Named entity disambiguation for questions in community question answering.
Knowl.-Based Syst.(2017)
摘要
Named entity disambiguation (NED) refers to the task of mapping entity mentions in running texts to the correct entries in a specific knowledge base (e.g., Wikipedia). Although there has been a lot of work on NED for long and formal texts like Wikipedia and news, the task is not well studied for questions in community question answering (CQA). The challenges of the task include little context for mentions in questions, lack of ground truth for learning, and language gaps between CQA and knowledge bases. To overcome these problems, we propose a topic modelling approach to NED for questions. Our model performs learning in an unsupervised manner, but can take advantage of weak supervision signals estimated from the metadata of CQA and knowledge bases. The signals can enrich the context of mentions in questions, and bridge the language gaps between CQA and knowledge bases. Besides these advantages, our model simulates peoples behavior in CQA and thus is intuitively interpretable. We conduct experiments on both Chinese and English CQA data. The experimental results show that our method can significantly outperform state-of-the-art methods when we apply them to questions in CQA.
更多查看译文
关键词
Named entity disambiguation,Topic model,Unsupervised learning,Community question answering
AI 理解论文
溯源树
样例
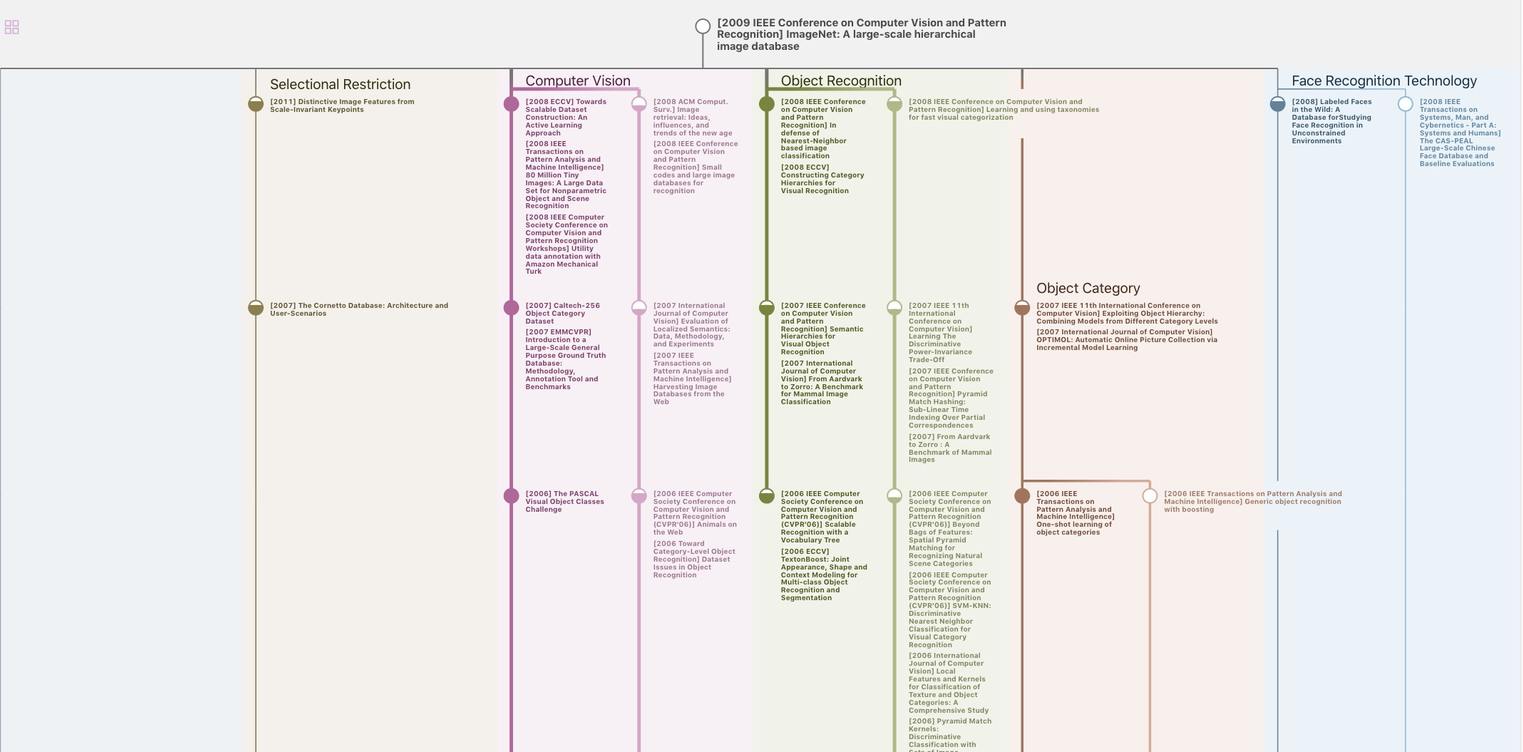
生成溯源树,研究论文发展脉络
Chat Paper
正在生成论文摘要