End-to-End Neural Ad-hoc Ranking with Kernel Pooling
IR(2017)
摘要
This paper proposes K-NRM, a kernel based neural model for document ranking. Given a query and a set of documents, K-NRM uses a translation matrix that models word-level similarities via word embeddings, a new kernel-pooling technique that uses kernels to extract multi-level soft match features, and a learning-to-rank layer that combines those features into the final ranking score. The whole model is trained end-to-end. The ranking layer learns desired feature patterns from the pairwise ranking loss. The kernels transfer the feature patterns into soft-match targets at each similarity level and enforce them on the translation matrix. The word embeddings are tuned accordingly so that they can produce the desired soft matches. Experiments on a commercial search engine's query log demonstrate the improvements of K-NRM over prior feature-based and neural-based states-of-the-art, and explain the source of K-NRM's advantage: Its kernel-guided embedding encodes a similarity metric tailored for matching query words to document words, and provides effective multi-level soft matches.
更多查看译文
关键词
Ranking,Neural IR,Kernel Pooling,Relevance Model,Embedding
AI 理解论文
溯源树
样例
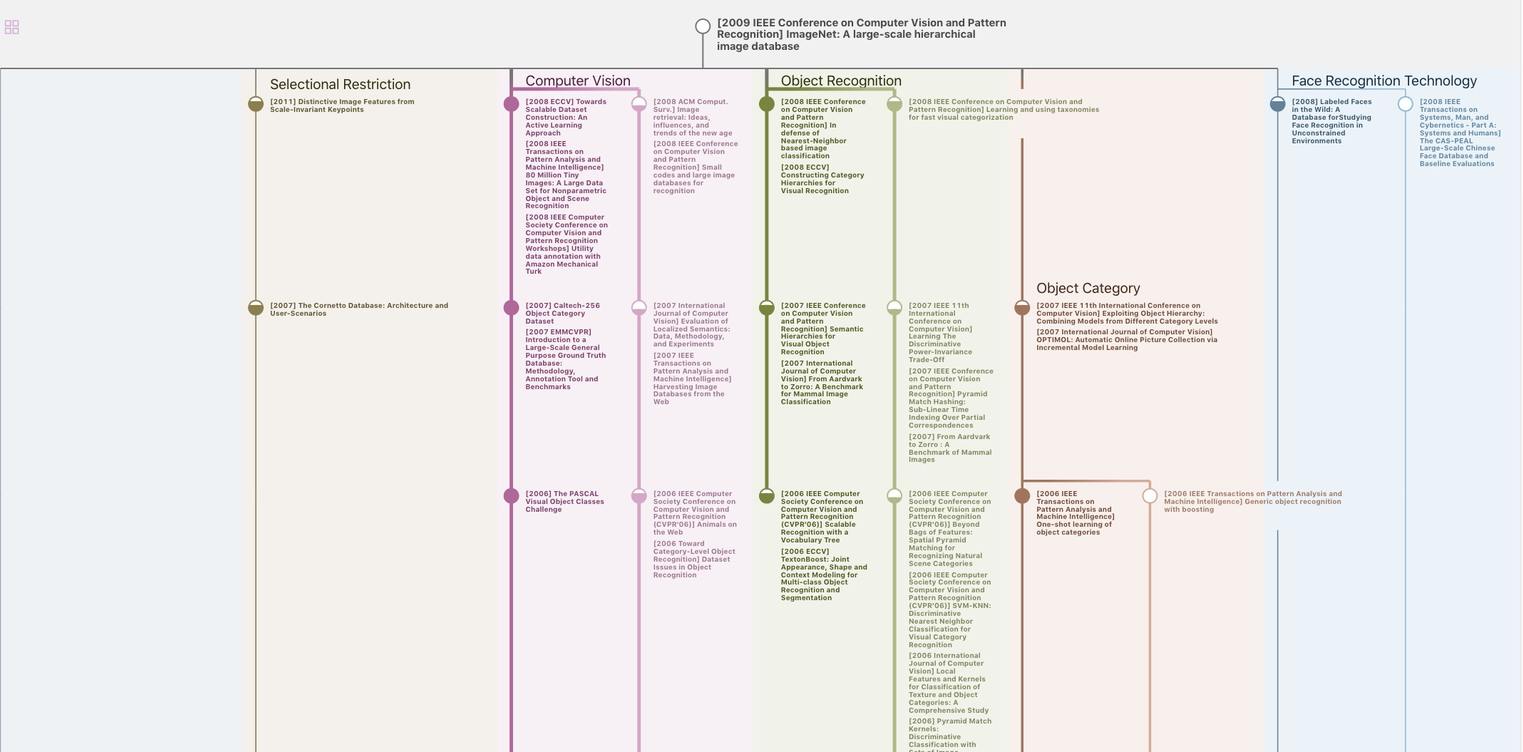
生成溯源树,研究论文发展脉络
Chat Paper
正在生成论文摘要