Mask R-CNN.
ICCV(2018)
摘要
We present a conceptually simple, flexible, and general framework for object instance segmentation. Our approach efficiently detects objects in an image while simultaneously generating a high-quality segmentation mask for each instance. The method, called Mask R-CNN, extends Faster R-CNN by adding a branch for predicting an object mask in parallel with the existing branch for bounding box recognition. Mask R-CNN is simple to train and adds only a small overhead to Faster R-CNN, running at 5 fps. Moreover, Mask R-CNN is easy to generalize to other tasks, e.g., allowing us to estimate human poses in the same framework. We show top results in all three tracks of the COCO suite of challenges, including instance segmentation, bounding-box object detection, and person keypoint detection. Without bells and whistles, Mask R-CNN outperforms all existing, single-model entries on every task, including the COCO 2016 challenge winners. We hope our simple and effective approach will serve as a solid baseline and help ease future research in instance-level recognition. Code has been made available at: https://github.com/facebookresearch/Detectron.
更多查看译文
关键词
Task analysis,Semantics,Feature extraction,Object detection,Proposals,Image segmentation,Quantization (signal)
AI 理解论文
溯源树
样例
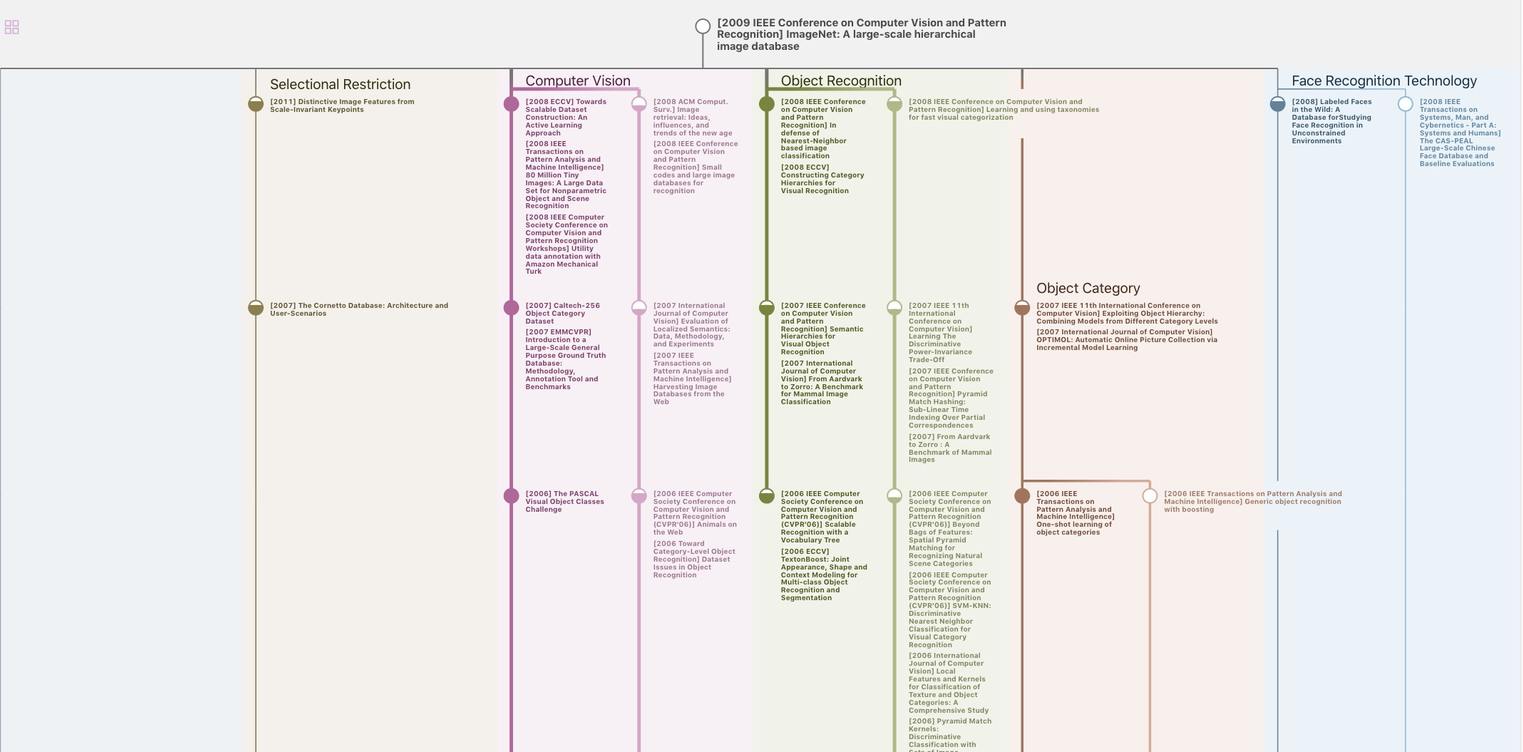
生成溯源树,研究论文发展脉络
Chat Paper
正在生成论文摘要