Droiddeeplearner: Identifying Android Malware Using Deep Learning
2016 IEEE 37TH SARNOFF SYMPOSIUM(2016)
摘要
With the proliferation of Android apps, encounters with malicious apps (malware) by mobile users are on the rise as vulnerabilities in the Android platform system are exploited by malware authors to access personal or sensitive information with ill intentions, often with financial gain in mind. To uphold security integrity and maintain user confidence, various approaches have been studied in the field of malware detection. As malware become more capable at hiding its malicious intent through the use of code obfuscation, it becomes imperative for malware detection techniques to keep up with the pace of malware changes. Currently, most of the existing malware detection approaches for Android platform use semantic pattern matching, which is highly effective but is limited to what the computers have encountered before. However, their performance degrades significantly when it comes to identifying malicious apps they have never tackled before. In this paper, we propose DroidDeepLearner, an Android malware characteri-zation and identification approach that uses deep learning algorithm to address the current need for malware detection to become more autonomous at learning to solve problems with less human intervention. Experimental results have shown that the DroidDeepLearner approach achieves good performance when compared to the existing widely used malware detection approaches.
更多查看译文
关键词
Android,malware,machine learning,deep learning
AI 理解论文
溯源树
样例
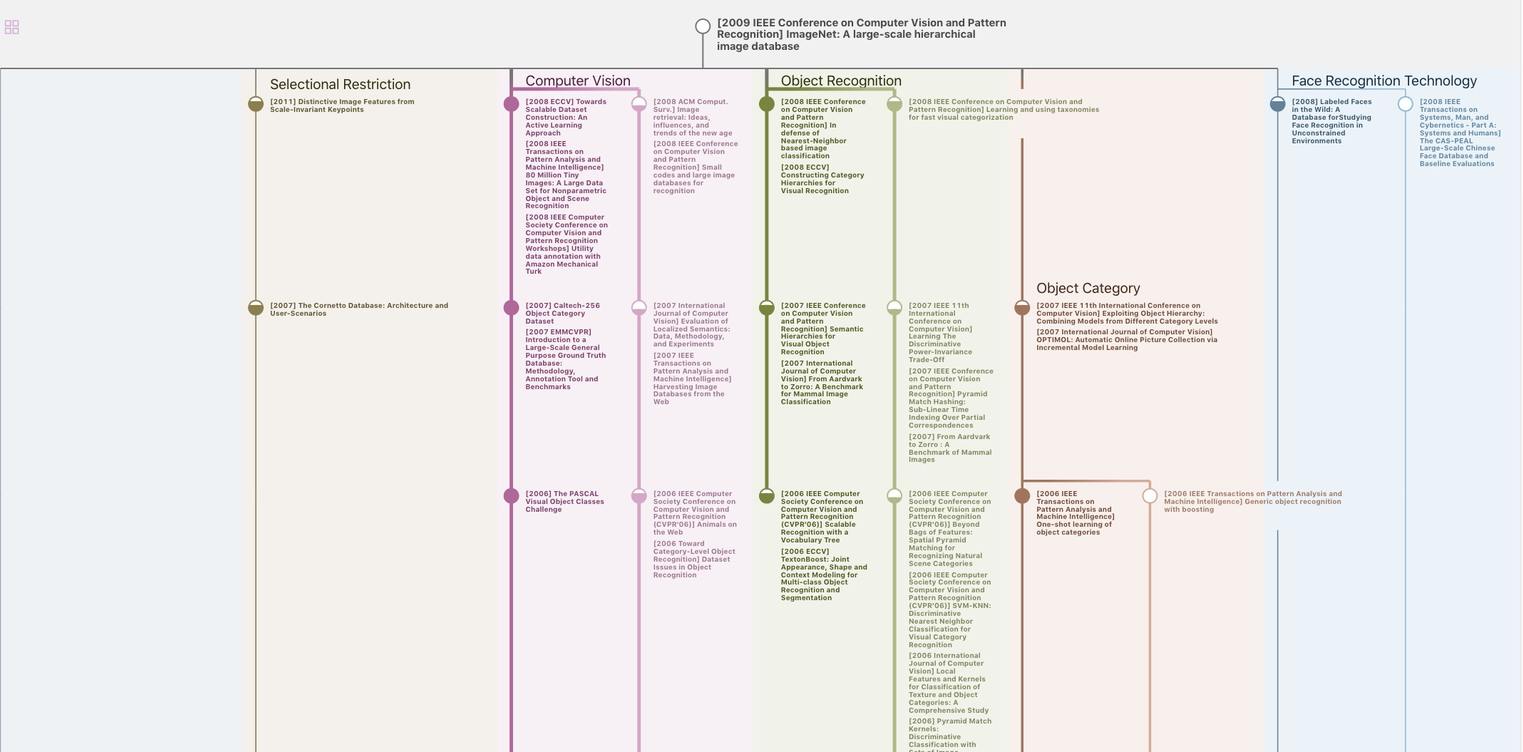
生成溯源树,研究论文发展脉络
Chat Paper
正在生成论文摘要