A New Approach Of Anomaly Detection In Wireless Sensor Networks Using Support Vector Data Description
INTERNATIONAL JOURNAL OF DISTRIBUTED SENSOR NETWORKS(2017)
摘要
Anomaly detection is an important challenge in wireless sensor networks for some applications, which require efficient, accurate, and timely data analysis to facilitate critical decision making and situation awareness. Support vector data description is well applied to anomaly detection using a very attractive kernel method. However, it has a high computational complexity since the standard version of support vector data description needs to solve quadratic programming problem. In this article, an improved method on the basis of support vector data description is proposed, which reduces the computational complexity and is used for anomaly detection in energy-constraint wireless sensor networks. The main idea is to improve the computational complexity from the training stage and the decision-making stage. First, the strategy of training sample reduction is used to cut back the number of samples and then the sequential minimal optimization algorithm based on the second-order approximation is implemented on the sample set to achieve the goal of reducing the training time. Second, through the analysis of the decision function, the pre-image in the original space corresponding to the center of hyper-sphere in kernel feature space can be obtained. The decision complexity is reduced from O(l) to O(1) using the pre-image. Eventually, the experimental results on several benchmark datasets and real wireless sensor networks datasets demonstrate that the proposed method can not only guarantee detection accuracy but also reduce time complexity.
更多查看译文
关键词
Wireless sensor networks, support vector data description, anomaly detection, sequential minimal optimization, pre-image
AI 理解论文
溯源树
样例
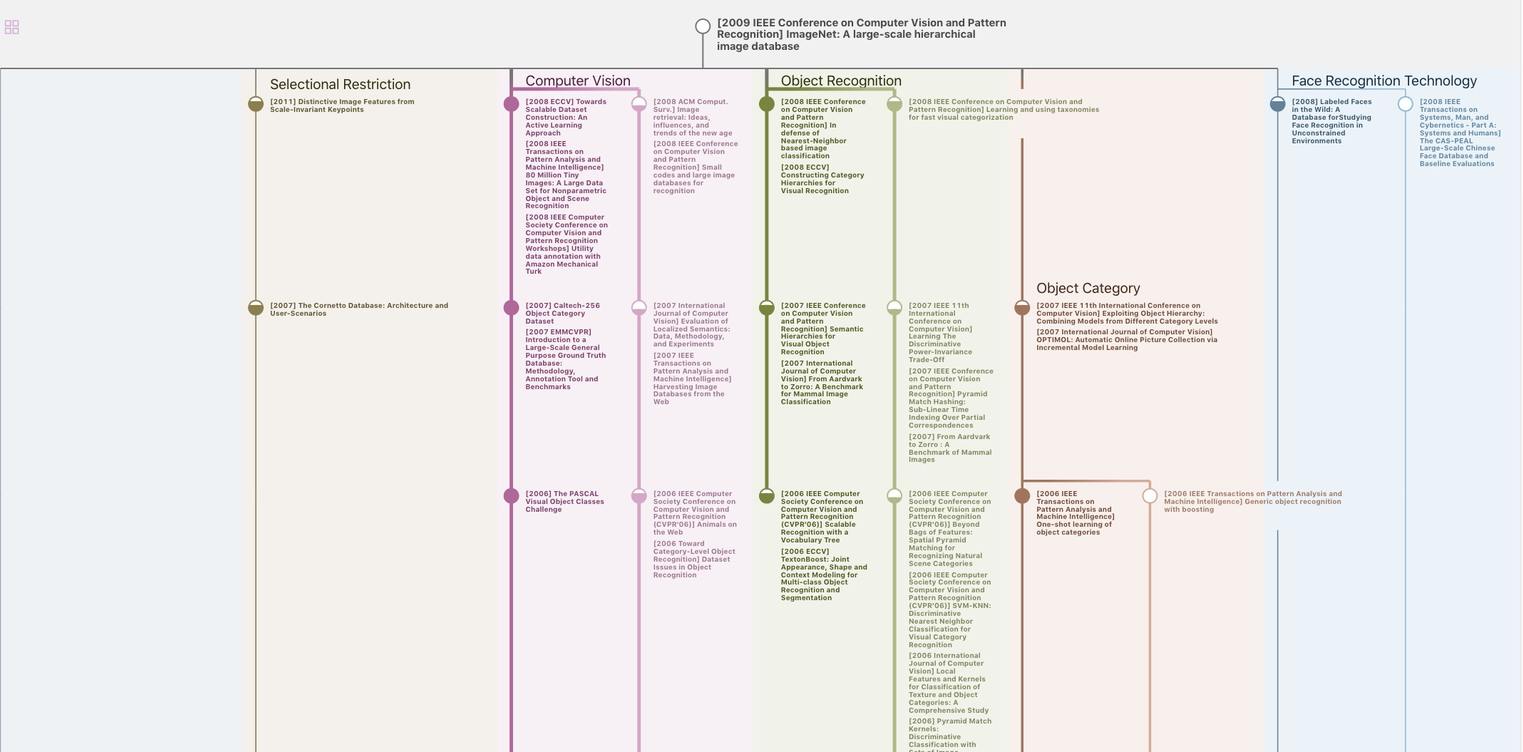
生成溯源树,研究论文发展脉络
Chat Paper
正在生成论文摘要