Maximization of Approximately Submodular Functions.
ADVANCES IN NEURAL INFORMATION PROCESSING SYSTEMS 29 (NIPS 2016)(2016)
摘要
We study the problem of maximizing a function that is approximately submodular under a cardinality constraint. Approximate submodularity implicitly appears in a wide range of applications as in many cases errors in evaluation of a submodular function break submodularity. Say that F is epsilon-approximately submodular if there exists a submodular function f such that (1 - epsilon)f (S) <= F(S) <= (1+epsilon) f (S) for all subsets S. We are interested in characterizing the query-complexity of maximizing F subject to a cardinality constraint k as a function of the error level epsilon > 0. We provide both lower and upper bounds: for epsilon > n(-1/2) we show an exponential query-complexity lower bound. In contrast, when epsilon < 1/k or under a stronger bounded curvature assumption, we give constant approximation algorithms.
更多查看译文
AI 理解论文
溯源树
样例
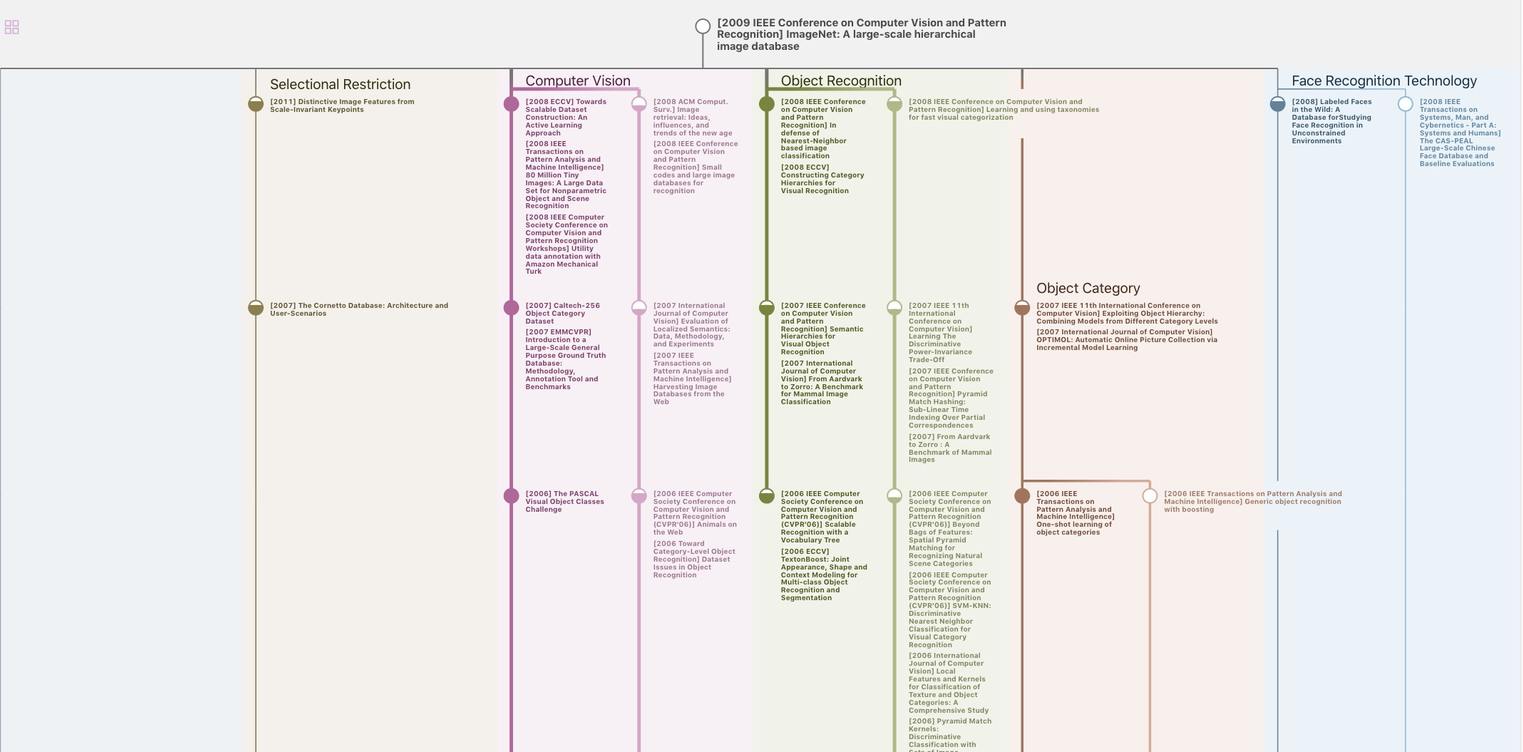
生成溯源树,研究论文发展脉络
Chat Paper
正在生成论文摘要