Deep relaxation: partial differential equations for optimizing deep neural networks
Research in the Mathematical Sciences(2018)
摘要
Entropy-SGD is a first-order optimization method which has been used successfully to train deep neural networks. This algorithm, which was motivated by statistical physics, is now interpreted as gradient descent on a modified loss function. The modified, or relaxed, loss function is the solution of a viscous Hamilton–Jacobi partial differential equation (PDE). Experimental results on modern, high-dimensional neural networks demonstrate that the algorithm converges faster than the benchmark stochastic gradient descent (SGD). Well-established PDE regularity results allow us to analyze the geometry of the relaxed energy landscape, confirming empirical evidence. Stochastic homogenization theory allows us to better understand the convergence of the algorithm. A stochastic control interpretation is used to prove that a modified algorithm converges faster than SGD in expectation.
更多查看译文
关键词
Deep learning,Partial differential equations,Stochastic gradient descent,Neural networks,Optimal control,Proximal
AI 理解论文
溯源树
样例
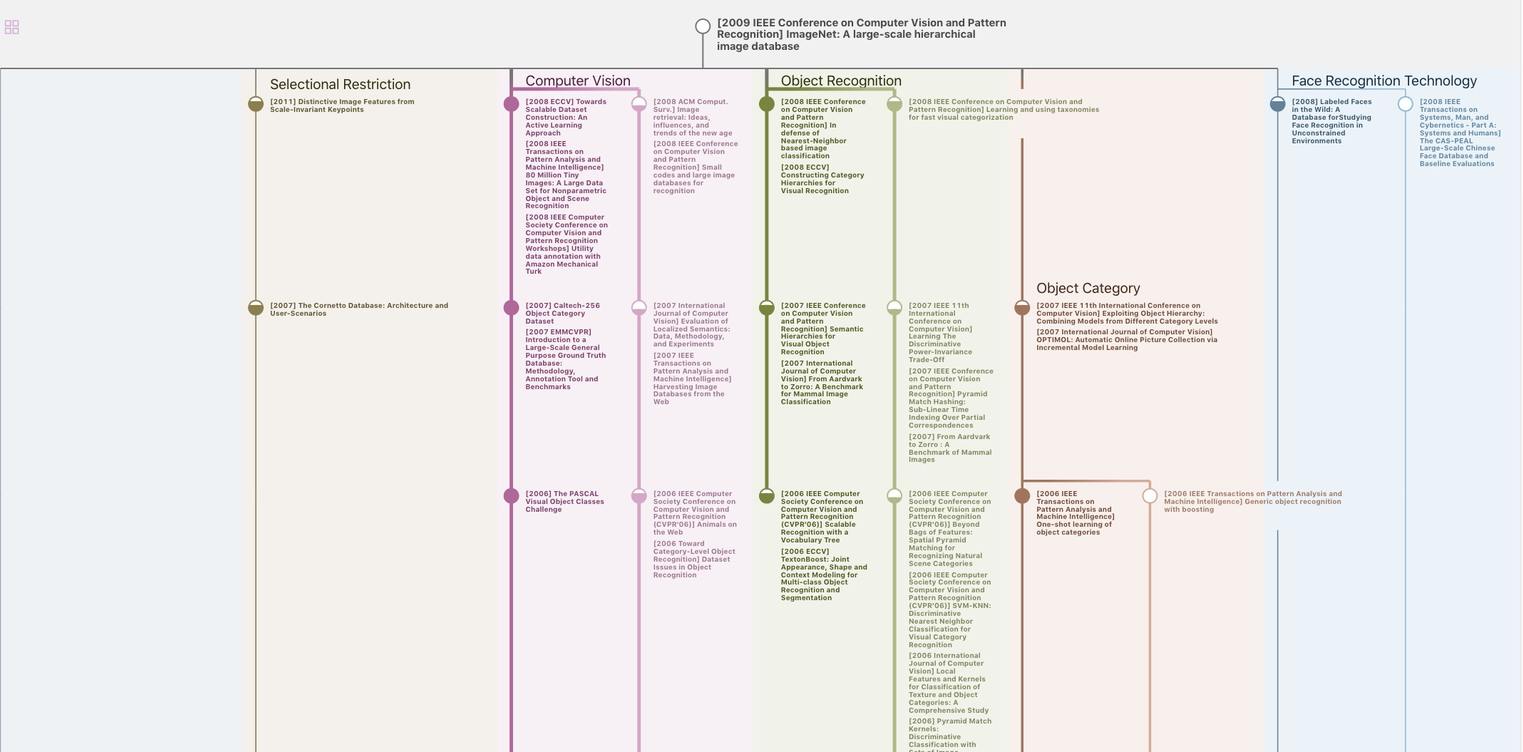
生成溯源树,研究论文发展脉络
Chat Paper
正在生成论文摘要