Efficiency Of Quantum Vs. Classical Annealing In Nonconvex Learning Problems
PROCEEDINGS OF THE NATIONAL ACADEMY OF SCIENCES OF THE UNITED STATES OF AMERICA(2018)
摘要
Quantum annealers aim at solving nonconvex optimization problems by exploiting cooperative tunneling effects to escape local minima. The underlying idea consists of designing a classical energy function whose ground states are the sought optimal solutions of the original optimization problem and add a controllable quantum transverse field to generate tunneling processes. A key challenge is to identify classes of nonconvex optimization problems for which quantum annealing remains efficient while thermal annealing fails. We show that this happens for a wide class of problems which are central to machine learning. Their energy landscapes are dominated by local minima that cause exponential slowdown of classical thermal annealers while simulated quantum annealing converges efficiently to rare dense regions of optimal solutions.
更多查看译文
关键词
nonconvex optimization, machine learning, quantum annealing, neural networks, statistical physics
AI 理解论文
溯源树
样例
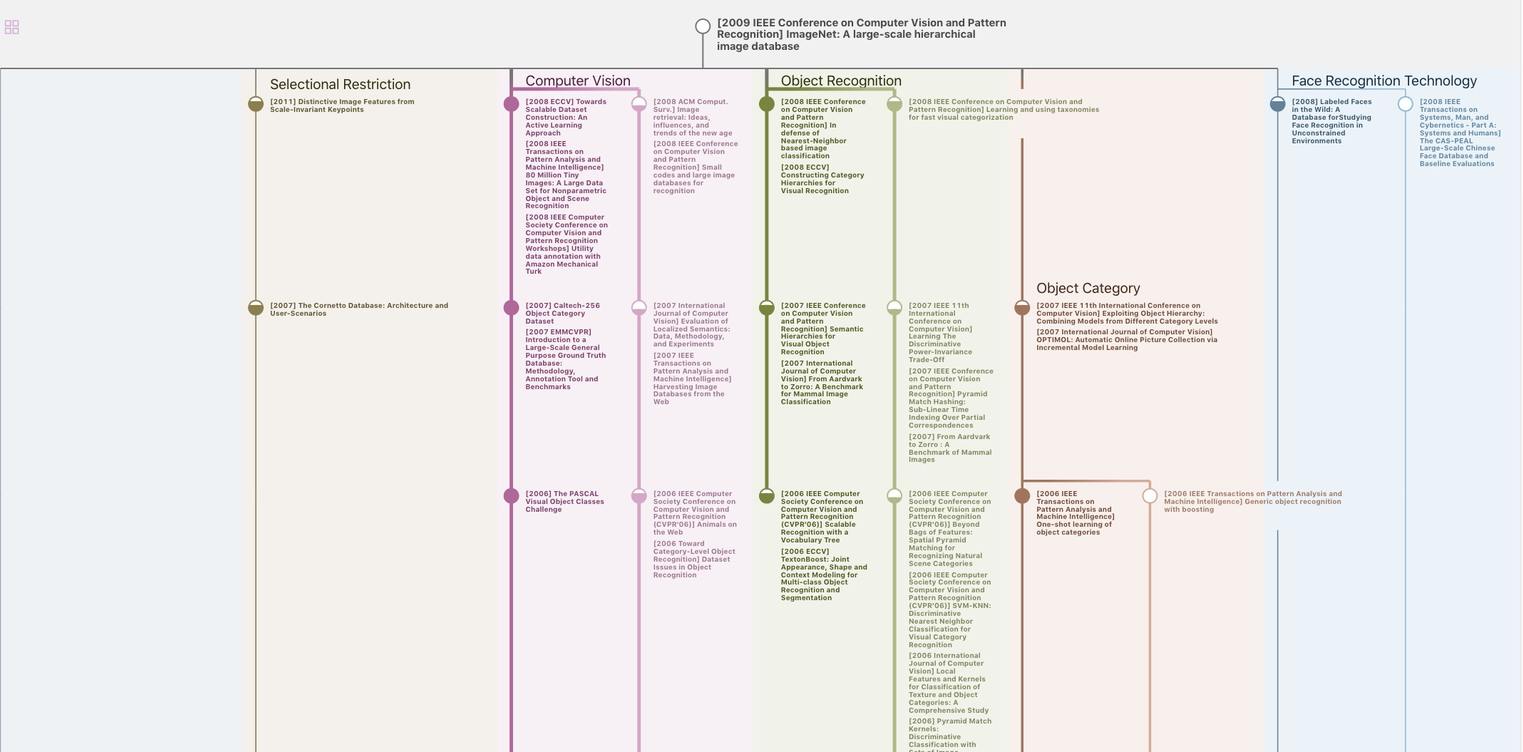
生成溯源树,研究论文发展脉络
Chat Paper
正在生成论文摘要