Neural Ctrl-F: Segmentation-Free Query-By-Stringword Spotting in Handwritten Manuscript Collections
IEEE International Conference on Computer Vision(2017)
摘要
In this paper, we approach the problem of segmentation-free query-by-string word spotting for handwritten documents. In other words, we use methods inspired from computer vision and machine learning to search for words in large collections of digitized manuscripts. In particular, we are interested in historical handwritten texts, which are often far more challenging than modern printed documents. This task is important, as it provides people with a way to quickly find what they are looking for in large collections that are tedious and difficult to read manually. To this end, we introduce an end-to-end trainable model based on deep neural networks that we call Ctrl-F-Net. Given a full manuscript page, the model simultaneously generates region proposals, and embeds these into a distributed word embedding space, where searches are performed. We evaluate the model on common benchmarks for handwritten word spotting, outperforming the previous state-of-the-art segmentation-free approaches by a large margin, and in some cases even segmentation-based approaches. One interesting real-life application of our approach is to help historians to find and count specific words in court records that are related to women's sustenance activities and division of labor. We provide promising preliminary experiments that validate our method on this task.
更多查看译文
关键词
handwritten word spotting,distributed word embedding space,manuscript page,Ctrl-F-Net,deep neural networks,end-to-end trainable model,historical handwritten texts,digitized manuscripts,machine learning,computer vision,handwritten documents,handwritten manuscript collections,segmentation-free query-by-string word spotting,neural Ctrl-F
AI 理解论文
溯源树
样例
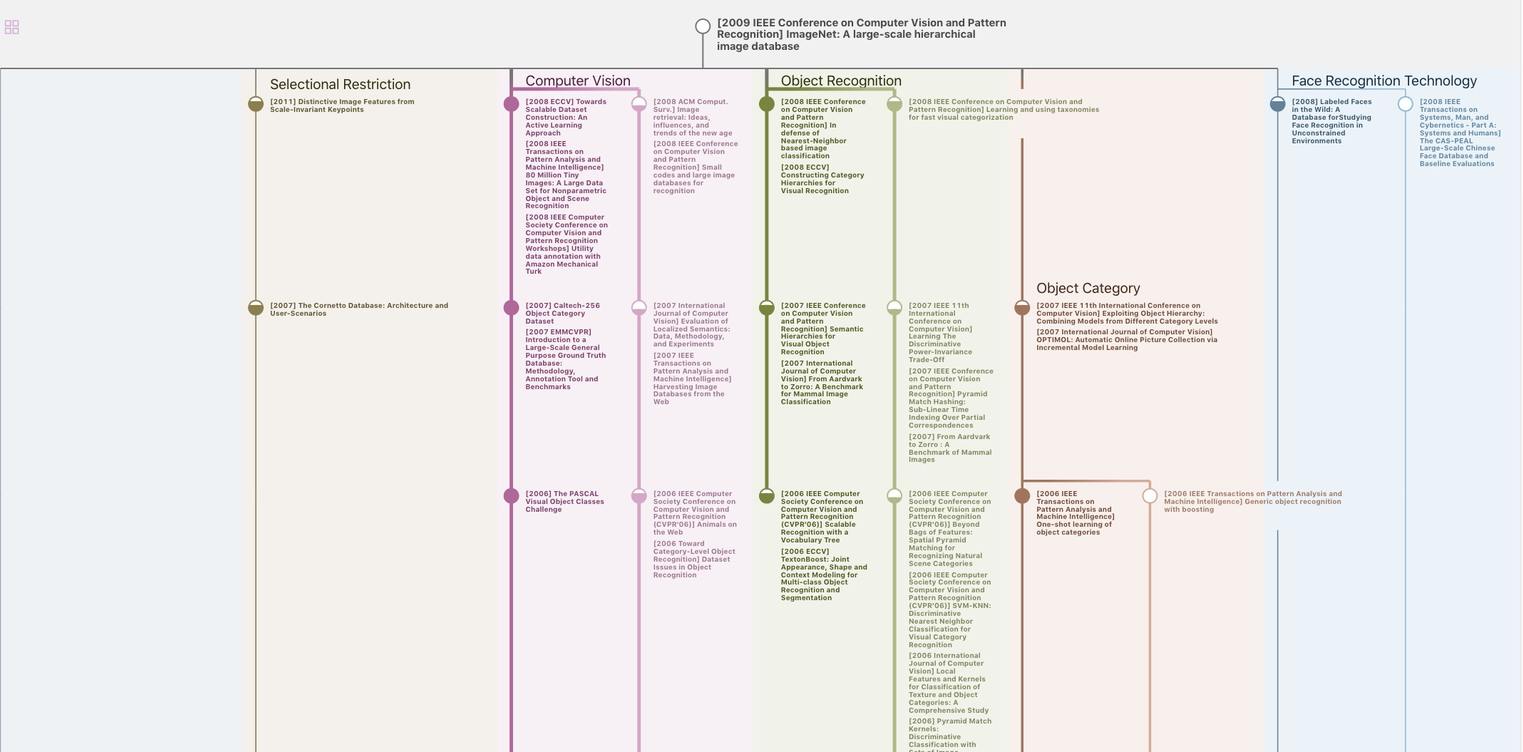
生成溯源树,研究论文发展脉络
Chat Paper
正在生成论文摘要