-grained categories always share a high degree of shape similarity, making both “localizing discriminative parts” and “learning appearance descriptors” extremely difficult. We propose a framework to leverage 2D+3D cues to handle above two challenges. Towards the goal of image alignment to localize discriminative parts, traditional methods rely on either manual part annotation or image segmentation. Instead, our framework leverages each image's 3D camera pose estimation to align images; Towards the goal of “learning appearance descriptors” confined with small training data and memory/computation cost, we propose an unsupervised Convolutional Sparse Coding (CSC) + manifold learning that significantly reduces model complexity, but still successfully produces highly diverse feature filters like deep neural network. Our experimental results attest the advocated framework's accuracy is comparable to a deep network, demonstrating its great potential on mobile devices.
更多查看译文
关键词
Fine-grained object classification, 2D+3D cues, convolutional sparse coding
AI 理解论文
溯源树
样例
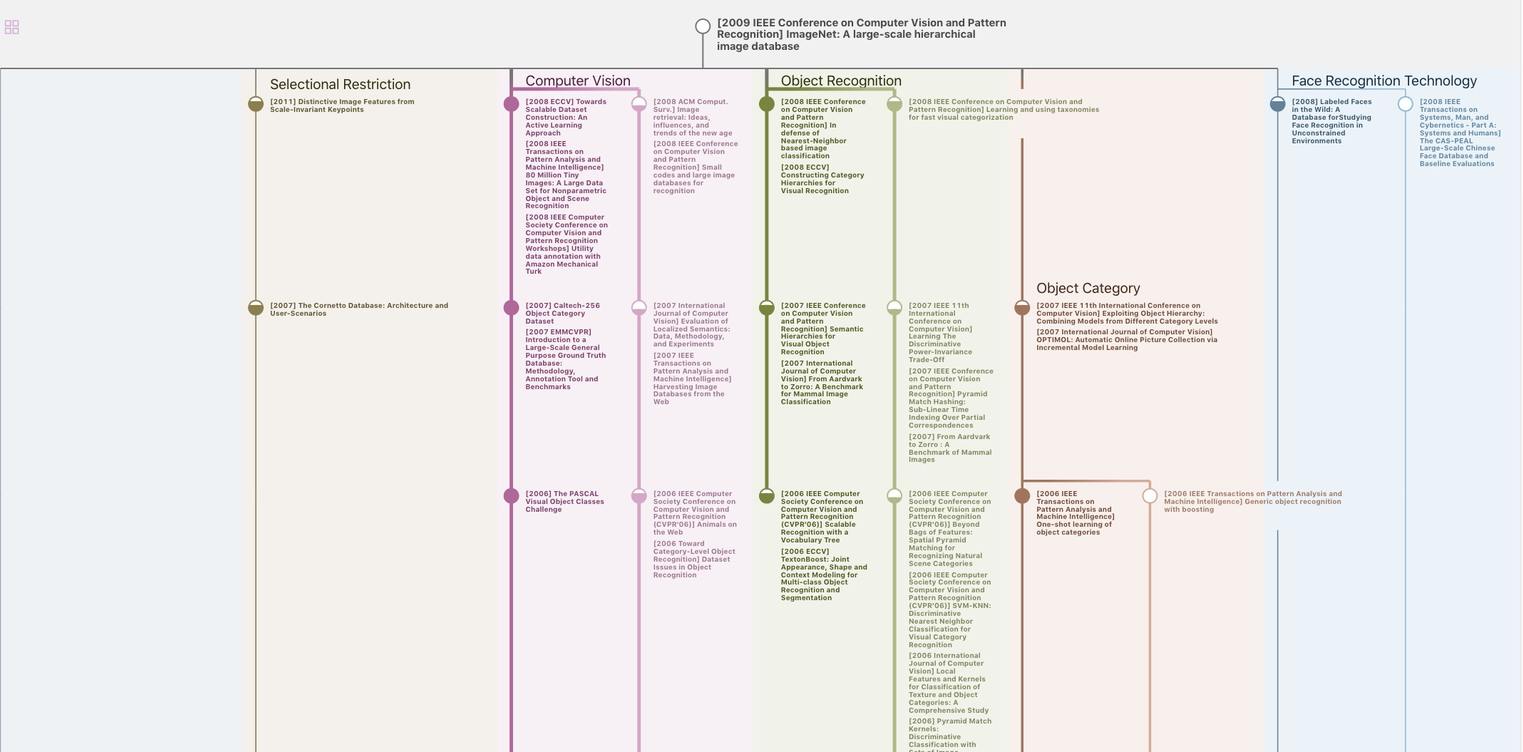
生成溯源树,研究论文发展脉络
Chat Paper
正在生成论文摘要