Using a CNN ensemble for detecting pornographic and upskirt images
2016 IEEE 8th International Conference on Biometrics Theory, Applications and Systems (BTAS)(2016)
摘要
The recent popularity of smartphones causes significant increase of upskirt filming cases in many countries and regions. To search upskirt images in suspects' IT devices and block them on social media, an effective detector is demanded, but it is neglected by both academic and commercial communities. Three commercial pornographic image detectors and one detector distributed by the U.S. National Institute of Justice are first examined on upskirt images. They classify 24.56%-41.90% of the testing upskirt images as pornographic images and the rest as normal images. These results imply that the detectors cannot be used to search upskirt images. To effectively use unbalanced training data, a convolution neural network (CNN) ensemble is proposed for classifying pornographic, upskirt and normal images. Training images for this classification problem are always unbalanced, because pornographic and normal images are numerous on the Internet, but upskirt images are significantly fewer. In this study, the ensemble is formed by multiple CNNs, which are trained on balanced datasets with different pornographic and normal images, but the same upskirt images. The probabilities from the CNNs are fused as the ensemble outputs. To train and test the CNN ensemble, 10,997 pornographic images, 1,673 upskirt images and 38,832 normal images are collected from the Internet. Experimental results show that the ensemble with six CNNs outperforms a single CNN by 2.93% and achieves detection accuracy of 90.23%. Comparing with the four pornographic image detectors, the ensemble also performs better in classifying pornographic images from normal images, with a significant margin of over 10%.
更多查看译文
关键词
CNN ensemble,upskirt images,pornographic image detectors,convolution neural network ensemble,image classification problem,probabilities,Internet
AI 理解论文
溯源树
样例
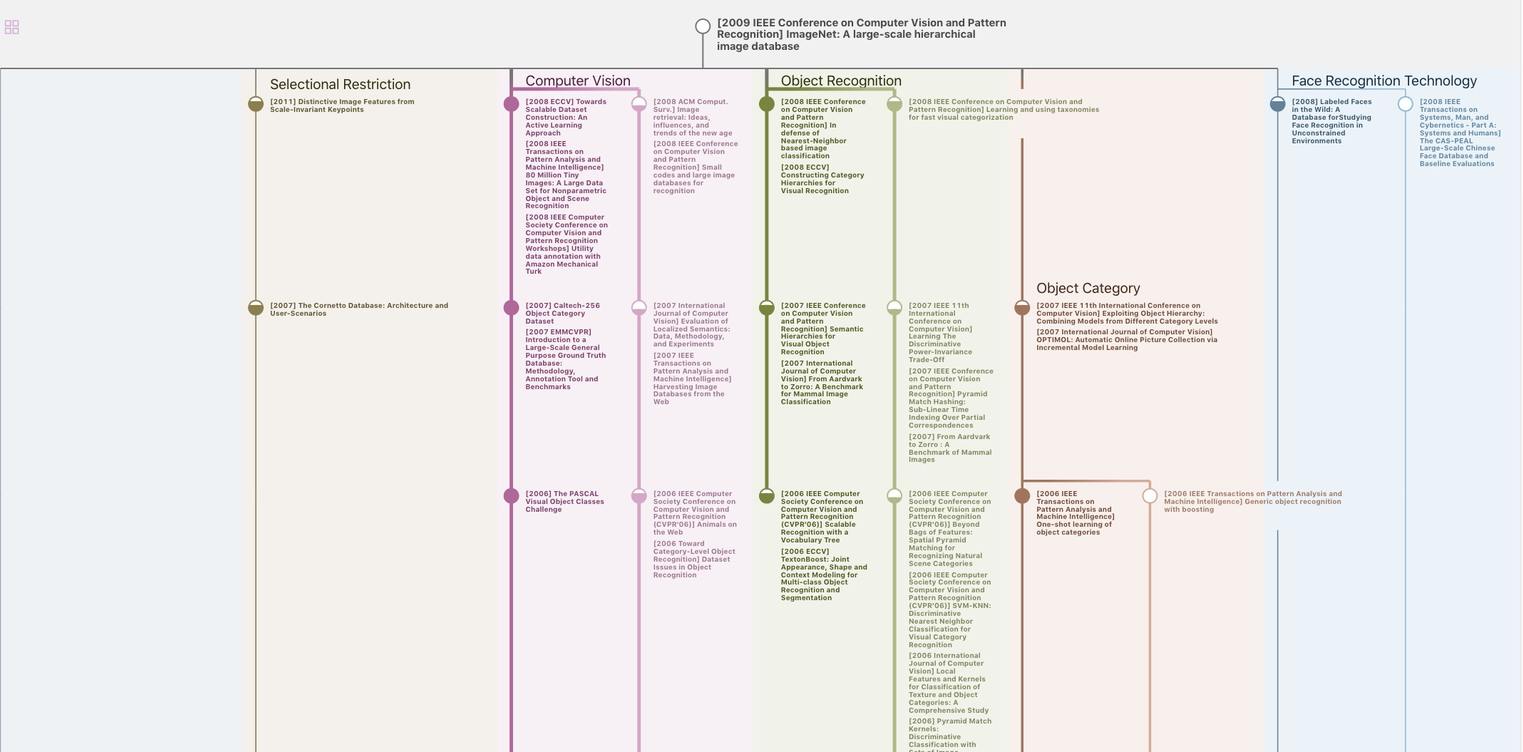
生成溯源树,研究论文发展脉络
Chat Paper
正在生成论文摘要