A coordinate descent algorithm for computing penalized smooth quantile regression
Statistics and Computing(2016)
摘要
The computation of penalized quantile regression estimates is often computationally intensive in high dimensions. In this paper we propose a coordinate descent algorithm for computing the penalized smooth quantile regression (cdaSQR) with convex and nonconvex penalties. The cdaSQR approach is based on the approximation of the objective check function, which is not differentiable at zero, by a modified check function which is differentiable at zero. Then, using the maximization-minimization trick of the gcdnet algorithm (Yang and Zou in, J Comput Graph Stat 22(2):396–415, 2013 ), we update each coefficient simply and efficiently. In our implementation, we consider the convex penalties ℓ _1+ℓ _2 and the nonconvex penalties SCAD (or MCP) + ℓ _2 . We establishe the convergence property of the csdSQR with ℓ _1+ℓ _2 penalty. The numerical results show that our implementation is an order of magnitude faster than its competitors. Using simulations we compare the speed of our algorithm to its competitors. Finally, the performance of our algorithm is illustrated on three real data sets from diabetes, leukemia and Bardet–Bidel syndrome gene expression studies.
更多查看译文
关键词
Variable selection,Quantile regression,Smooth check function,Coordinate descent algorithm
AI 理解论文
溯源树
样例
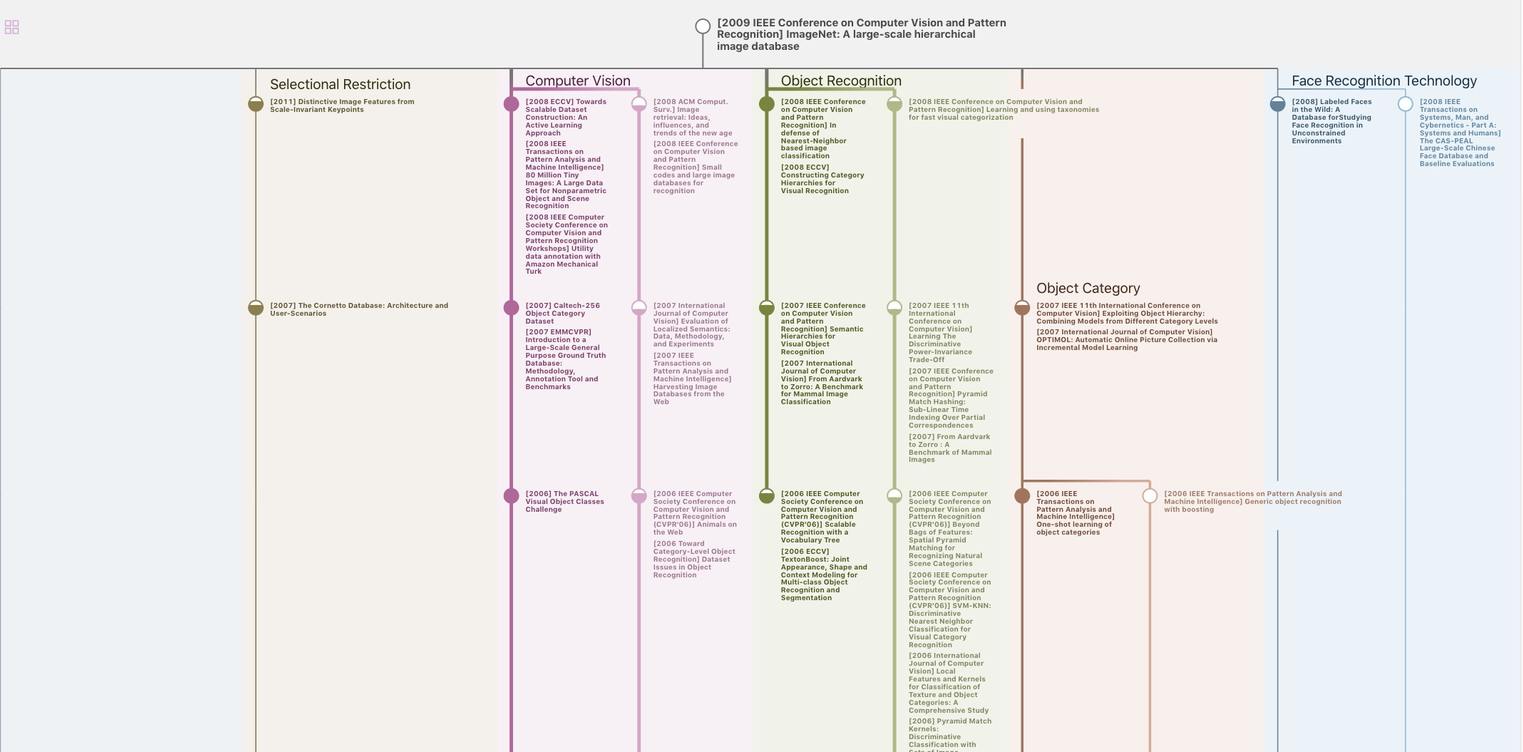
生成溯源树,研究论文发展脉络
Chat Paper
正在生成论文摘要