TALL: Temporal Activity Localization via Language Query
2017 IEEE INTERNATIONAL CONFERENCE ON COMPUTER VISION (ICCV)(2017)
摘要
This paper focuses on temporal localization of actions in untrimmed videos. Existing methods typically train classifiers for a pre-defined list of actions and apply them in a sliding window fashion. However, activities in the wild consist of a wide combination of actors, actions and objects; it is difficult to design a proper activity list that meets users' needs. We propose to localize activities by natural language queries. Temporal Activity Localization via Language (TALL) is challenging as it requires: (1) suitable design of text and video representations to allow cross-modal matching of actions and language queries; (2) ability to locate actions accurately given features from sliding windows of limited granularity. We propose a novel Cross-modal Temporal Regression Localizer (CTRL) to jointly model text query and video clips, output alignment scores and action boundary regression results for candidate clips. For evaluation, we adopt TaCoS dataset, and build a new dataset for this task on top of Charades by adding sentence temporal annotations, called Charades-STA. We also build complex sentence queries in Charades-STA for test. Experimental results show that CTRL outperforms previous methods significantly on both datasets.
更多查看译文
关键词
TALL,video representations,cross-modal matching,text query,video clips,sentence temporal annotations,untrimmed videos,natural language queries,temporal activity localization via language query,cross-modal temporal regression localizer,CTRL,action boundary regression,output alignment scores
AI 理解论文
溯源树
样例
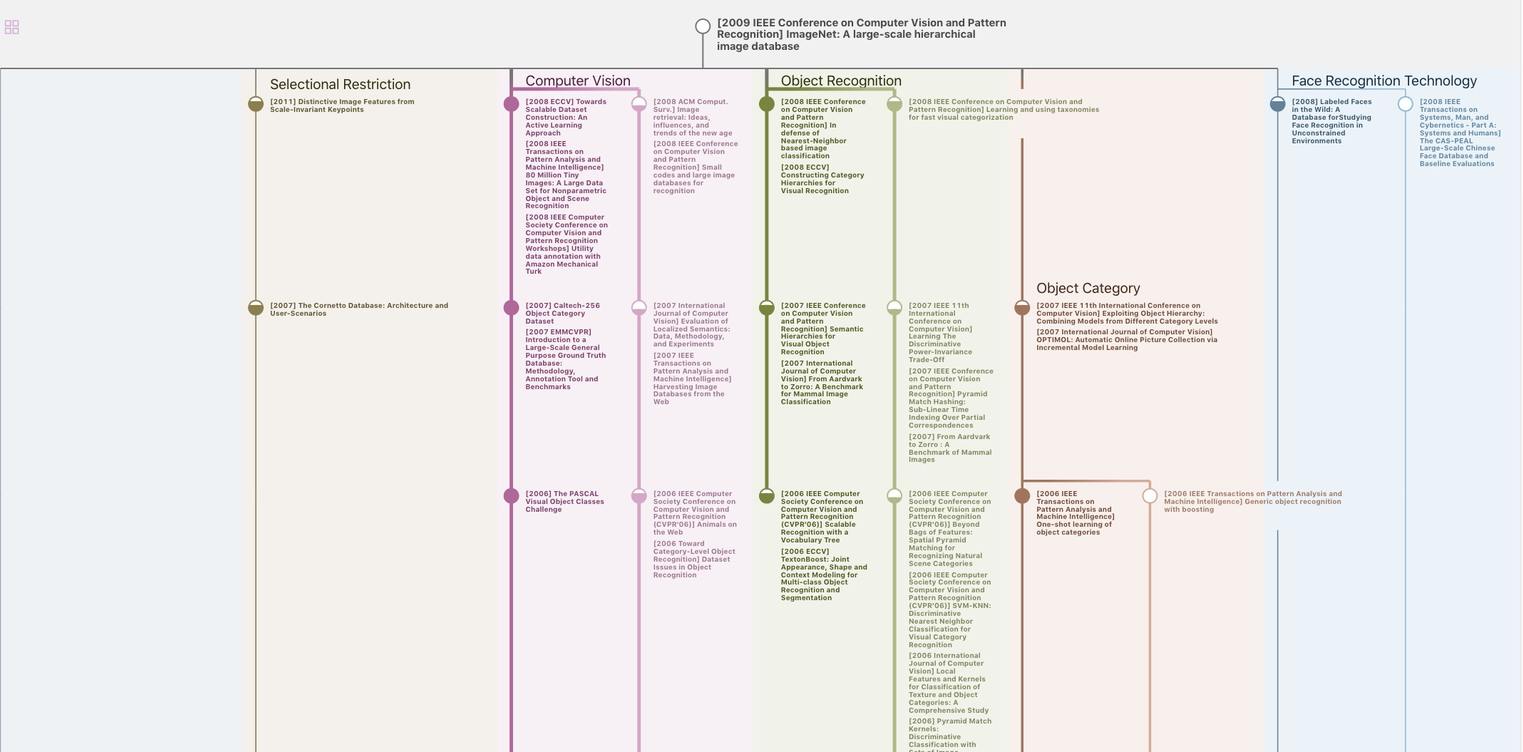
生成溯源树,研究论文发展脉络
Chat Paper
正在生成论文摘要