The value of vehicle telematics data in insurance risk selection processes.
Decision Support Systems(2017)
摘要
The advent of the Internet of Things enables companies to collect an increasing amount of sensor generated data which creates plenty of new business opportunities. This study investigates how this sensor data can improve the risk selection process in an insurance company. More specifically, several risk assessment models based on three different data mining techniques are augmented with driving behaviour data collected from In-Vehicle Data Recorders. This study proves that including standard telematics variables significantly improves the risk assessment of customers. As a result, insurers will be better able to tailor their products to the customers' risk profile. Moreover, this research illustrates the importance of including industry knowledge, combined with data expertise, in the variable creation process. Especially when a regulator forces the use of easily interpretable data mining techniques, expert-based telematics variables are able to improve the risk assessment model in addition to the standard telematics variables. Further, the results suggest that if a manager wants to implement Usage-Based-Insurances, Pay-As-You-Drive related variables are most valuable to tailor the premium to the risk. Finally, the study illustrates that this new type of telematics-based insurance product can quickly be implemented since three months of data is already sufficient to obtain the best risk estimations. This study proves the value of telematics-based data in the risk selection process of an insurance companyIt compares the performance of three models in this context: a logistic regression, random forests and artificial neural networks modelThis research illustrates the importance of industry knowledge in the variable creation processThree months of data is sufficient to obtain the best risk estimations
更多查看译文
关键词
Internet of things,Usage-based-insurance,Risk assessment model,Logistic regression,Random forests,Artificial neural networks
AI 理解论文
溯源树
样例
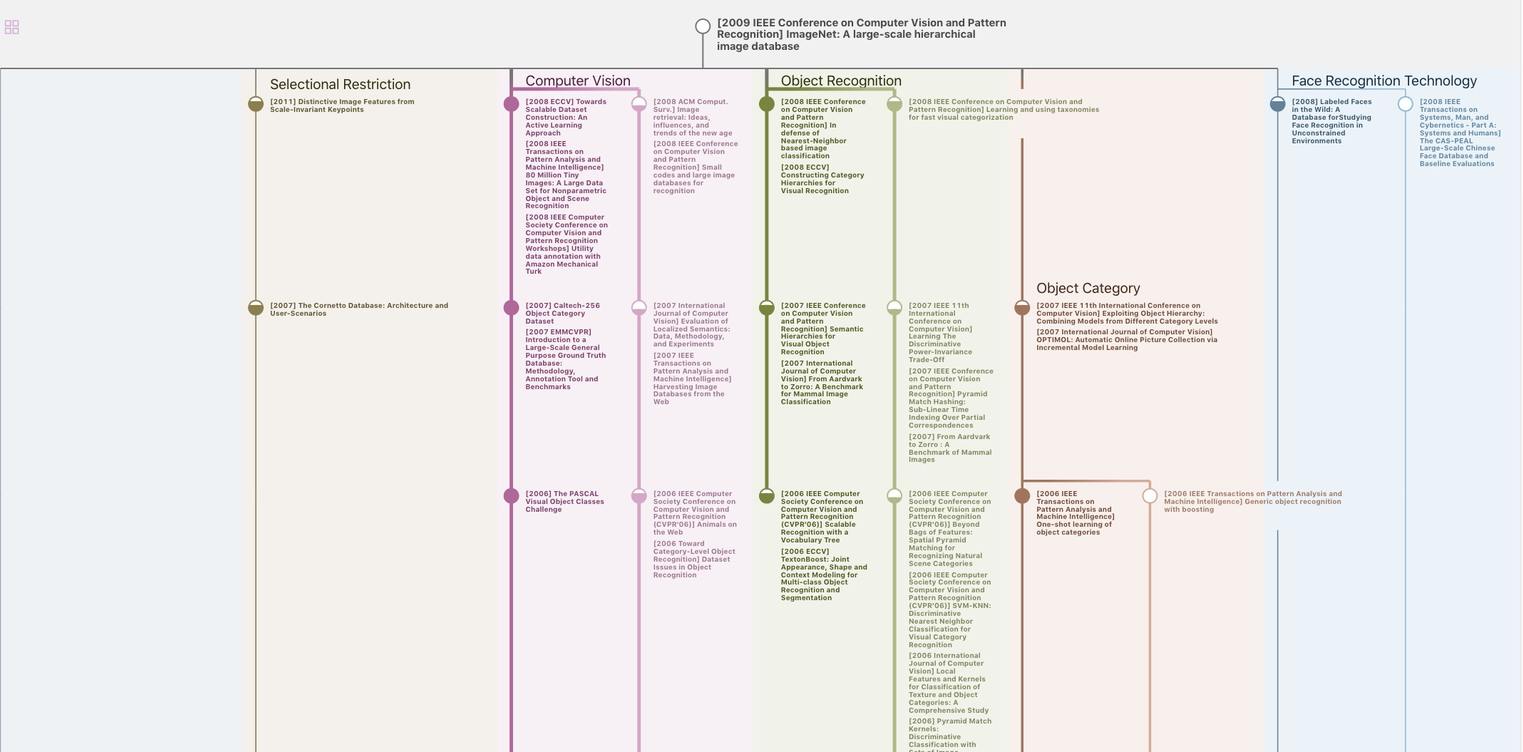
生成溯源树,研究论文发展脉络
Chat Paper
正在生成论文摘要