Efficient deep neural networks for speech synthesis using bottleneck features.
Asia-Pacific Signal and Information Processing Association Annual Summit and Conference(2016)
摘要
This paper proposes a cascading deep neural network (DNN) structure for speech synthesis system that consists of text-to-bottleneck (TTB) and bottleneck-to-speech (BTS) models. Unlike conventional single structure that requires a large database to find complicated mapping rules between linguistic and acoustic features, the proposed structure is very effective even if the available training database is inadequate. The bottleneck feature utilized in the proposed approach represents the characteristics of linguistic features and its average acoustic features of several speakers. Therefore, it is more efficient to learn a mapping rule between bottleneck and acoustic features than to learn directly a mapping rule between linguistic and acoustic features. Experimental results show that the learning capability of the proposed structure is much higher than that of the conventional structures. Objective and subjective listening test results also verify the superiority of the proposed structure.
更多查看译文
关键词
deep neural networks,speech synthesis,bottleneck features,text-to-bottleneck model,bottleneck-to-speech model,training database,mapping rule
AI 理解论文
溯源树
样例
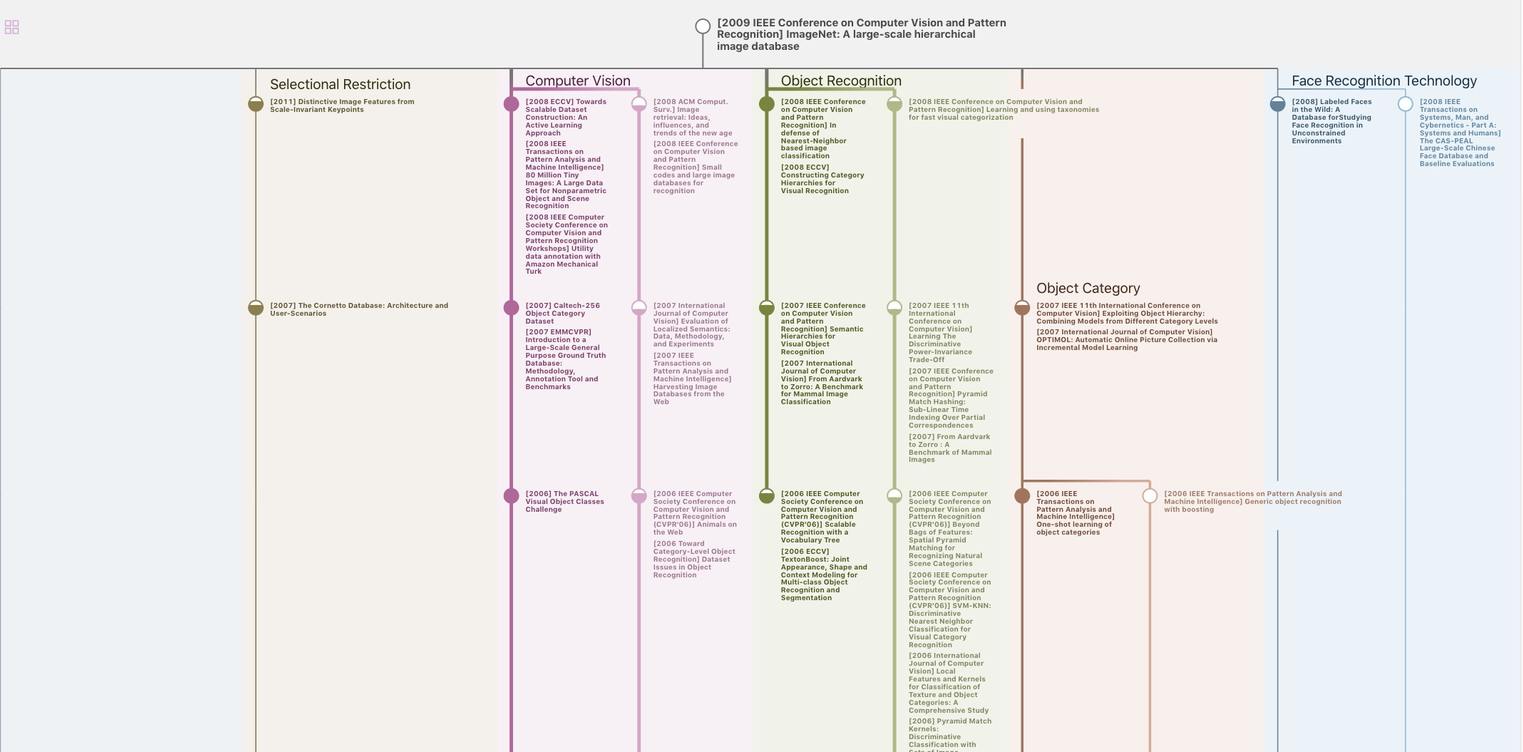
生成溯源树,研究论文发展脉络
Chat Paper
正在生成论文摘要