Latent Label Consistent K-Svd For Joint Machine Faults Representation And Classification
2016 IEEE 14th International Conference on Industrial Informatics (INDIN)(2016)
摘要
We propose a new discriminative dictionary learning framework termed Latent Label Consistent K-SVD (LLC-KSVD) for representing and classifying machine faults. Our LLC-KSVD handles the task by minimizing the reconstruction, discriminative sparse-code and classification errors at the same time. To enhance the representation and classification powers, LLC-KSVD aim to decompose given data into a sparse reconstruction part, a salient feature part and an error part. The salient features are learnt by embedding data onto a projection and a classifier is then trained over extracted salient features so that features are ensured to be optimal for classification. Thus, the classification approach of our LLC-KSVD is very efficient, since there is no need to involve a time-consuming sparse reconstruction process with well-trained dictionary for each test signal as existing models. Besides, to make the classifier be robust to noise and outliers, we regularize the l(2,1)-norm on classifier so that the predictions are more accurate. Simulations on several machine fault datasets demonstrate the state-of-the-art performance by our LLC-KSVD.
更多查看译文
关键词
label consistent dictionary learning,feature extraction,machine faults representation and classification
AI 理解论文
溯源树
样例
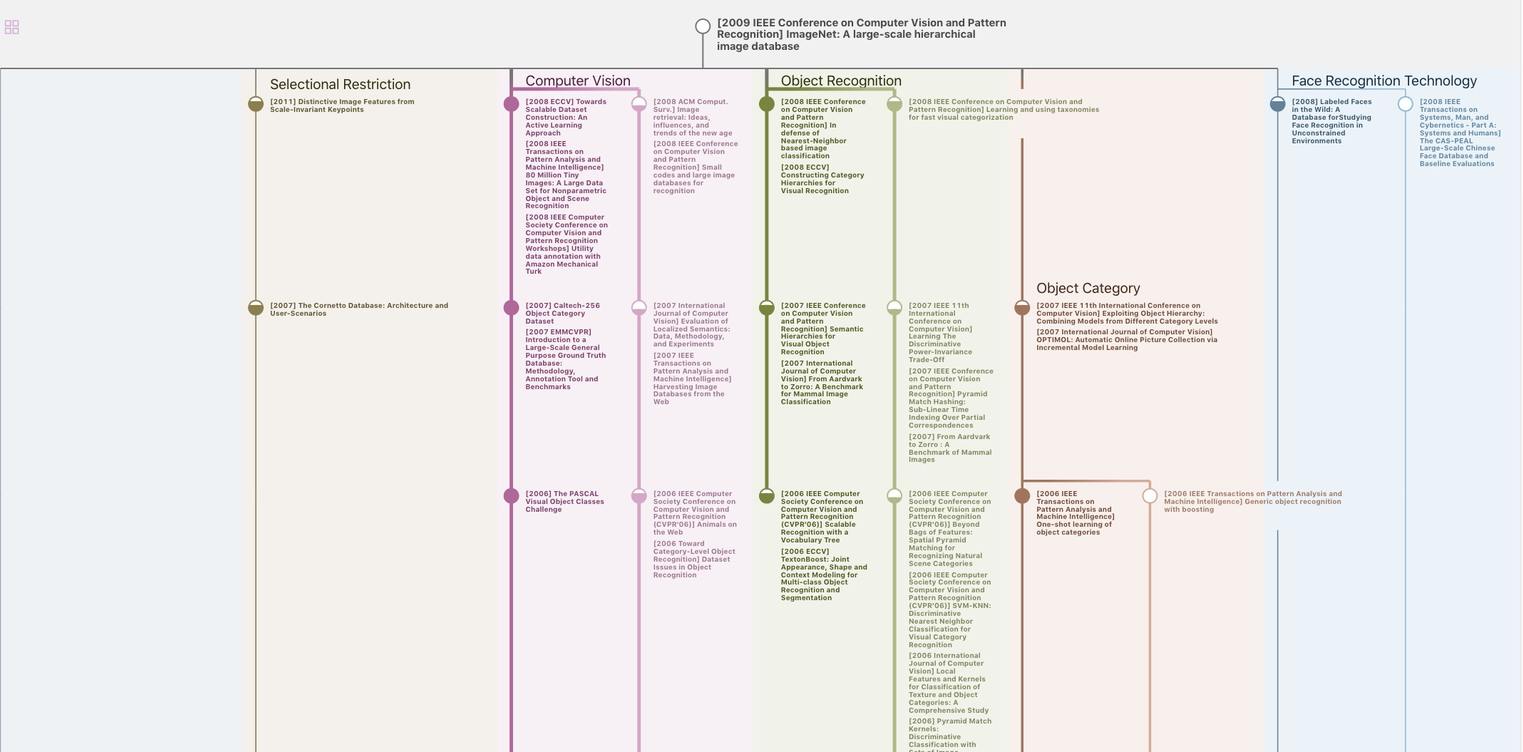
生成溯源树,研究论文发展脉络
Chat Paper
正在生成论文摘要