Accelerating Topic Exploration Of Multi-Dimensional Documents
2017 IEEE INTERNATIONAL PARALLEL AND DISTRIBUTED PROCESSING SYMPOSIUM WORKSHOPS (IPDPSW)(2017)
摘要
As multi-dimensional text data are being generated at dazzling rate, topic modelling has become an important instrument for learning from large unstructured document sets. To focus on specific subsets of large document corpora, a user may specify various criteria to identify documents of interest before extracting topics from the documents. In this paper, we aim to accelerate the computation of topic models for documents that satisfy range queries. Our strategy is to create indexes for identifying documents, and pre-compute the topic models for certain subsets of documents. The indexes enable to efficiently identify the exact set (called the Canonical Set) of documents that fall within the user-specified range. The target topic model is computed by combining the pre-computed models associated with the Canonical Set of documents. In contrast, the best known approach based on Octrees only allows identifying an estimated set of documents in relation to user's query. Because of the inexact identification of the document sets, the existing approach does not offer an effective error bound on the resulting model. Moreover, for a collection of n d-dimensional documents, the Octree approach requires O(n(1-1/d)) time in the worst case to search for the relevant documents, whereas our new approach guarantees to find the exact set in O(lg(d)n) time in the worst case. Because the new approach can identify the exact set of documents and the right pre-trained topic models to combine, it offers a much improved solution. The new scheme can also be parallelized with ease, including the building of the indexes, the pre-computing of the topic models, and the query processing.
更多查看译文
关键词
probabilistic topic model, social media, spatiotemporal documents, range queries, range trees, parallel processing for social media
AI 理解论文
溯源树
样例
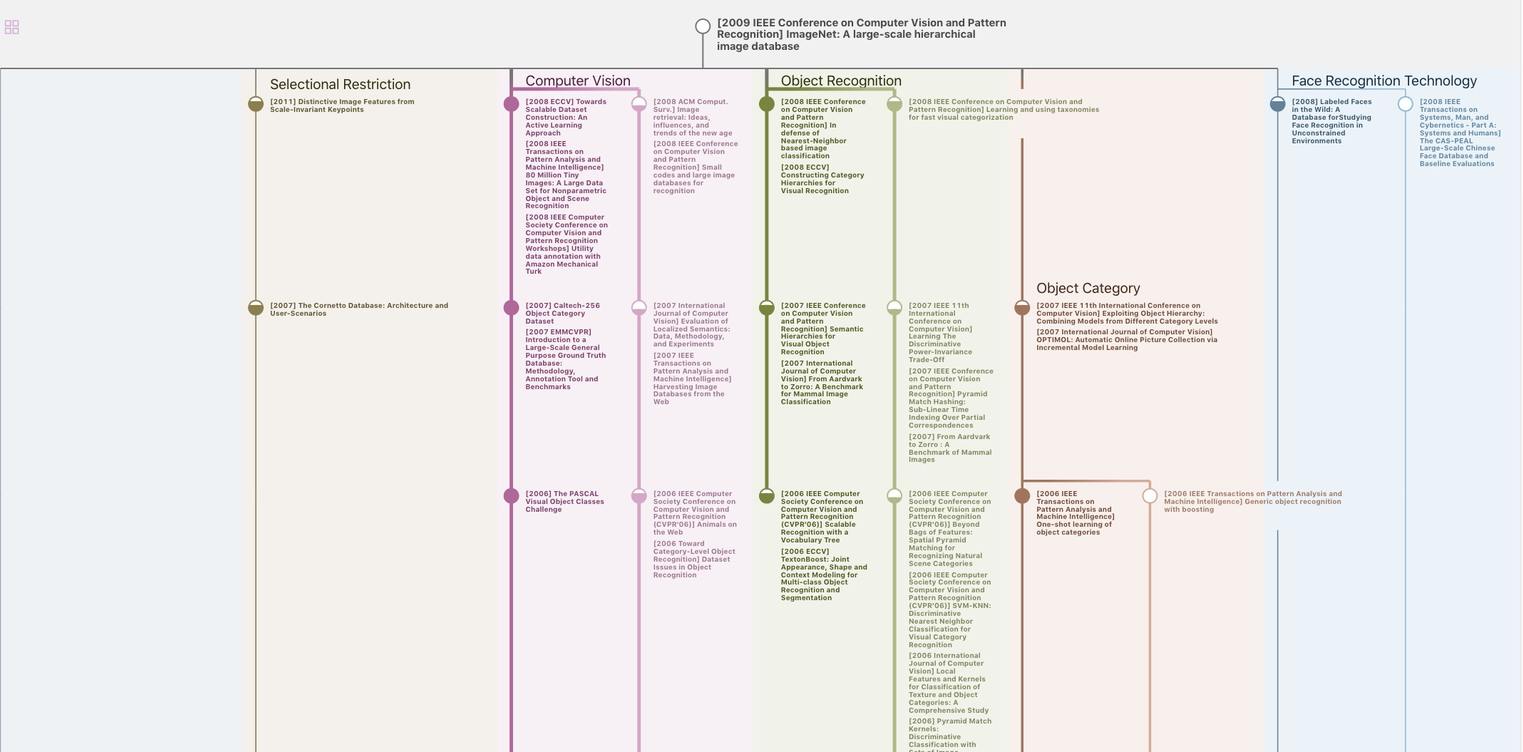
生成溯源树,研究论文发展脉络
Chat Paper
正在生成论文摘要