Minimizing Regret On Reflexive Banach Spaces And Nash Equilibria In Continuous Zero-Sum Games
NIPS'16: Proceedings of the 30th International Conference on Neural Information Processing Systems(2016)
摘要
We study a general adversarial online learning problem, in which we are given a decision set X in a reflexive Banach space X and a sequence of reward vectors in the dual space of X. At each iteration, we choose an action from X, based on the observed sequence of previous rewards. Our goal is to minimize regret. Using results from infinite dimensional convex analysis, we generalize the method of Dual Averaging to our setting and obtain upper bounds on the worst-case regret that generalize many previous results. Under the assumption of uniformly continuous rewards, we obtain explicit regret bounds in a setting where the decision set is the set of probability distributions on a compact metric space S. Importantly, we make no convexity assumptions on either S or the reward functions. We also prove a general lower bound on the worst-case regret for any online algorithm. We then apply these results to the problem of learning in repeated two-player zero-sum games on compact metric spaces. In doing so, we first prove that if both players play a Hannan-consistent strategy, then with probability 1 the empirical distributions of play weakly converge to the set of Nash equilibria of the game. We then show that, under mild assumptions, Dual Averaging on the (infinite-dimensional) space of probability distributions indeed achieves Hannan-consistency.
更多查看译文
AI 理解论文
溯源树
样例
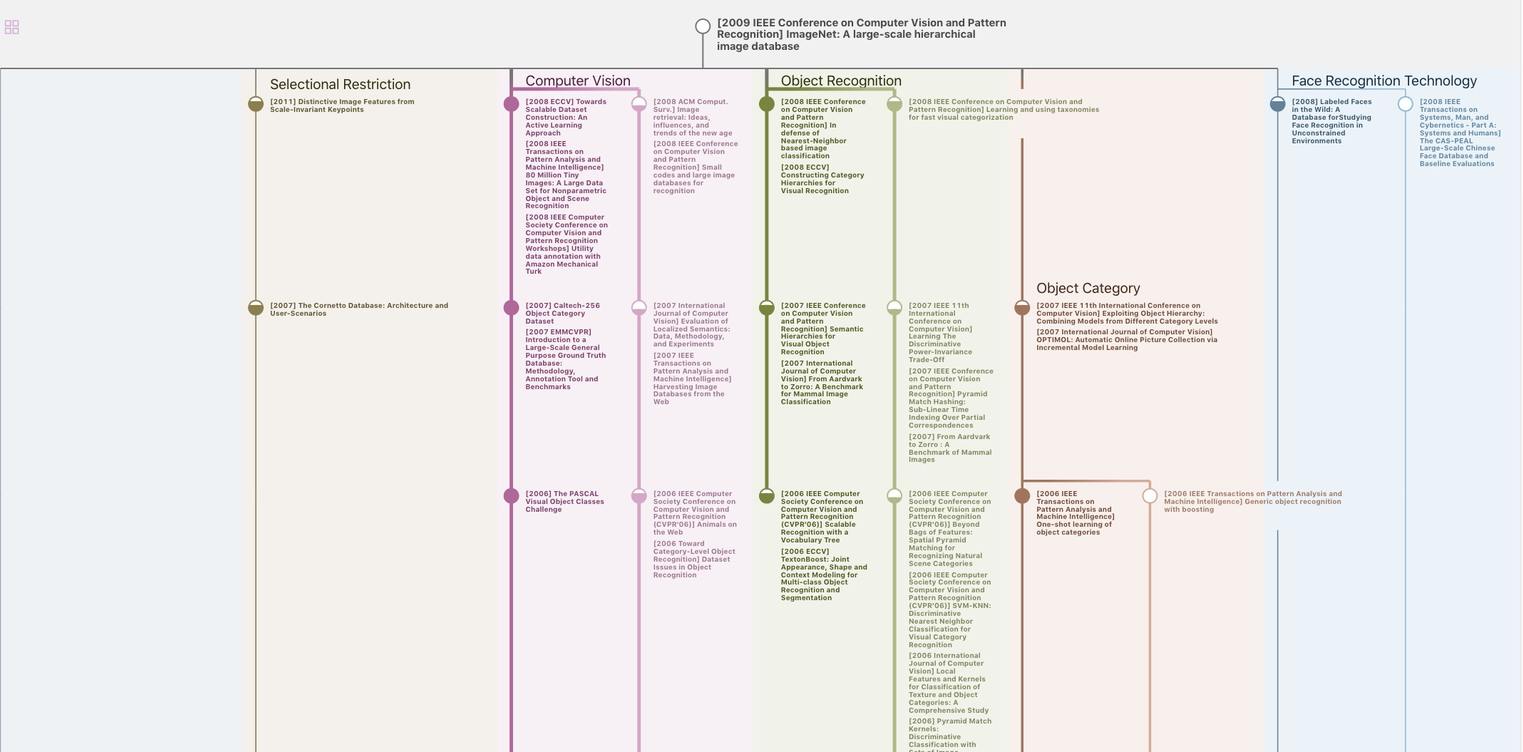
生成溯源树,研究论文发展脉络
Chat Paper
正在生成论文摘要