Hierarchical feature concatenation-based kernel sparse representations for image categorization
The Visual Computer(2016)
摘要
In order to obtain improved performance in complicated visual categorization tasks, considerable research has adopted multiple kernel learning based on dozens of different features. However, it is a complex process that needs to extract a multitude of features and seeks the optimal combination of multiple kernels. Inspired by the key idea of hierarchical learning, in this paper, we propose to find sparse representation based on feature concatenation using hierarchical kernel orthogonal matching pursuit (HKOMP). In addition to commonly used spatial pyramid feature for kernel representation, our method only employs one type of generic image feature, i.e., p.d.f gradient-based orientation histogram for concatenation of sparse codes. Next, the resulting concatenated features kernelized with widely used Gaussian radial basis kernel function form compact sparse representations in the second layer for linear support vector machine. HKOMP algorithm combines the advantages of building image representations layer-by-layer and kernel learning. Several publicly available image datasets are used to evaluate the presented approach and empirical results for various datasets show that the proposed scheme outperforms many kernel learning based and other competitive image categorization algorithms.
更多查看译文
关键词
Image categorization,Kernel sparse representation,Feature concatenation,Hierarchical learning,Linear support vector machine
AI 理解论文
溯源树
样例
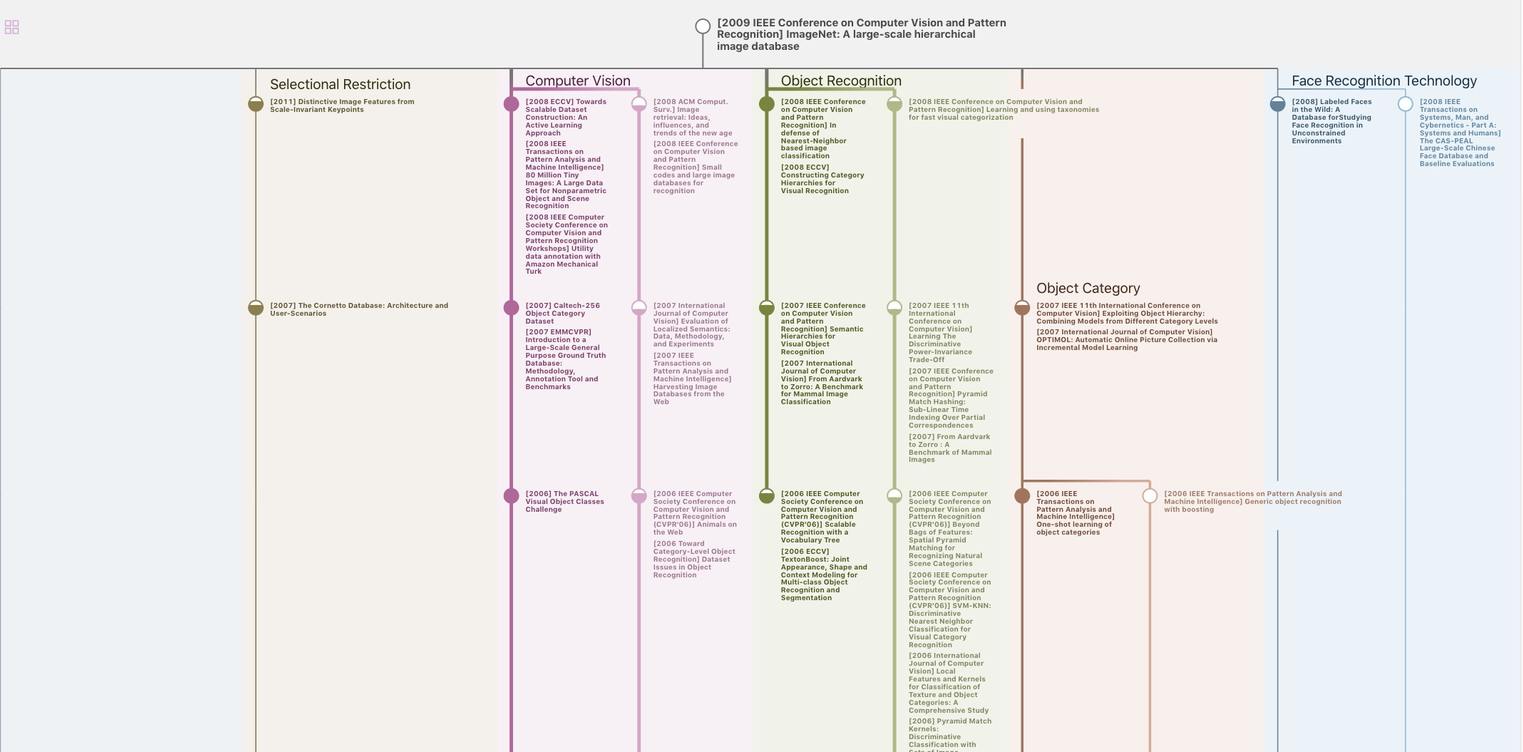
生成溯源树,研究论文发展脉络
Chat Paper
正在生成论文摘要