Learning to Remember Rare Events.
ICLR(2017)
摘要
Despite recent advances, memory-augmented deep neural networks are still limitedwhen it comes to life-long and one-shot learning, especially in remembering rare events.We present a large-scale life-long memory module for use in deep learning.The module exploits fast nearest-neighbor algorithms for efficiency andthus scales to large memory sizes.Except for the nearest-neighbor query, the module is fully differentiableand trained end-to-end with no extra supervision. It operates ina life-long manner, i.e., without the need to reset it during training.Our memory module can be easily added to any part of a supervised neural network.To show its versatility we add it to a number of networks, from simpleconvolutional ones tested on image classification to deep sequence-to-sequenceand recurrent-convolutional models.In all cases, the enhanced network gains the ability to rememberand do life-long one-shot learning.Our module remembers training examples shown many thousandsof steps in the past and it can successfully generalize from them.We set new state-of-the-art for one-shot learning on the Omniglot datasetand demonstrate, for the first time, life-long one-shot learning inrecurrent neural networks on a large-scale machine translation task.
更多查看译文
AI 理解论文
溯源树
样例
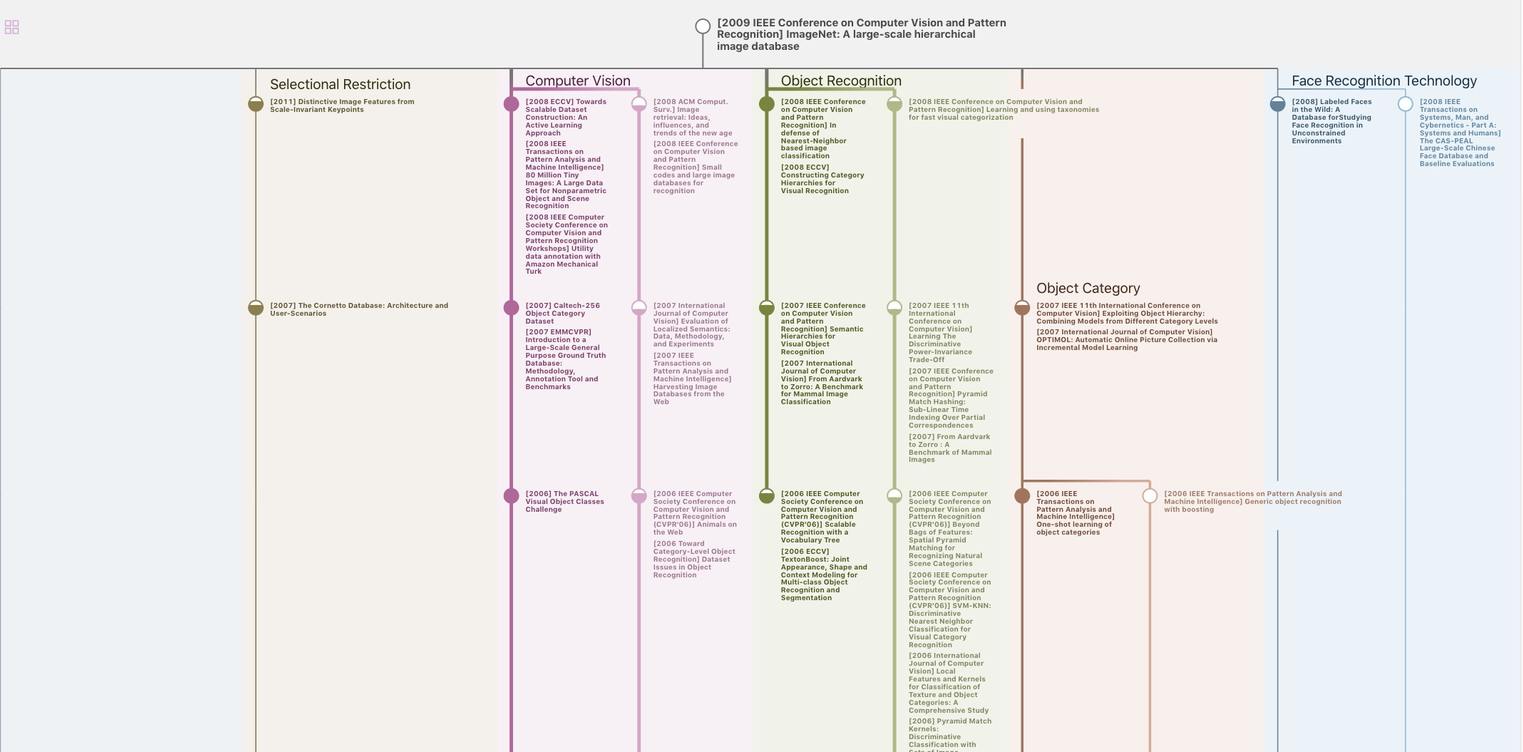
生成溯源树,研究论文发展脉络
Chat Paper
正在生成论文摘要