Distributed Parallel Particle Swarm Optimization for Multi-Objective and Many-Objective Large-Scale Optimization.
IEEE ACCESS(2017)
摘要
With the advent of big data era, complex optimization problems with many objectives and large numbers of decision variables are constantly emerging. Traditional research about multi-objective particle swarm optimization (PSO) focuses on multi-objective optimization problems (MOPs) with small numbers of variables and less than four objectives. At present, MOPs with large numbers of variables and many objectives (greater than or equal to four) are constantly emerging. When tackling this type of MOPs, the traditional multi-objective PSO algorithms have low efficiency. Aiming at these multi-objective large-scale optimization problems (MOLSOPs) and many-objective large-scale optimization problems (MaOLSOPs), we need to explore thoroughly parallel attributes of the particle swarm, and design the novel PSO algorithms according to the characteristics of distributed parallel computation. We survey the related research on PSO: multi-objective large-scale optimization, many-objective optimization, and distributed parallelism. Based on the aforementioned three aspects, the multi-objective large-scale distributed parallel PSO and many-objective large-scale distributed parallel PSO methodologies are proposed and discussed, and the other future research trends are also illuminated.
更多查看译文
关键词
Particle swarm optimization (PSO),multi-objective optimization,many-objective optimization,large-scale optimization,distributed parallelism
AI 理解论文
溯源树
样例
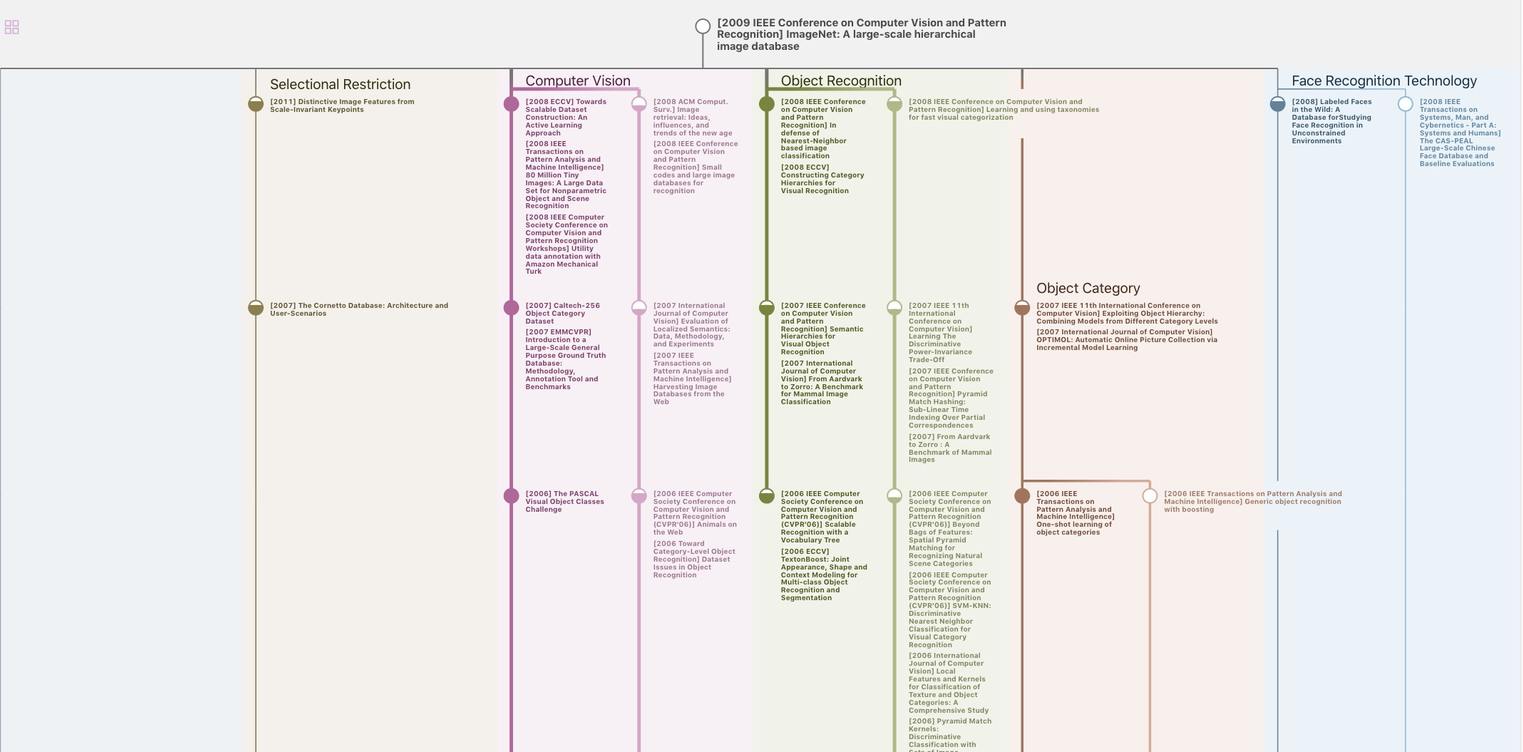
生成溯源树,研究论文发展脉络
Chat Paper
正在生成论文摘要