A ranking-based strategy to prune variable selection ensembles.
Knowl.-Based Syst.(2017)
摘要
Ensemble pruning techniques are introduced in the context of variable selection.A ranking-based strategy is devised to prune a variable selection ensemble.The ensemble members are sorted by the prediction error of their associated models.Higher selection accuracy is gained by fusing fewer members ranked ahead.The superiority of the novel method over some other methods is validated. Ensemble learning has attracted significant interest in the literature of variable selection due to its great potential to reduce false discovery rate and to stabilize selection results. In this paper, a novel ensemble pruning technique called PST2E (i.e., pruned ST2E) is introduced to obtain smaller but stronger variable selection ensembles. For the ensemble members generated by the ST2E algorithm [3], we sort them in descending order according to the prediction errors associated with their determined models. Subsequently, only a desired number of members ranked ahead are integrated to compose a subensemble. On the basis of the average importance measures produced by the pruned ensemble, all candidate variables are then ranked and decided to be important or not. In the context of linear and logistic regression models, the experiments conducted with both simulated and real-world data illustrate that PST2E significantly outperforms several other popular techniques in most cases when evaluating them with multiple measures. Another advantage of PST2E is that it admits easy implementation. As a result, PST2E can be deemed as an attractive alternative to tackle variable selection tasks in real applications.
更多查看译文
关键词
Variable selection ensemble,Ensemble pruning,Selection accuracy,Aggregation order,Variable ranking,Stochastic stepwise selection
AI 理解论文
溯源树
样例
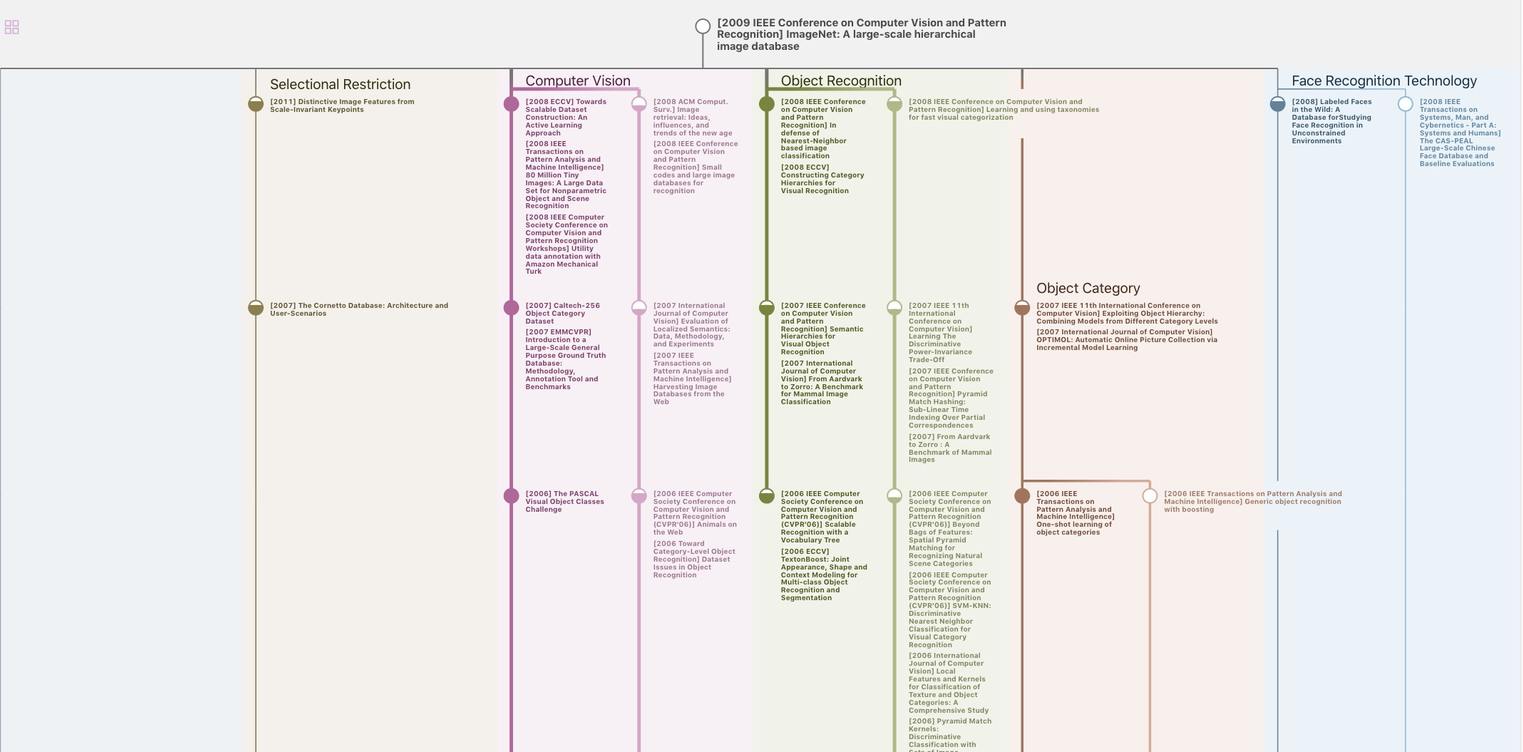
生成溯源树,研究论文发展脉络
Chat Paper
正在生成论文摘要