Feature extraction for robust physical activity recognition
Human-centric Computing and Information Sciences(2017)
摘要
This paper presents the development of a Human Activity Recognition (HAR) system that uses a network of nine inertial measurement units situated in different body parts. Every unit provides 3D (3-dimension) acceleration, 3D angular velocity, 3D magnetic field orientation, and 4D quaternions. This system identifies 33 different physical activities (walking, running, cycling, lateral elevation of arms, etc.). The system is composed of two main modules: a feature extractor for obtaining the most relevant characteristics from the inertial signals every second, and a machine learning algorithm for classifying between the different activities. This paper focuses on the feature extractor module, evaluating several types of features and proposing different normalization approaches. This paper also analyses the performance of every sensor included in the inertial measurement units. The main experiments have been done using a public available dataset named REALDISP Activity Recognition dataset. This dataset includes recordings from 17 subjects performing 33 different activities in three different scenarios. Final results demonstrate that the proposed HAR system significantly improves the classification accuracy compared to previous works on this dataset. For the best configuration, the system accuracy is 99.1%. This system has been also evaluated with the OPPORTUNITY dataset obtaining competitive results.
更多查看译文
关键词
Physical activity recognition,Human Activity Recognition,Feature extraction robustness,Type of sensor,Signal normalization,REALDISP Activity Recognition dataset,OPPORTUNITY dataset,Random Forest,Pattern recognition,Machine learning
AI 理解论文
溯源树
样例
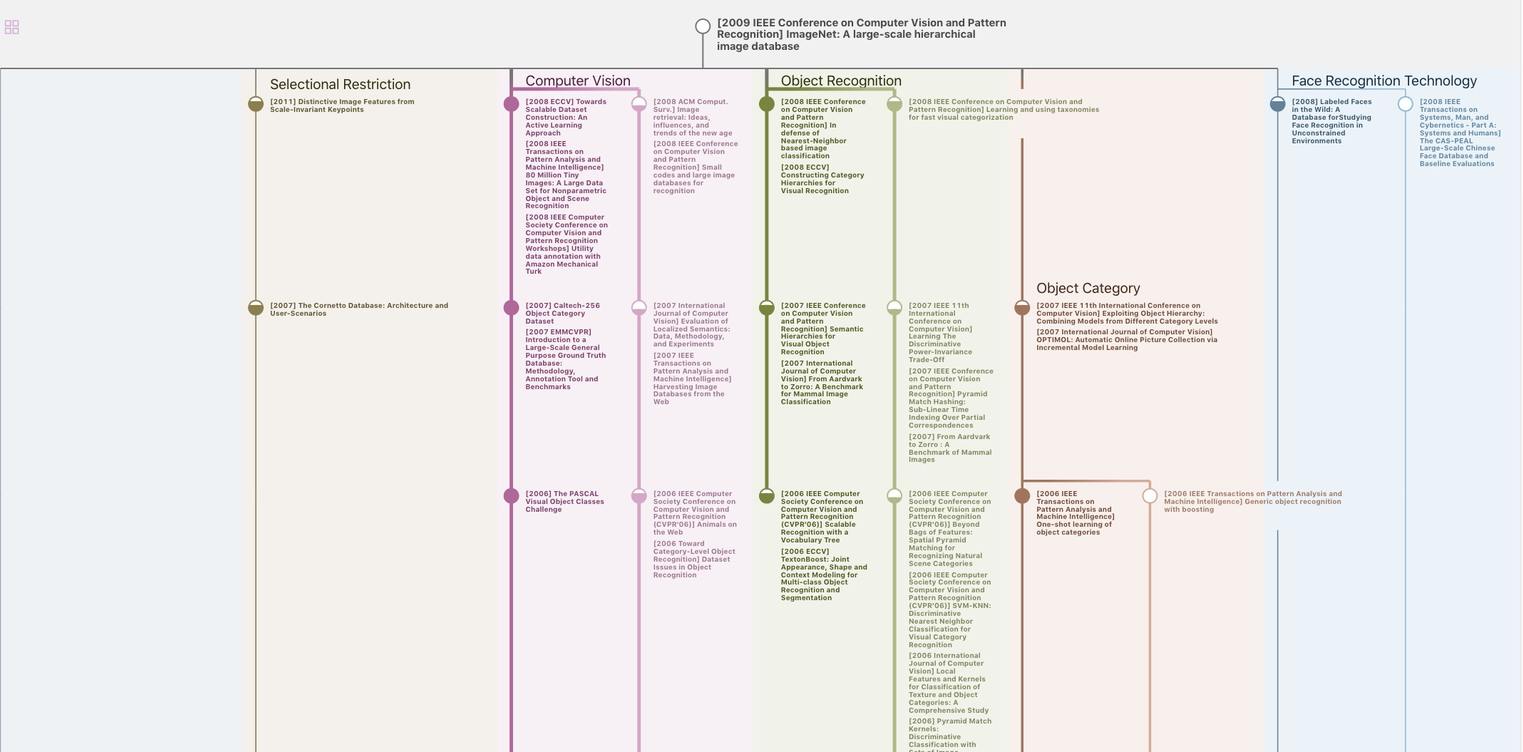
生成溯源树,研究论文发展脉络
Chat Paper
正在生成论文摘要