Underdetermined Joint Blind Source Separation of Multiple Datasets.
IEEE ACCESS(2017)
摘要
In this paper, we tackle the problem of jointly separating instantaneous linear underdetermined mixtures of latent sources from multiple data sets, where the number of sources exceeds that of observations in each data set. Currently available blind source separation (BSS) methods, including joint BSS (JBSS) and underdetermined BSS (UBSS), cannot address this underdetermined problem effectively. We exploit the second-order statistics of observations, and present a novel BSS method, referred to as underdetermined joint BSS for multiple data sets (UJBSS-m), as a generalization of our previous work on two data sets. In this paper, the cross correlation between each pair of data sets is modeled by a third-order tensor in which a set of spatial covariance matrices corresponding to different time delays are stacked. Considering the latent common structure of these constructed tensors, the mixing matrices are jointly estimated via joint canonical polyadic decomposition of these specialized tensors. Furthermore, we recover the sources from each data set separately based on the estimated mixing matrices. Simulation results demonstrate that the proposed UJBSS-m method yields superior performances when compared with commonly used single-set UBSS and JBSS methods.
更多查看译文
关键词
Underdetermined joint blind source separation,joint canonical polyadic decomposition,cross correlation
AI 理解论文
溯源树
样例
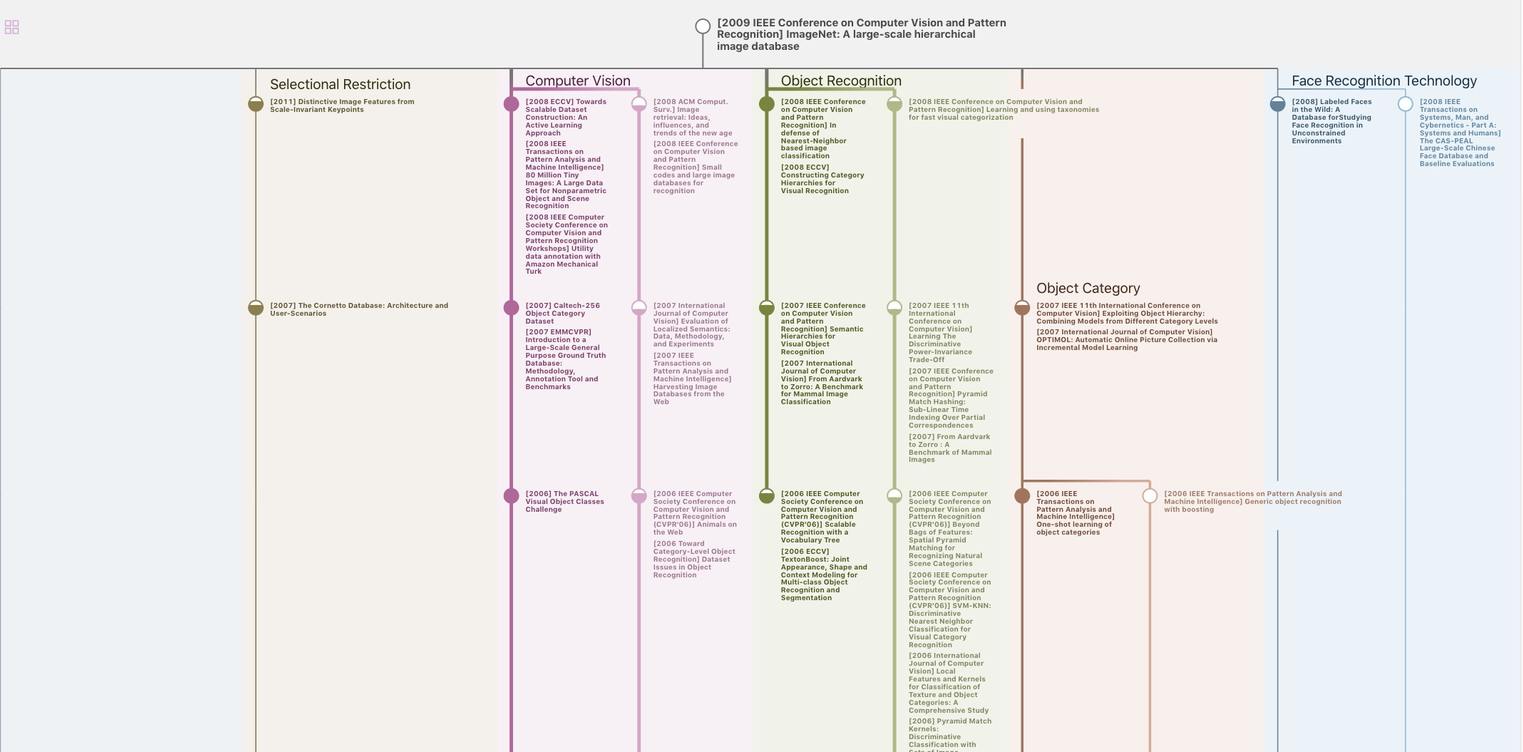
生成溯源树,研究论文发展脉络
Chat Paper
正在生成论文摘要