Weakly-Supervised Spatial Context Networks
2019 IEEE Winter Conference on Applications of Computer Vision (WACV)(2019)
摘要
We explore the power of spatial context as a self-supervisory signal for learning visual representations. In particular, we propose spatial context networks that learn to predict a representation of one image patch from another image patch, within the same image, conditioned on their real-valued relative spatial offset. Unlike auto-encoders, that aim to encode and reconstruct original image patches, our network aims to encode and reconstruct intermediate representations of the spatially offset patches. As such, the network learns a spatially conditioned contextual representation. By testing performance with various patch selection mechanisms we show that focusing on object-centric patches is important, and that using object proposal as a patch selection mechanism leads to the highest improvement in performance. Further, unlike auto-encoders, context encoders [21], or other forms of unsupervised feature learning, we illustrate that contextual supervision (with pre-trained model initialization) can improve on existing pre-trained model performance. We build our spatial context networks on top of standard VGG_19 and CNN_M architectures and, among other things, show that we can achieve improvements (with no additional explicit supervision) over the original ImageNet pre-trained VGG_19 and CNN_M models in object categorization and detection on VOC2007.
更多查看译文
关键词
unsupervised feature learning,pre-trained model performance,supervised spatial context networks,visual representations,image patch,relative spatial,auto-encoders,original image patches,intermediate representations,spatially conditioned contextual representation,patch selection mechanism,object-centric patches
AI 理解论文
溯源树
样例
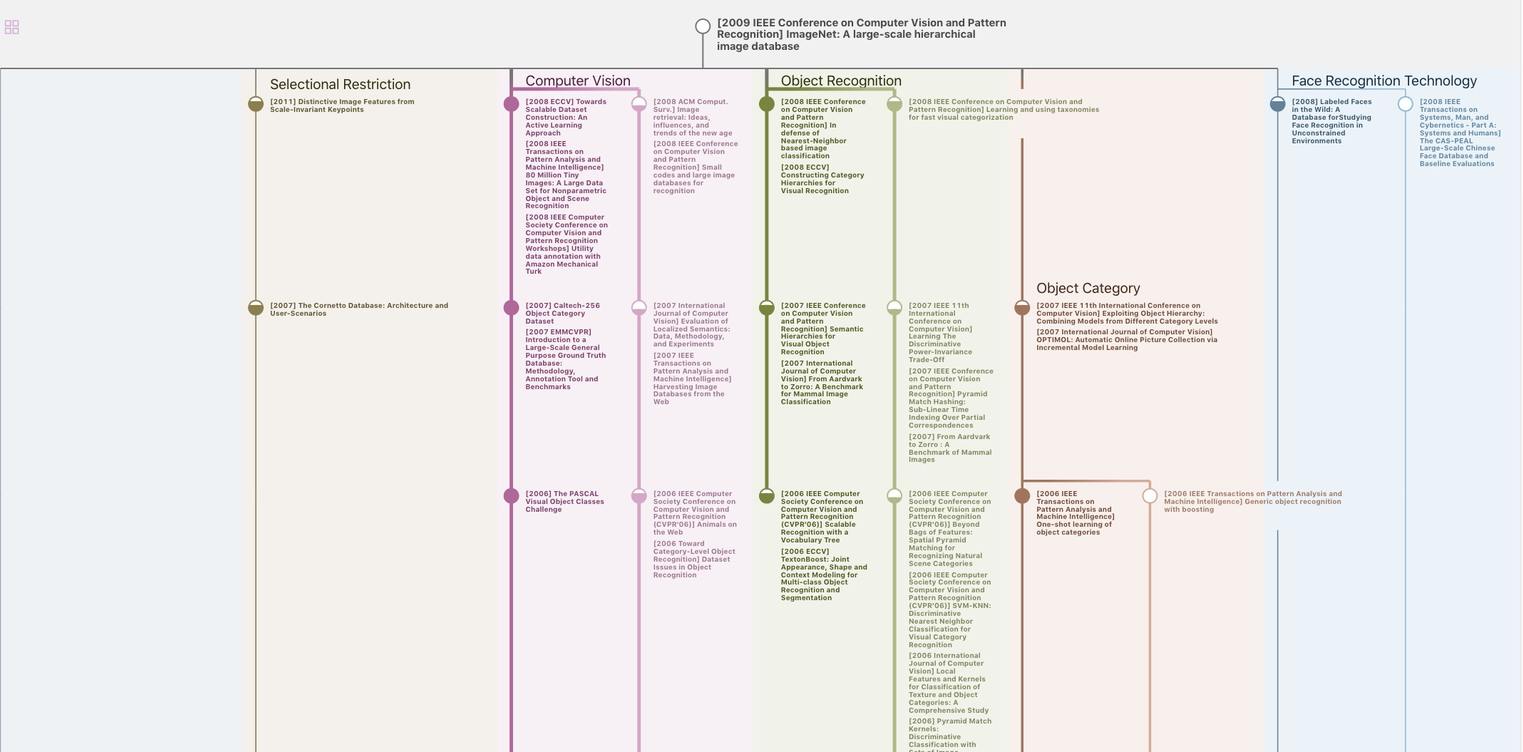
生成溯源树,研究论文发展脉络
Chat Paper
正在生成论文摘要