Computational Fact Checking through Query Perturbations.
ACM Trans. Database Syst.(2017)
摘要
Our media is saturated with claims of “facts” made from data. Database research has in the past focused on how to answer queries, but has not devoted much attention to discerning more subtle qualities of the resulting claims, for example, is a claim “cherry-picking”? This article proposes a framework that models claims based on structured data as parameterized queries. Intuitively, with its choice of the parameter setting, a claim presents a particular (and potentially biased) view of the underlying data. A key insight is that we can learn a lot about a claim by “perturbing” its parameters and seeing how its conclusion changes. For example, a claim is not robust if small perturbations to its parameters can change its conclusions significantly. This framework allows us to formulate practical fact-checking tasks—reverse-engineering vague claims, and countering questionable claims—as computational problems. Along with the modeling framework, we develop an algorithmic framework that enables efficient instantiations of “meta” algorithms by supplying appropriate algorithmic building blocks. We present real-world examples and experiments that demonstrate the power of our model, efficiency of our algorithms, and usefulness of their results.
更多查看译文
关键词
Sensitivity analysis,fact checking,computational journalism
AI 理解论文
溯源树
样例
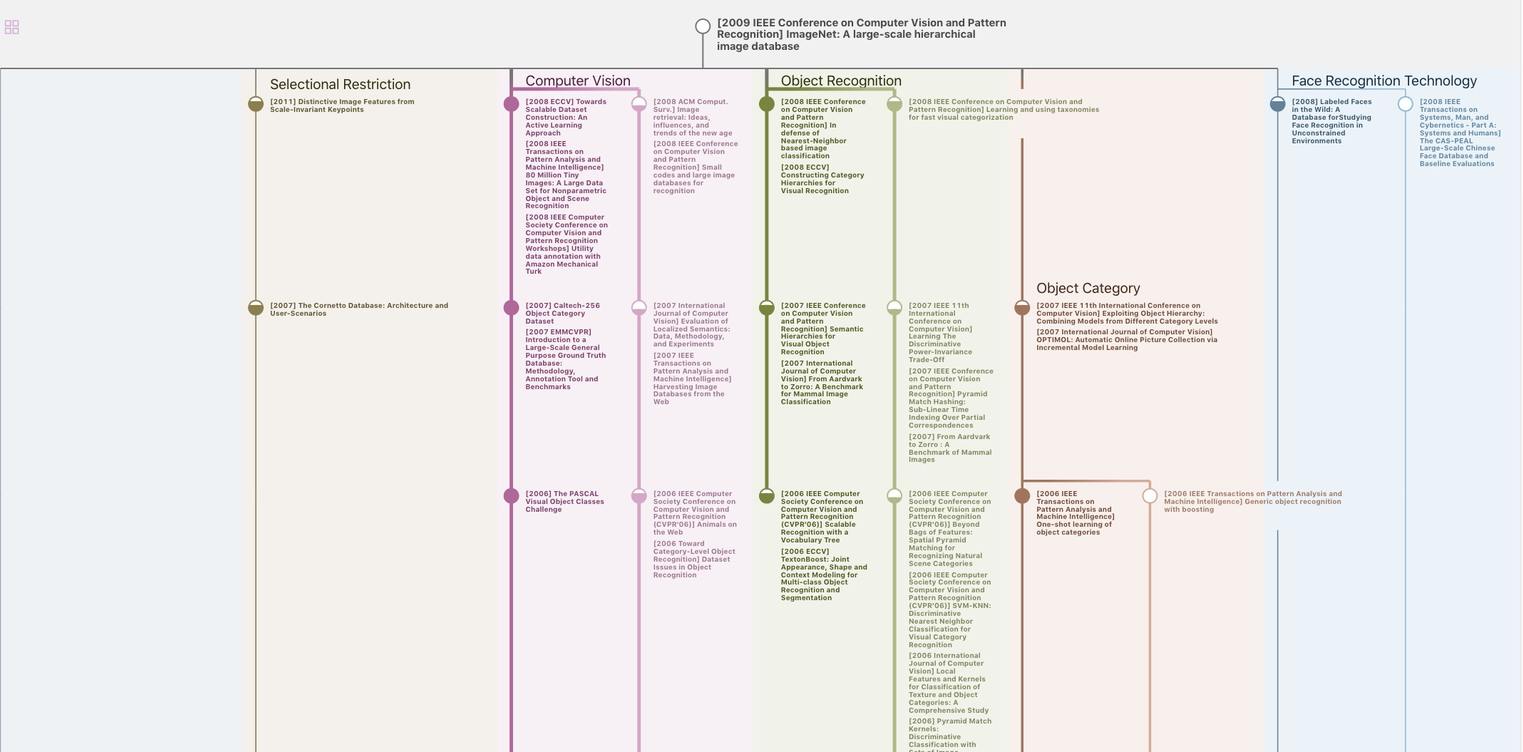
生成溯源树,研究论文发展脉络
Chat Paper
正在生成论文摘要