Neuromorphic Hardware In The Loop: Training a Deep Spiking Network on the BrainScaleS Wafer-Scale System
2017 International Joint Conference on Neural Networks (IJCNN)(2017)
摘要
Emulating spiking neural networks on analog neuromorphic hardware offers several advantages over simulating them on conventional computers, particularly in terms of speed and energy consumption. However, this usually comes at the cost of reduced control over the dynamics of the emulated networks. In this paper, we demonstrate how iterative training of a hardware-emulated network can compensate for anomalies induced by the analog substrate. We first convert a deep neural network trained in software to a spiking network on the BrainScaleS wafer-scale neuromorphic system, thereby enabling an acceleration factor of 10 000 compared to the biological time domain. This mapping is followed by the in-the-loop training, where in each training step, the network activity is first recorded in hardware and then used to compute the parameter updates in software via backpropagation. An essential finding is that the parameter updates do not have to be precise, but only need to approximately follow the correct gradient, which simplifies the computation of updates. Using this approach, after only several tens of iterations, the spiking network shows an accuracy close to the ideal software-emulated prototype. The presented techniques show that deep spiking networks emulated on analog neuromorphic devices can attain good computational performance despite the inherent variations of the analog substrate.
更多查看译文
关键词
neuromorphic hardware,deep spiking network training,BrainScaleS wafer-scale system,spiking neural networks,emulated networks,acceleration factor,biological time domain,in-the-loop training,backpropagation,analog neuromorphic devices,analog substrate
AI 理解论文
溯源树
样例
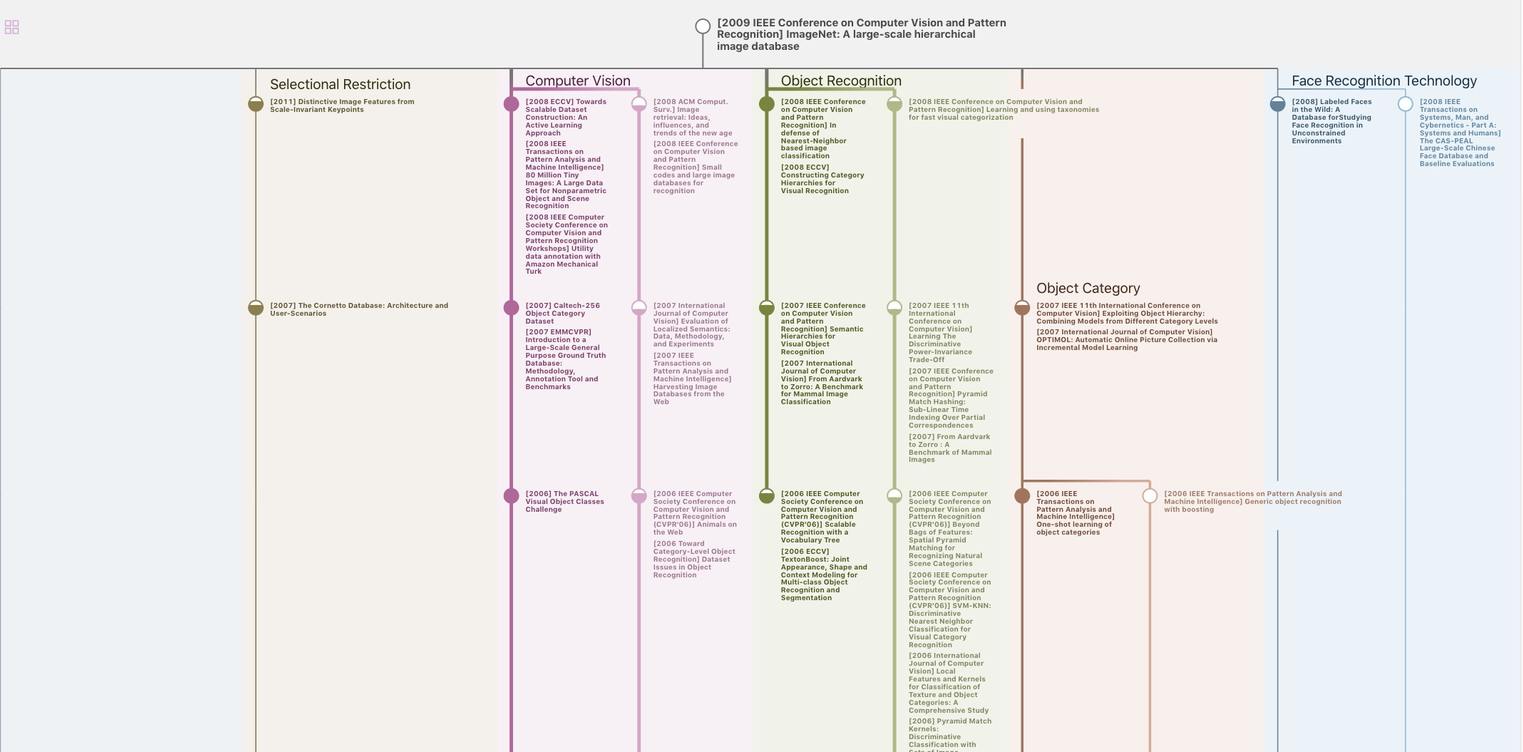
生成溯源树,研究论文发展脉络
Chat Paper
正在生成论文摘要