Augmenting Deep Convolutional Neural Networks With Depth-Based Layered Detection For Human Detection
2016 IEEE/RSJ International Conference on Intelligent Robots and Systems (IROS)(2016)
摘要
Deep convolutional neural networks are being increasingly deployed for image classification tasks as they can learn sensor and environmental independence from large quantities of training data. Most, however, have focused on classifying uploaded photographs rather than the often occluded, arbitrary height and camera angles images found commonly in robotic applications. In this work, we look at the performance of the popular AlexNet architecture to detect people in different robotic scenarios using different sensors and/or environments. Furthermore, we demonstrate how fusing this architecture with the depth-based layered detection system, a more traditional geometric feature-based classifier, leads to significant improvements in classification precision/recall, whether working with depth data alone or a combination of depth and RGB images.
更多查看译文
关键词
deep convolutional neural networks,depth-based layered detection,human detection,image classification task,robotic applications,AlexNet architecture,geometric feature-based classifier,classification precision,classification recall,depth images,RGB images,red-green-blue images
AI 理解论文
溯源树
样例
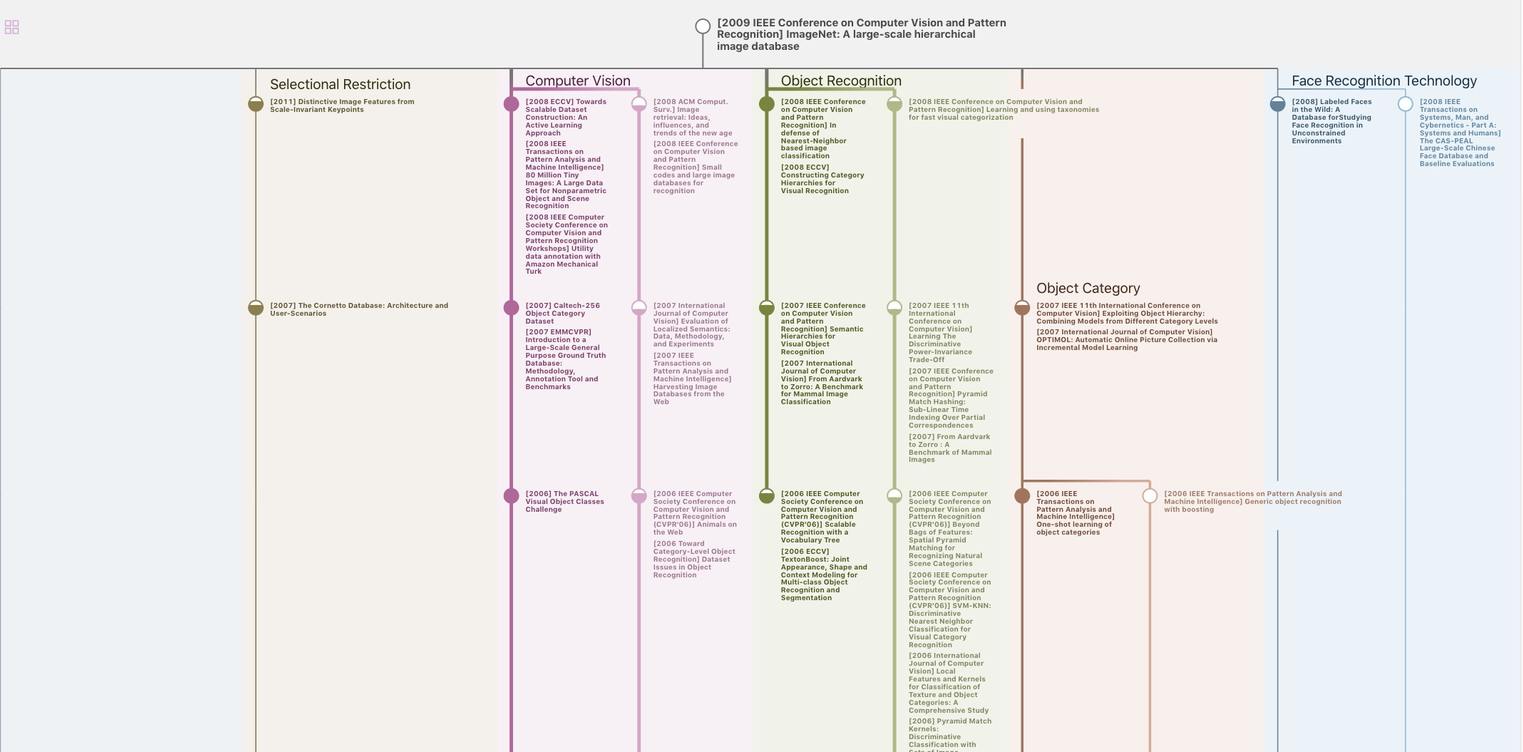
生成溯源树,研究论文发展脉络
Chat Paper
正在生成论文摘要