A Validity Index Method For Clusters With Different Degrees Of Dispersion And Overlap
2016 EIGHTH INTERNATIONAL CONFERENCE ON ADVANCED COMPUTATIONAL INTELLIGENCE (ICACI)(2016)
摘要
Cluster validity index is used for estimating the quality of partitions to a dataset by clustering algorithms, and finding the optimal number of clusters to be partitioned. In this paper, we propose a new validity index, which is based on a dispersion measure and an overlap measure. The dispersion measure estimates the overall data density of the clusters in the dataset; whereas the overlap measure estimates the degree of isolation among all clusters. Low degree of dispersion means that the overall clusters are densely distributed and hence are compact; and low degree of overlap means that clusters are overall well separated. Thus, a good clustering result is expected to have a lower dispersion measure and a lower overlap measure. We conducted several experiments to validate the effectiveness of our validity indexing method, including artificial datasets and public real datasets. Experimental results show that our validity indexing method has superior effectiveness and reliability for estimating the optimal number of clusters that widely differ in degrees of dispersion and overlap, when compared to nine other indices proposed in the literature.
更多查看译文
关键词
Cluster validity index,fuzzy C-means,dispersion measure,overlap measure
AI 理解论文
溯源树
样例
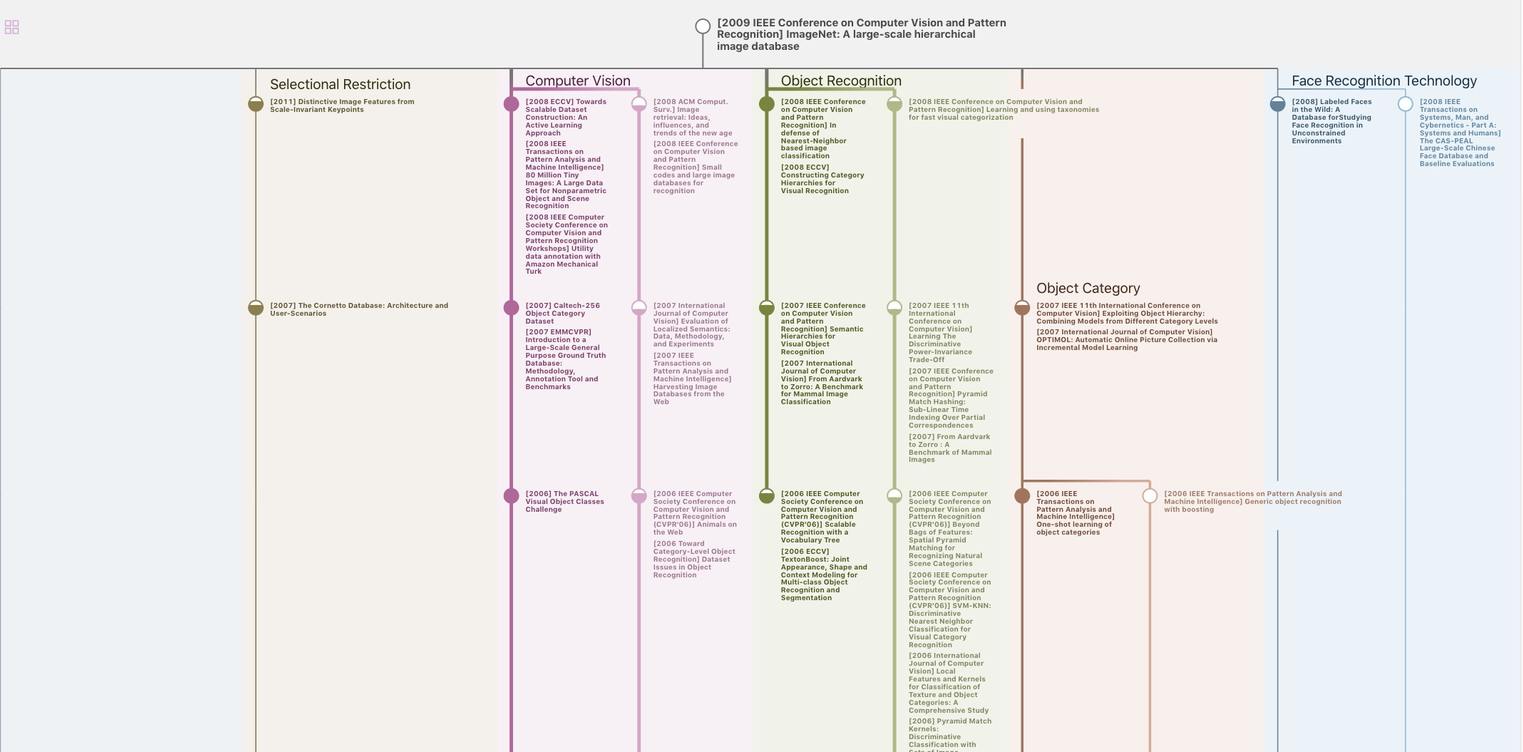
生成溯源树,研究论文发展脉络
Chat Paper
正在生成论文摘要