Service Recommendation Based on Separated Time-aware Collaborative Poisson Factorization.
JOURNAL OF WEB ENGINEERING(2017)
摘要
With the booming of web service ecosystems, finding suitable services and making service compositions have become an principal challenge for inexperienced developers. Therefore, recommending services based on service composition queries turns out to be a promising solution. Many recent studies apply Latent Dirichlet Allocation (LDA) to model the queries and services' description. However, limited by the restrictive assumption of the Dirichlet-Multinomial distribution assumption, LDA cannot generate highquality latent presentation, thus the accuracy of recommendation isn't quite satisfactory. Based on our previous work, we propose a Separated Time-aware Collaborative Poisson Factorization (STCPF) to tackle the problem in this paper. STCPF takes Poisson Factorization as the foundation to model mashup queries and service descriptions separately, and incorporates them with the historical usage data together by using collective matrix factorization. Experiments on the real-world show that our model outperforms than the state-of-the-art methods (e.g., Time-aware collaborative domain regression) in terms of mean average precision, and costs much less time on the sparse but massive data from web service ecosystem.
更多查看译文
关键词
service recommendation,service composition,Time-aware,Poisson Factorization
AI 理解论文
溯源树
样例
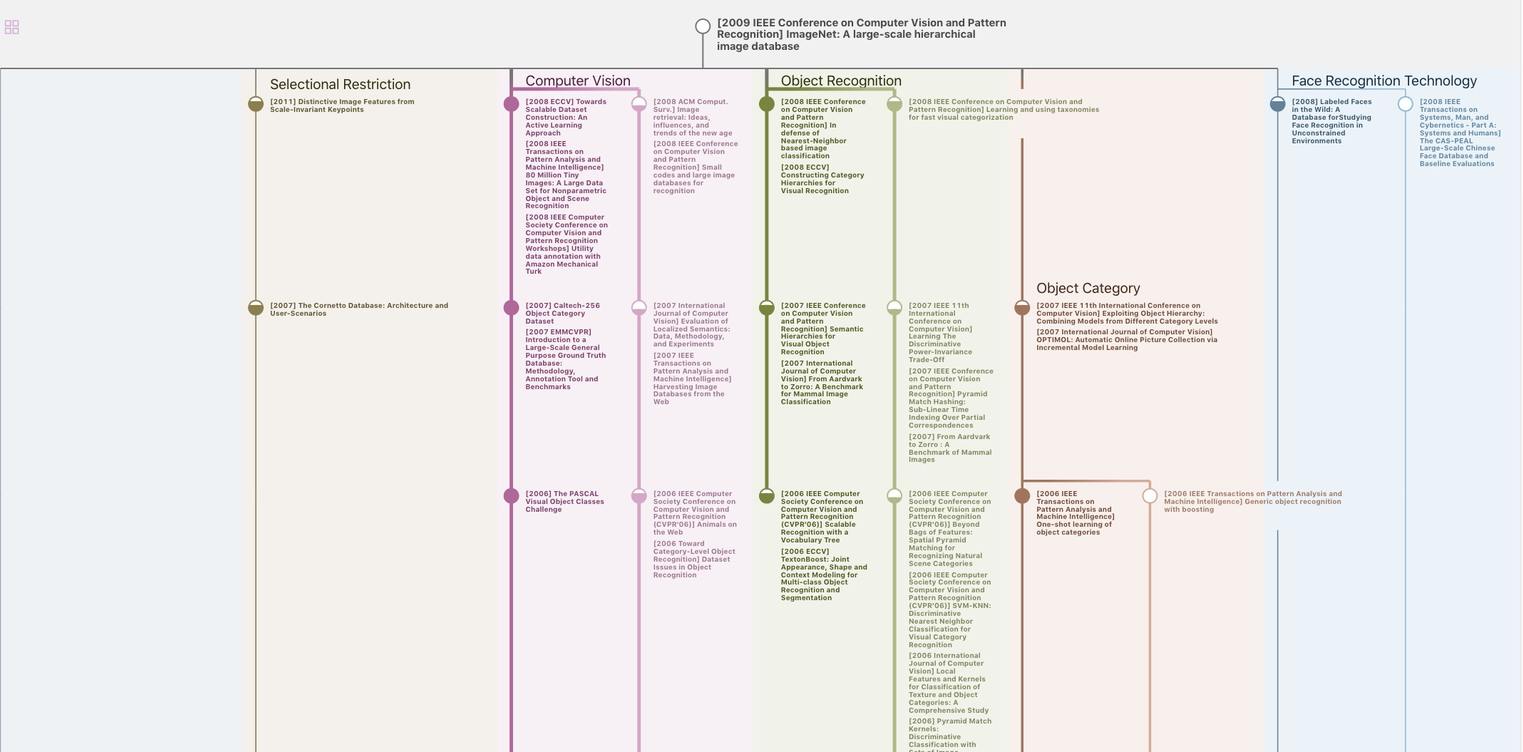
生成溯源树,研究论文发展脉络
Chat Paper
正在生成论文摘要