Scalable modeling and solution of stochastic multiobjective optimization problems.
Computers & Chemical Engineering(2017)
摘要
Abstract We present a scalable computing framework for the solution stochastic multiobjective optimization problems. The proposed framework uses a nested conditional value-at-risk (nCVaR) metric to find compromise solutions among conflicting random objectives. We prove that the associated nCVaR minimization problem can be cast as a standard stochastic programming problem with expected value (linking) constraints. We also show that these problems can be implemented in a modular and compact manner using PLASMO (a Julia -based structured modeling framework) and can be solved efficiently using PIPS-NLP (a parallel nonlinear solver). We apply the framework to a CHP design study in which we seek to find compromise solutions that trade-off cost, water, and emissions in the face of uncertainty in electricity and water demands.
更多查看译文
关键词
Large scale,Optimization,Stochastic,Multiobjective,CVaR
AI 理解论文
溯源树
样例
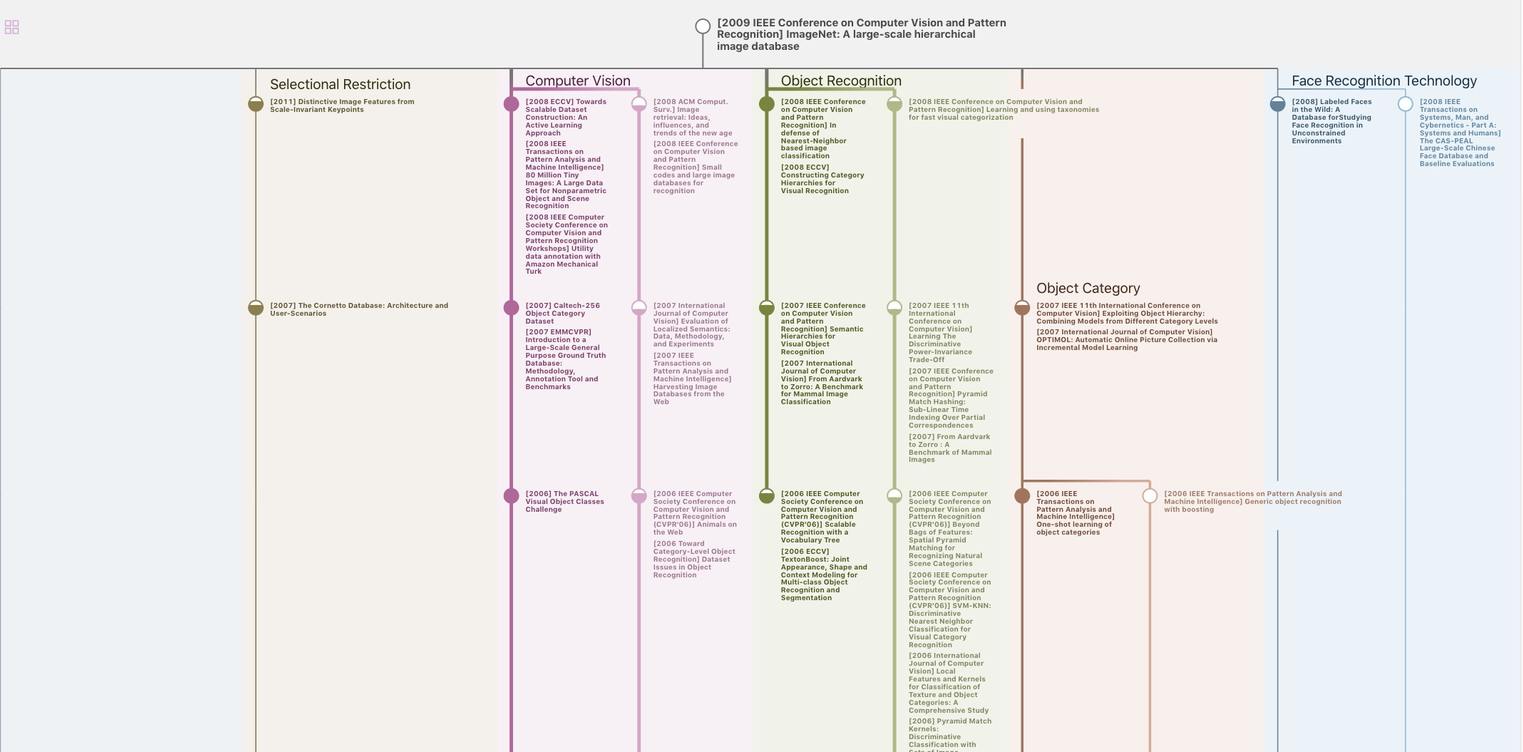
生成溯源树,研究论文发展脉络
Chat Paper
正在生成论文摘要