Monte Carlo profile confidence intervals for dynamic systems.
Journal of the Royal Society, Interface(2017)
摘要
Monte Carlo methods to evaluate and maximize the likelihood function enable the construction of confidence intervals and hypothesis tests, facilitating scientific investigation using models for which the likelihood function is intractable. When Monte Carlo error can be made small, by sufficiently exhaustive computation, then the standard theory and practice of likelihood-based inference applies. As datasets become larger, and models more complex, situations arise where no reasonable amount of computation can render Monte Carlo error negligible. We develop profile likelihood methodology to provide frequentist inferences that take into account Monte Carlo uncertainty. We investigate the role of this methodology in facilitating inference for computationally challenging dynamic latent variable models. We present examples arising in the study of infectious disease transmission, demonstrating our methodology for inference on nonlinear dynamic models using genetic sequence data and panel time-series data. We also discuss applicability to nonlinear time-series and spatio-temporal data.
更多查看译文
关键词
likelihood-based inference,sequential Monte Carlo,panel data,time series,spatio-temporal data,phylodynamic inference
AI 理解论文
溯源树
样例
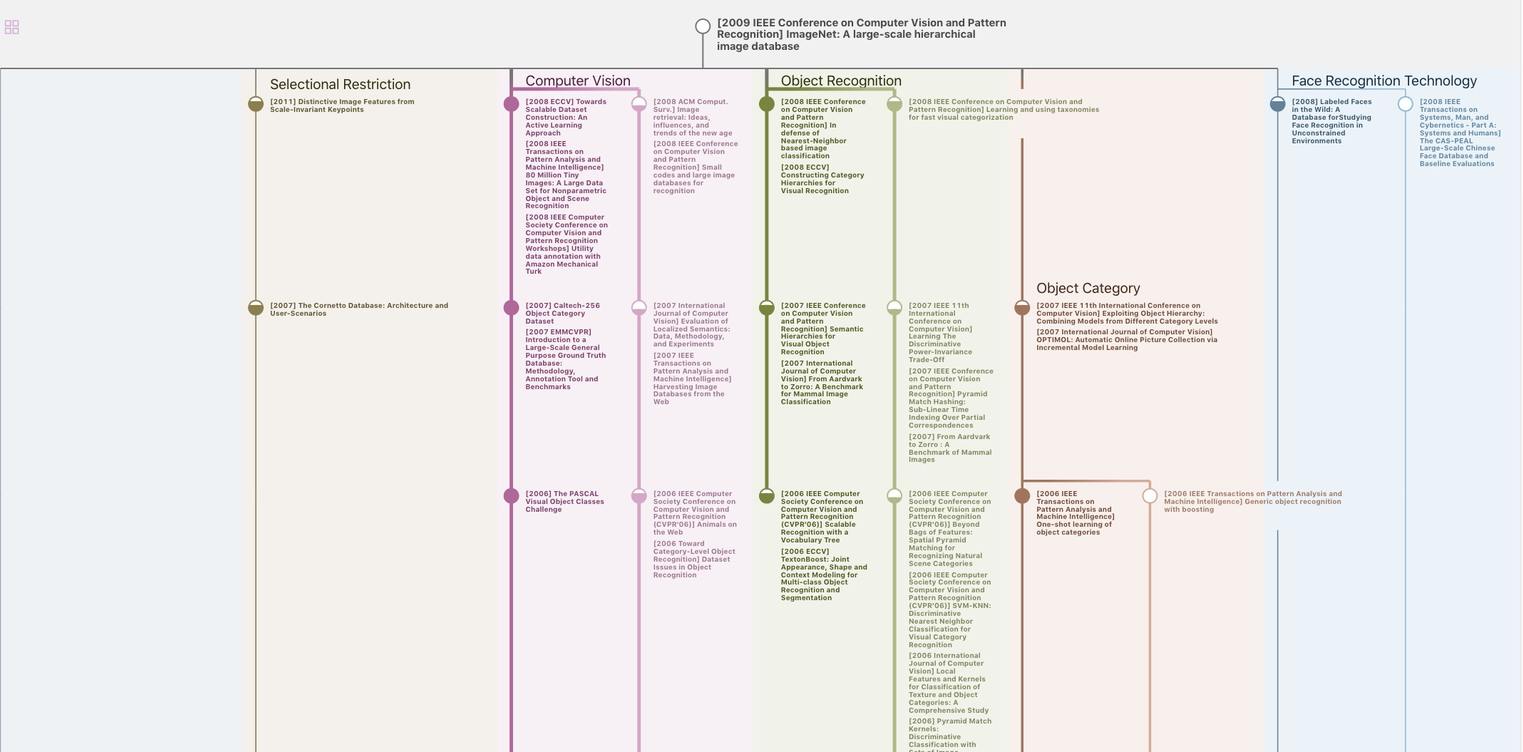
生成溯源树,研究论文发展脉络
Chat Paper
正在生成论文摘要