A Proximity-Aware Hierarchical Clustering of Faces
2017 12th IEEE International Conference on Automatic Face & Gesture Recognition (FG 2017)(2017)
摘要
In this paper, we propose an unsupervised face clustering algorithm called “Proximity-Aware Hierarchical Clustering” (PAHC) that exploits the local structure of deep representations. In the proposed method, a similarity measure between deep features is computed by evaluating linear SVM margins. SVMs are trained using nearest neighbors of sample data, and thus do not require any external training data. Clus- ters are then formed by thresholding the similarity scores. We evaluate the clustering performance using three challenging un- constrained face datasets, including Celebrity in Frontal-Profile (CFP), IARPA JANUS Benchmark A (IJB-A), and JANUS Challenge Set 3 (JANUS CS3) datasets. Experimental results demonstrate that the proposed approach can achieve significant improvements over state-of-the-art methods. Moreover, we also show that the proposed clustering algorithm can be applied to curate a set of large-scale and noisy training dataset while maintaining sufficient amount of images and their variations due to nuisance factors. The face verification performance on JANUS CS3 improves significantly by finetuning a DCNN model with the curated MS-Celeb-1M dataset which contains over three million face images.
更多查看译文
关键词
proximity-aware hierarchical clustering,PAHC,unsupervised face clustering,local structure,deep representations,similarity measure,deep features,linear SVM margins,similarity score thresholding,celebrity in frontal-profile dataset,CFP,IARPA JANUS Benchmark A dataset,IJB-A,JANUS challenge set 3 dataset,JANUS CS3,noisy training dataset,face verification,MS-Celeb-1M dataset,face images
AI 理解论文
溯源树
样例
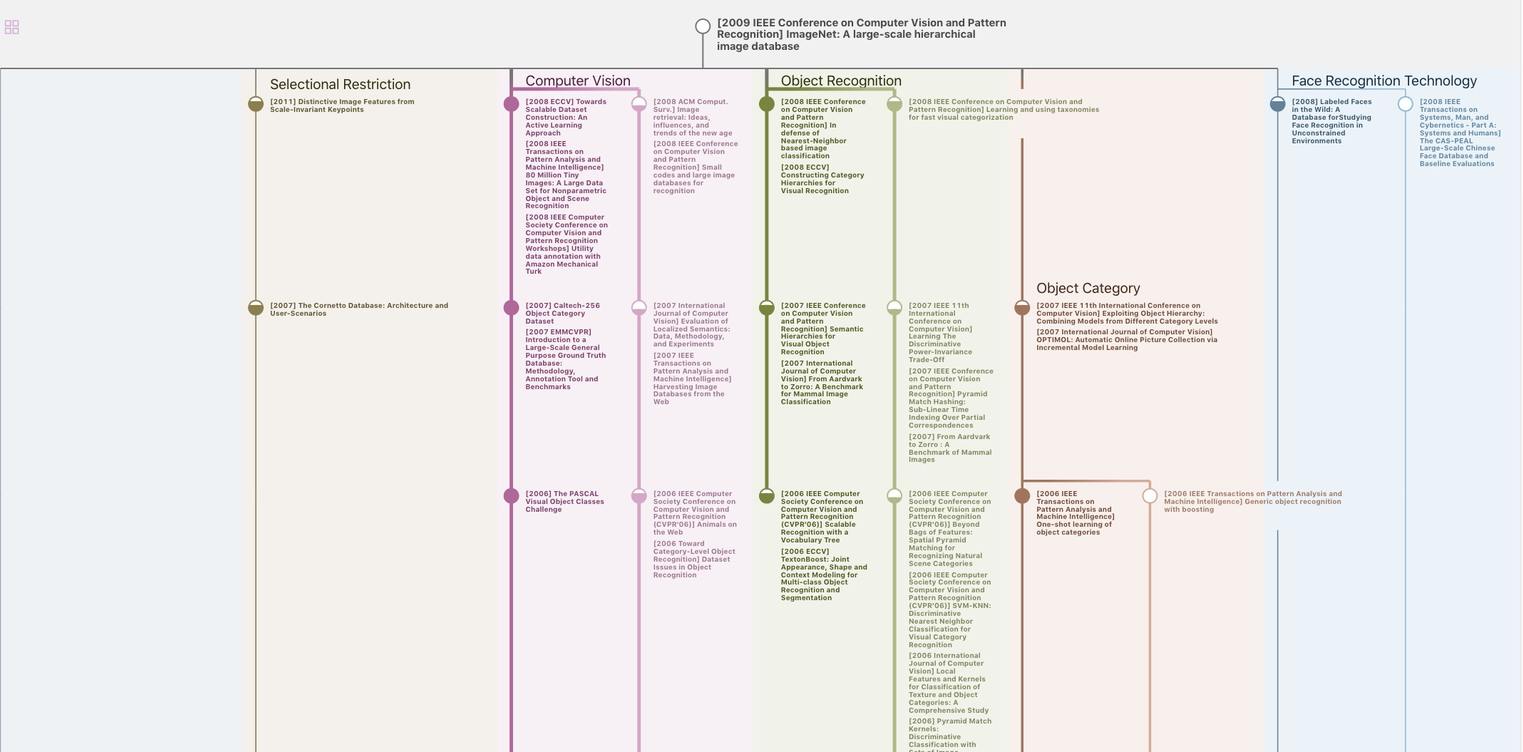
生成溯源树,研究论文发展脉络
Chat Paper
正在生成论文摘要