Low-Rank And Structured Sparse Subspace Clustering
IEEE Conference Proceedings(2016)
摘要
High dimensional data often lie approximately in low dimensional subspaces corresponding to multiple classes or categories. Segmenting the high dimensional data into their corresponding low dimensional subspaces is referred as subspace clustering. State of the art methods solve this problem in two steps. First, an affinity matrix is built from data based on self expressiveness model, in which each data point is expressed as a linear combination of other data points. Second, the segmentation is obtained by spectral clustering. However, solving two dependent steps separately is still suboptimal. In this paper, we propose a joint affinity learning and spectral clustering approach for low rank representation based subspace clustering, termed Low-Rank and Structured Sparse Subspace Clustering (LRS3C), where a subspace structured norm that depends on subspace clustering result is introduced into the objective of low-rank representation problem. We solve it efficiently via a combination of Linearized Alternation Direction Method (LADM) with spectral clustering. Experiments on Hopkins 155 motion segmentation database and Extended Yale B data set demonstrated the effectiveness of our method.
更多查看译文
关键词
high dimensional data,low dimensional subspaces,affinity matrix,self-expressiveness model,spectral clustering,affinity learning,low-rank representation based subspace clustering,low-rank and structured sparse subspace clustering,LRS3C,linearized alternation direction method,LADM
AI 理解论文
溯源树
样例
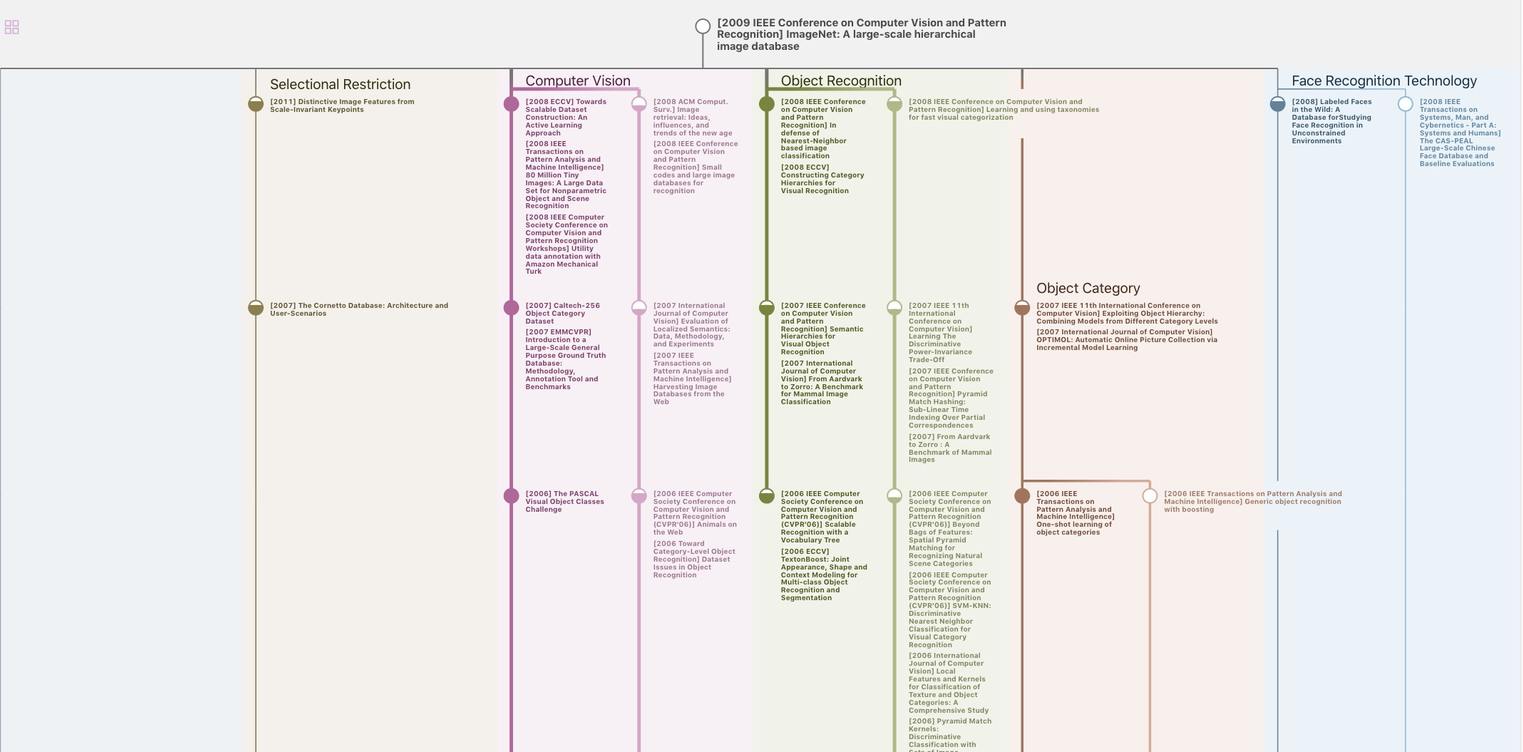
生成溯源树,研究论文发展脉络
Chat Paper
正在生成论文摘要